Interpretable Partitioned Embedding for Intelligent Multi-item Fashion Outfit Composition.
ACM Transactions on Multimedia Computing, Communications, and Applications(2019)
摘要
Intelligent fashion outfit composition has become more popular in recent years. Some deep-learning-based approaches reveal competitive composition. However, the uninterpretable characteristic makes such a deep-learning-based approach fail to meet the businesses’, designers’, and consumers’ urges to comprehend the importance of different attributes in an outfit composition. To realize interpretable and intelligent multi-item fashion outfit compositions, we propose a partitioned embedding network to learn interpretable embeddings from clothing items. The network contains two vital components: attribute partition module and partition adversarial module. In the attribute partition module, multiple attribute labels are adopted to ensure that different parts of the overall embedding correspond to different attributes. In the partition adversarial module, adversarial operations are adopted to achieve the independence of different parts. With the interpretable and partitioned embedding, we then construct an outfit-composition graph and an attribute matching map. Extensive experiments demonstrate that (1) the partitioned embedding have unmingled parts that correspond to different attributes and (2) outfits recommended by our model are more desirable in comparison with the existing methods.
更多查看译文
关键词
Outfit composition,adversarial,embedding,interpretable
AI 理解论文
溯源树
样例
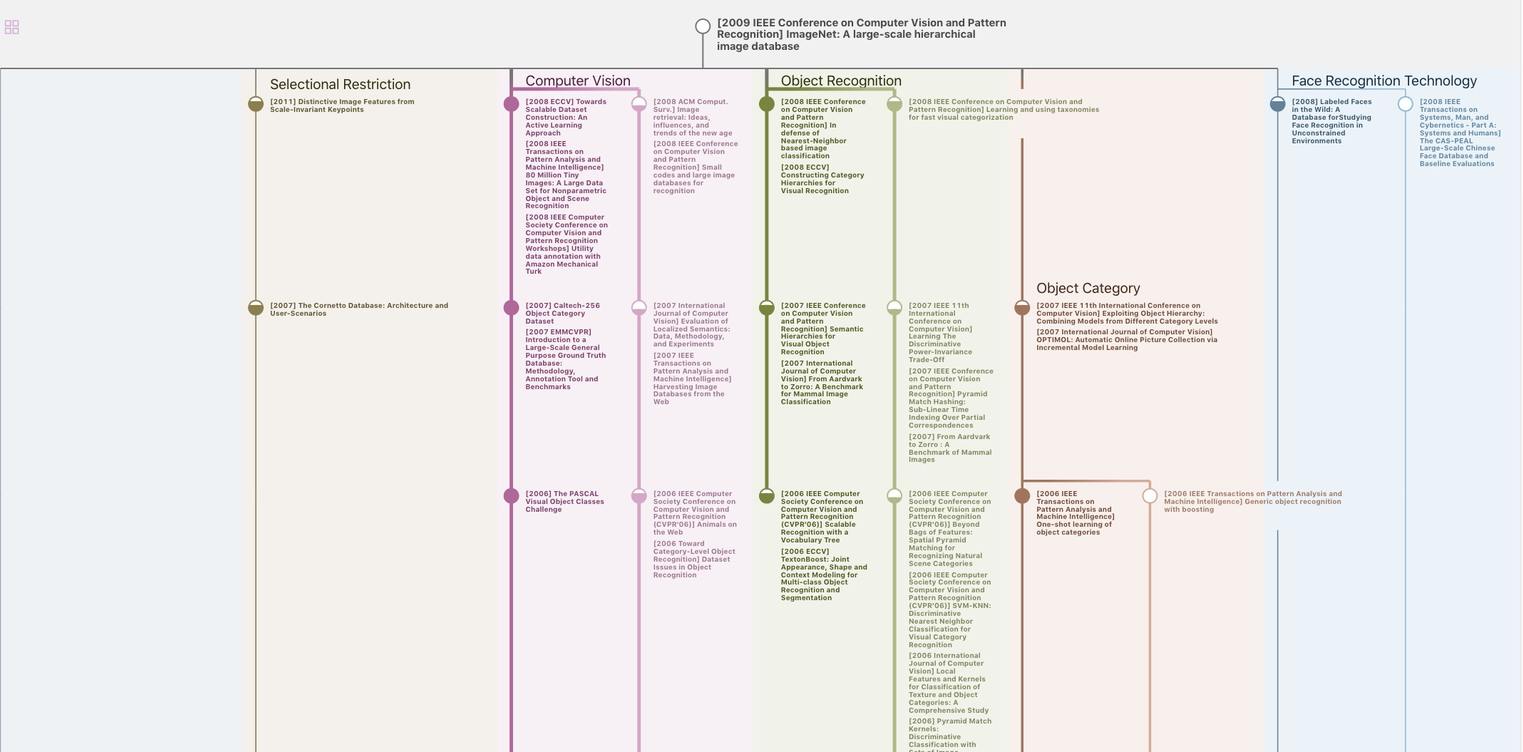
生成溯源树,研究论文发展脉络
Chat Paper
正在生成论文摘要