Neural Activity from Attention Networks Predicts Movement Errors
2019 41ST ANNUAL INTERNATIONAL CONFERENCE OF THE IEEE ENGINEERING IN MEDICINE AND BIOLOGY SOCIETY (EMBC)(2019)
摘要
Traditionally, movement-related behavior is estimated using activity from motor regions in the brain. This predictive capability of interpreting neural signals into tangible outputs has led to the emergence of Brain-Computer Interface (BCI) systems. However, nonmotor regions can play a significant role in shaping how movements are executed. Our goal was to explore the contribution of nonmotor brain regions to movement using a unique experimental paradigm in which local field potential recordings of several cortical and subcortical regions were obtained from eight epilepsy patients implanted with depth electrodes as they performed goal-directed reaching movements. The instruction of the task was to move a cursor with a robotic arm to the indicated target with a specific speed, where correct trials were ones in which the subject achieved the instructed speed. We constructed subject-specific models that predict the speed error of each trial from neural activity in nonmotor regions. Neural features were found by averaging spectral power of activity in multiple frequency bands produced during the planning or execution of movement. Features with high predictive power were selected using a forward selection greedy search. Using our modeling framework, we were able to identify networks of regions related to attention that significantly contributed to predicting trial errors. Our results suggest that nonmotor brain regions contain relevant information about upcoming movements and should be further studied.
更多查看译文
关键词
Attention,Brain,Brain Mapping,Brain-Computer Interfaces,Humans,Movement
AI 理解论文
溯源树
样例
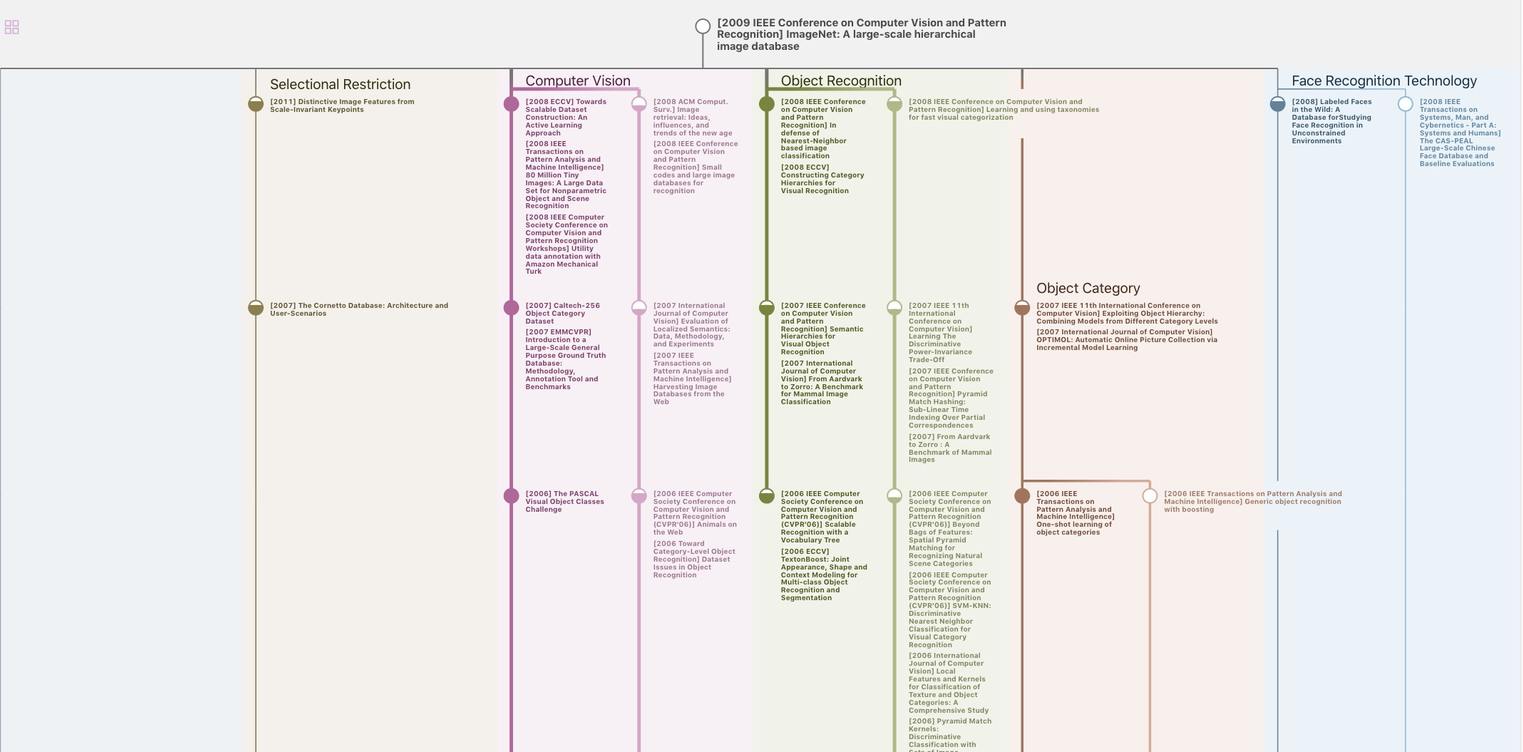
生成溯源树,研究论文发展脉络
Chat Paper
正在生成论文摘要