Data Stream Trajectory Analysis Using Sequential Possibilistic Gaussian Mixture Model.
FUZZ-IEEE(2019)
摘要
Data stream processing has gained much attention lately, in the era of big data. Streaming clustering is an effective tool to recognize normal baseline and to detect outliers in sequentially presented data. Perhaps more importantly would be the ability to predict that incoming data indicates movement towards a likely anomaly. In this paper, a Gaussian Mixture Model (GMM) is employed to represent different patterns in the data stream. The Sequential Possibilistic One-Means (SP1M) is used for initialization, and is incorporated into the GMM framework to recognize new mixture components in the data stream. The new proposed algorithm is called Sequential Possibilistic Gaussian Mixture Model (SPGMM). Furthermore, two methods of trajectory analysis, the “maximum typicality decline” and the “trend value measurement,” are used together with SPGMM to detect early signs of pattern changes before unusual pattern data arrive in the stream. The proposed SPGMM is tested on synthetic and real-world datasets, and is shown to have excellent performance on predicting early signs of pattern changes in these sequential streams.
更多查看译文
关键词
data stream trajectory analysis,sequential possibilistic gaussian mixture model,data stream processing,big data,streaming clustering,sequentially presented data,mixture components,pattern changes,unusual pattern data,sequential streams,sequential possibilistic one-means
AI 理解论文
溯源树
样例
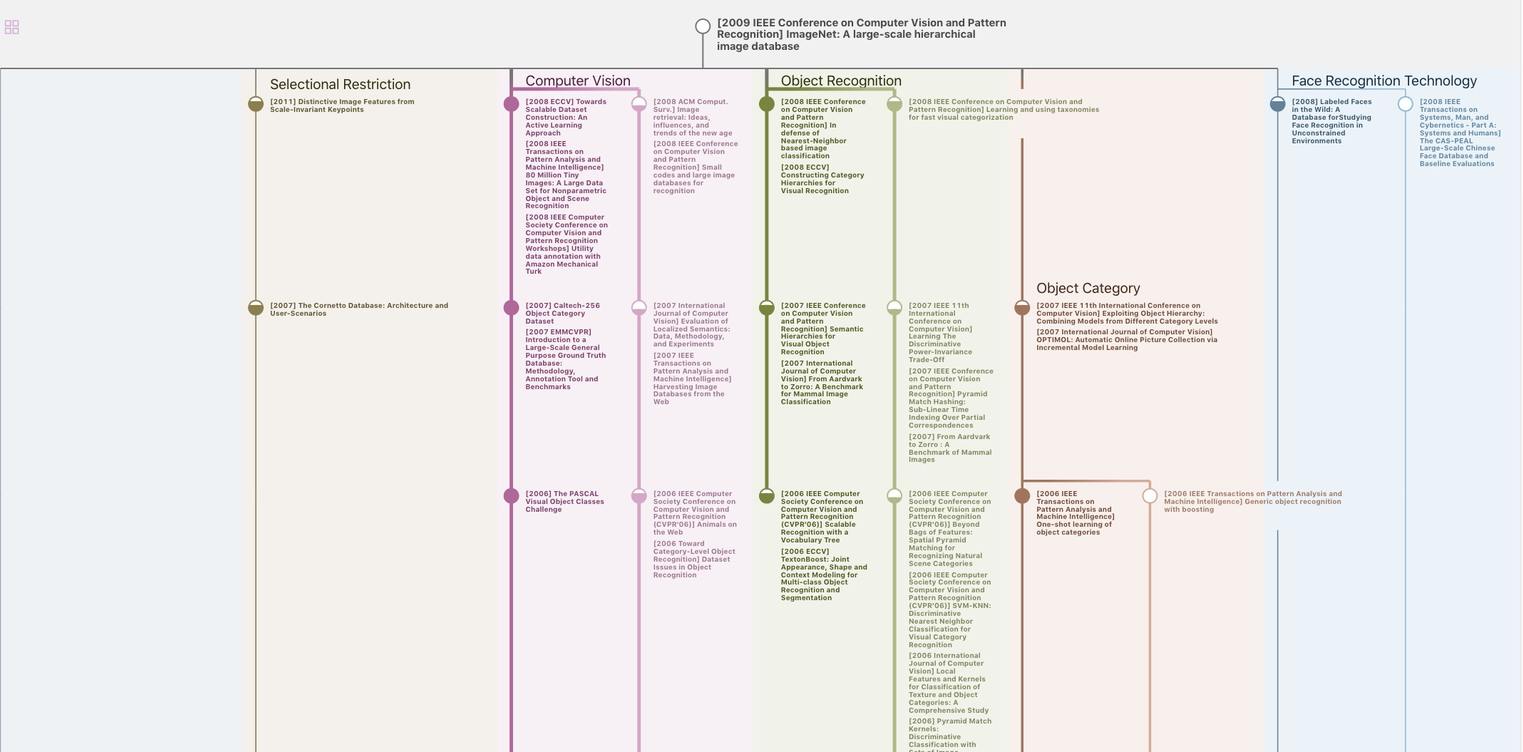
生成溯源树,研究论文发展脉络
Chat Paper
正在生成论文摘要