A Pseudo-likelihood Approach for Geo-localization of Events from Crowd-sourced Sensor-Metadata.
ACM Transactions on Multimedia Computing, Communications, and Applications(2019)
摘要
Events such as live concerts, protest marches, and exhibitions are often video recorded by many people at the same time, typically using smartphone devices. In this work, we address the problem of geo-localizing such events from crowd-generated data. Traditional approaches for solving such a problem using multiple video sequences of the event would require highly complex computer vision (CV) methods, which are computation intensive and are not robust under the environment where visual data are collected through crowd-sourced medium. In the present work, we approach the problem in a probabilistic framework using only the sensor metadata obtained from smartphones. We model the event location and camera locations and orientations (camera parameters) as the hidden states in a Hidden Markov Model. The sensor metadata from GPS and the digital compass from user smartphones are used as the observations associated with the hidden states of the model. We have used a suitable potential function to capture the complex interaction between the hidden states (i.e., event location and camera parameters). The non-Gaussian densities involved in the model, such as the potential function involving hidden states, make the maximum-likelihood estimation intractable. We propose a pseudo-likelihood-based approach to maximize the approximate-likelihood, which provides a tractable solution to the problem. The experimental results on the simulated as well as real data show correct event geo-localization using the proposed method. When compared with several baselines the proposed method shows a superior performance. The overall computation time required is much smaller, since only the sensor metadata are used instead of visual data.
更多查看译文
关键词
GPS,HMM,Pseudo-likelihood,crowd sourcing,digital compass,event localization,smartphones,video analysis,von-Mises distribution
AI 理解论文
溯源树
样例
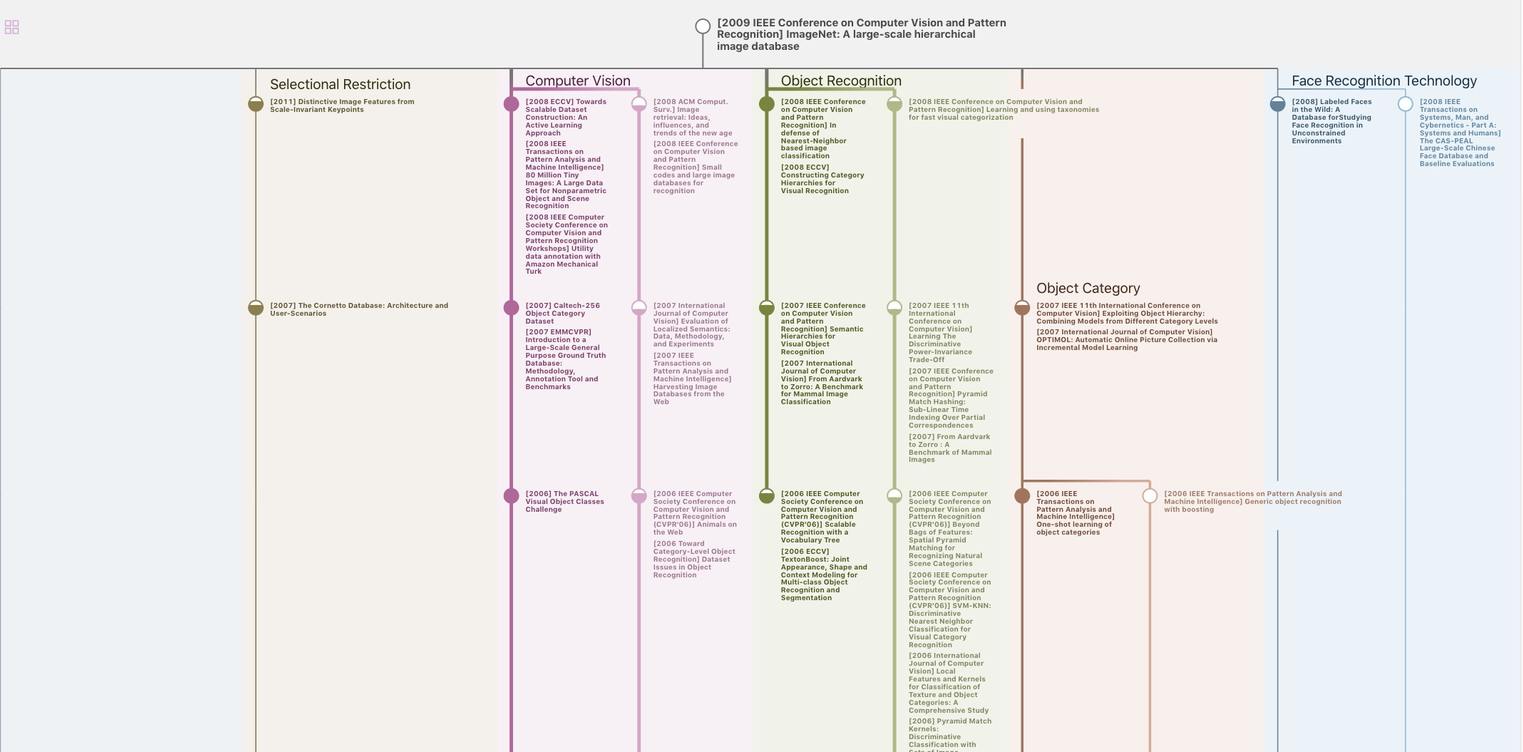
生成溯源树,研究论文发展脉络
Chat Paper
正在生成论文摘要