Unsupervised Question Answering for Fact-Checking
CoRR(2019)
摘要
Recent Deep Learning (DL) models have succeeded in achieving human-level accuracy on various natural language tasks such as question-answering, natural language inference (NLI), and textual entailment. These tasks not only require the contextual knowledge but also the reasoning abilities to be solved efficiently. In this paper, we propose an unsupervised question-answering based approach for a similar task, fact-checking. We transform the FEVER dataset into a Cloze-task by masking named entities provided in the claims. To predict the answer token, we utilize pre-trained Bidirectional Encoder Representations from Transformers (BERT). The classifier computes label based on the correctly answered questions and a threshold. Currently, the classifier is able to classify the claims as "SUPPORTS" and "MANUAL_REVIEW". This approach achieves a label accuracy of 80.2% on the development set and 80.25% on the test set of the transformed dataset.
更多查看译文
关键词
fact-checking
AI 理解论文
溯源树
样例
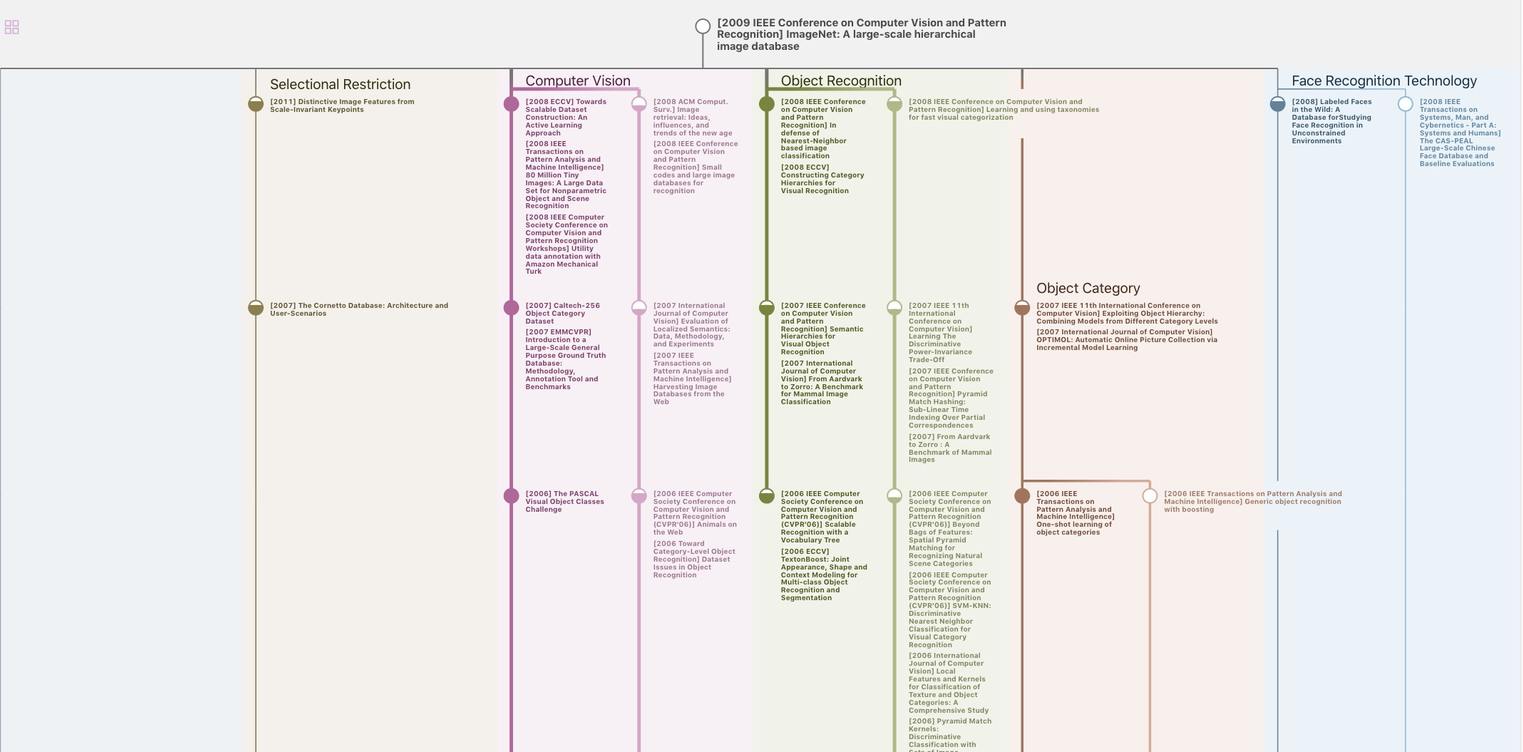
生成溯源树,研究论文发展脉络
Chat Paper
正在生成论文摘要