Machine Learning-Based Risk Of Hospital Readmissions: Predicting Acute Readmissions Within 30 Days Of Discharge
2019 41ST ANNUAL INTERNATIONAL CONFERENCE OF THE IEEE ENGINEERING IN MEDICINE AND BIOLOGY SOCIETY (EMBC)(2019)
摘要
The objective of this study was to design and develop a 30-day risk of hospital readmission predictive model using machine learning techniques. The proposed risk of readmission predictive model was then validated with the two most commonly used risk of readmission models - LACE index and patient at-risk of hospital readmission (PARR). The study cohort consisted of 180,118 admissions with 22565 (12.5%) of actual readmissions within 30-day of hospital discharge, from 01 Jan 2015 to 31 Dec 2016 from two Auckland-region hospitals. We developed a machine learning model to predict 30-day readmissions using the model types: XGBoost, Random Forests and Adaboost with decision stumps as a base learner with different feature combinations and preprocessing procedures. The proposed model achieved the F1-score (0.386 +/- 0.006), sensitivity (0.598 +/- 0.013), positive predictive value (PPV) (0.285 +/- 0.004) and negative predictive value (NPV) (0.932 +/- 0.002). When compared with LACE and PARR (NZ) models, the proposed model achieved better F1-score by 12.5% compared to LACE and 22.9% compared to PARR (NZ). The mean sensitivity of the proposed model was 6.0% higher than LACE and 42.4% higher than PARR (NZ). The mean PPV was 15.9% and 13.5% higher than LACE and PARR (NZ) respectively.
更多查看译文
关键词
Risk of readmission, machine learning model, LACE, PARR and Patient at risk of hospital readmission
AI 理解论文
溯源树
样例
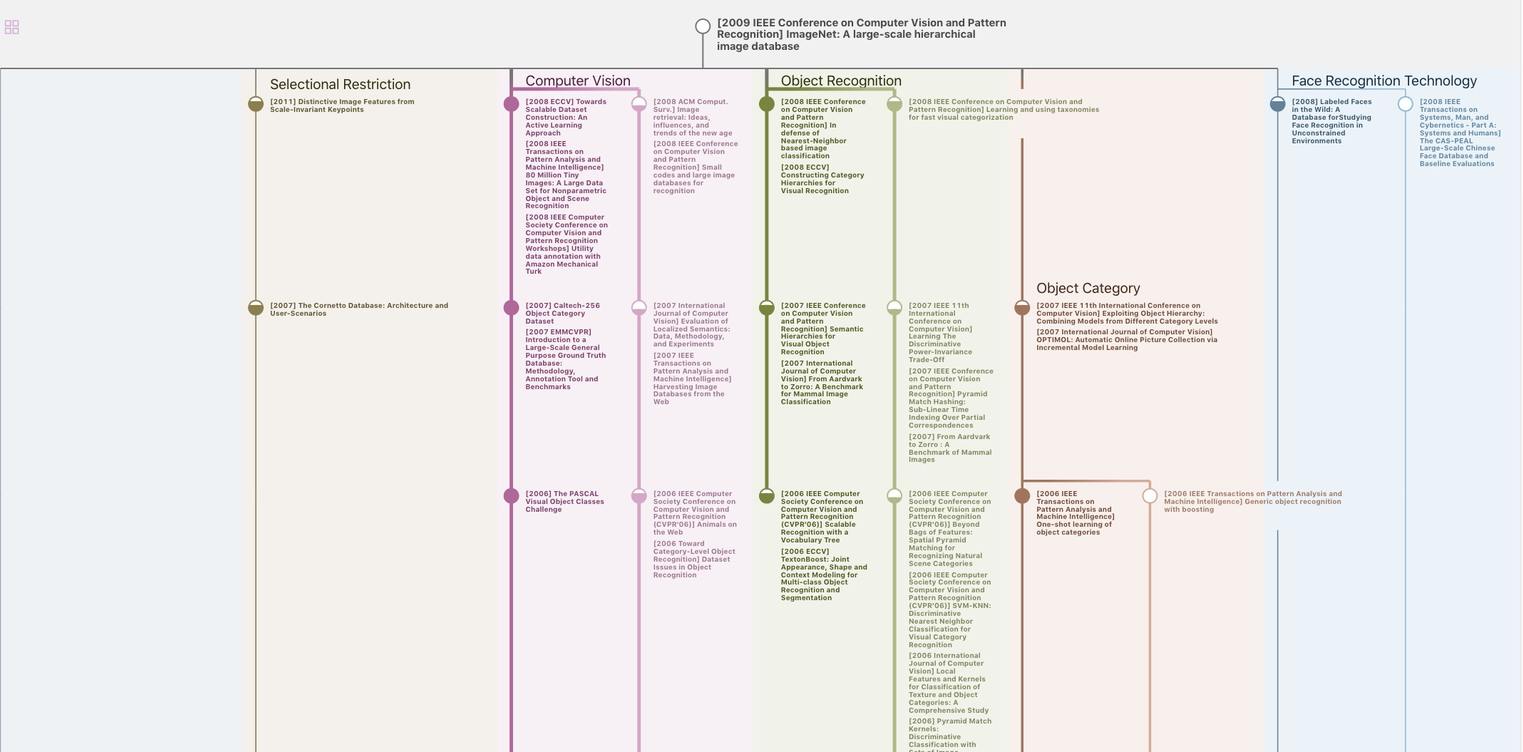
生成溯源树,研究论文发展脉络
Chat Paper
正在生成论文摘要