PREDICTION OF SORGHUM BIOMASS USING UAV TIME SERIES DATA AND RECURRENT NEURAL NETWORKS
IEEE Computer Society Conference on Computer Vision and Pattern Recognition Workshops(2019)
摘要
Phenotyping via Unmanned Aerial Vehicles (UAVs) is of increasing interest for many applications because of their capability to carry advanced sensors and achieve accurate positioning required to collect both high temporal and high spatial resolution data required over relatively limited areas. This paper focuses development of a data analytics based predictive modeling strategy that incorporates multi-sensor data acquisition systems and accommodates environmental inputs. Unsupervised feature learning based on fully connected and convolutional neural networks is investigated. Predictive models based on Recurrent Neural Networks (RNNs) are designed and implemented to accommodate high dimensional, multi modal, multi-temporal data. Remote sensing data, including Light Detection and Ranging (LiDAR) and hyperspectral inputs, as well as weather data, are incorporated in RNN models. Results from multiple experiments focused on high throughput phenotyping of sorghum for biomass predictions are provided and evaluated for agricultural test fields at the Agronomy Center for Research and Education (ACRE) at Purdue University.
更多查看译文
关键词
predictive models,recurrent neural networks,multitemporal data,remote sensing data,hyperspectral inputs,weather data,RNN models,biomass predictions,sorghum biomass,unmanned aerial vehicles,advanced sensors,high temporal resolution data,high spatial resolution data,relatively limited areas,data analytics,modeling strategy,accommodates environmental inputs,unsupervised feature,fully connected networks,convolutional neural networks,UAV time series data
AI 理解论文
溯源树
样例
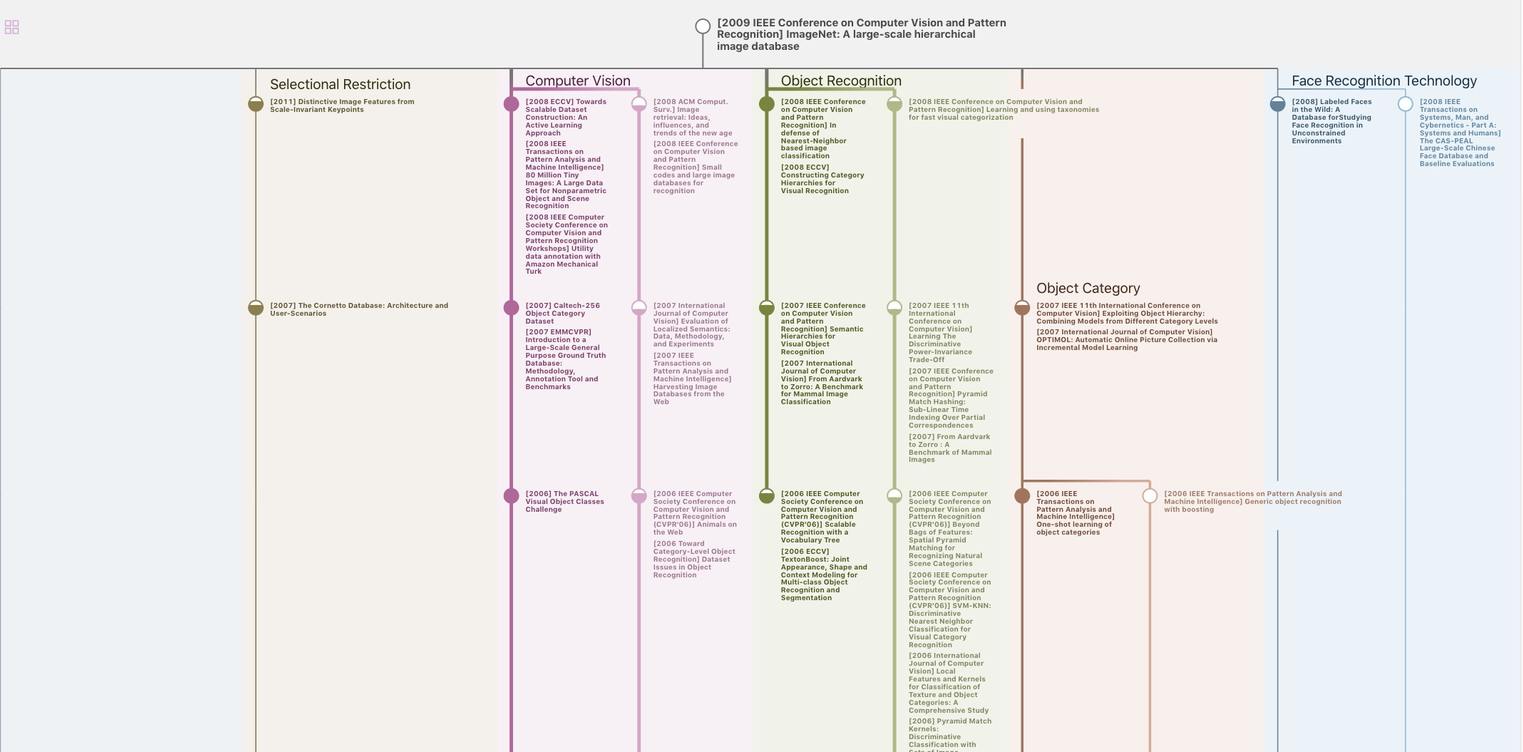
生成溯源树,研究论文发展脉络
Chat Paper
正在生成论文摘要