Privacy-Preserving Artificial Intelligence: Application To Precision Medicine
2019 41ST ANNUAL INTERNATIONAL CONFERENCE OF THE IEEE ENGINEERING IN MEDICINE AND BIOLOGY SOCIETY (EMBC)(2019)
摘要
Motivated by state-of-the-art performances across a wide variety of areas, over the last few years Machine Learning has drawn a significant amount of attention from the healthcare domain. Despite their potential in enabling personalized medicine applications, the adoption of Deep Learning based solutions in clinical workflows has been hindered in many cases by the strict regulations concerning the privacy of patient health data. We propose a solution that relies on Fully Homomorphic Encryption, particularly on the MORE scheme, as a mechanism for enabling computations on sensitive health data, without revealing the underlying data. The chosen variant of the encryption scheme allows for the computations in the Neural Network model to be directly performed on floating point numbers, while incurring a reasonably small computational overhead. For feasibility evaluation, we demonstrate on the MNIST digit recognition task that Deep Learning can be performed on encrypted data without compromising the accuracy. We then address a more complex task by training a model on encrypted data to estimate the outputs of a whole-body circulation (WBC) model. These results underline the potential of the proposed approach to outperform current solutions by delivering comparable results to the unencrypted Deep Learning based solutions, in a reasonable amount of time. Lastly, the security aspects of the encryption scheme are analyzed, and we show that, even though the chosen encryption scheme favors performance and utility at the cost of weaker security, it can still be used in certain practical applications.
更多查看译文
关键词
Artificial Intelligence,Computer Security,Humans,Precision Medicine,Privacy
AI 理解论文
溯源树
样例
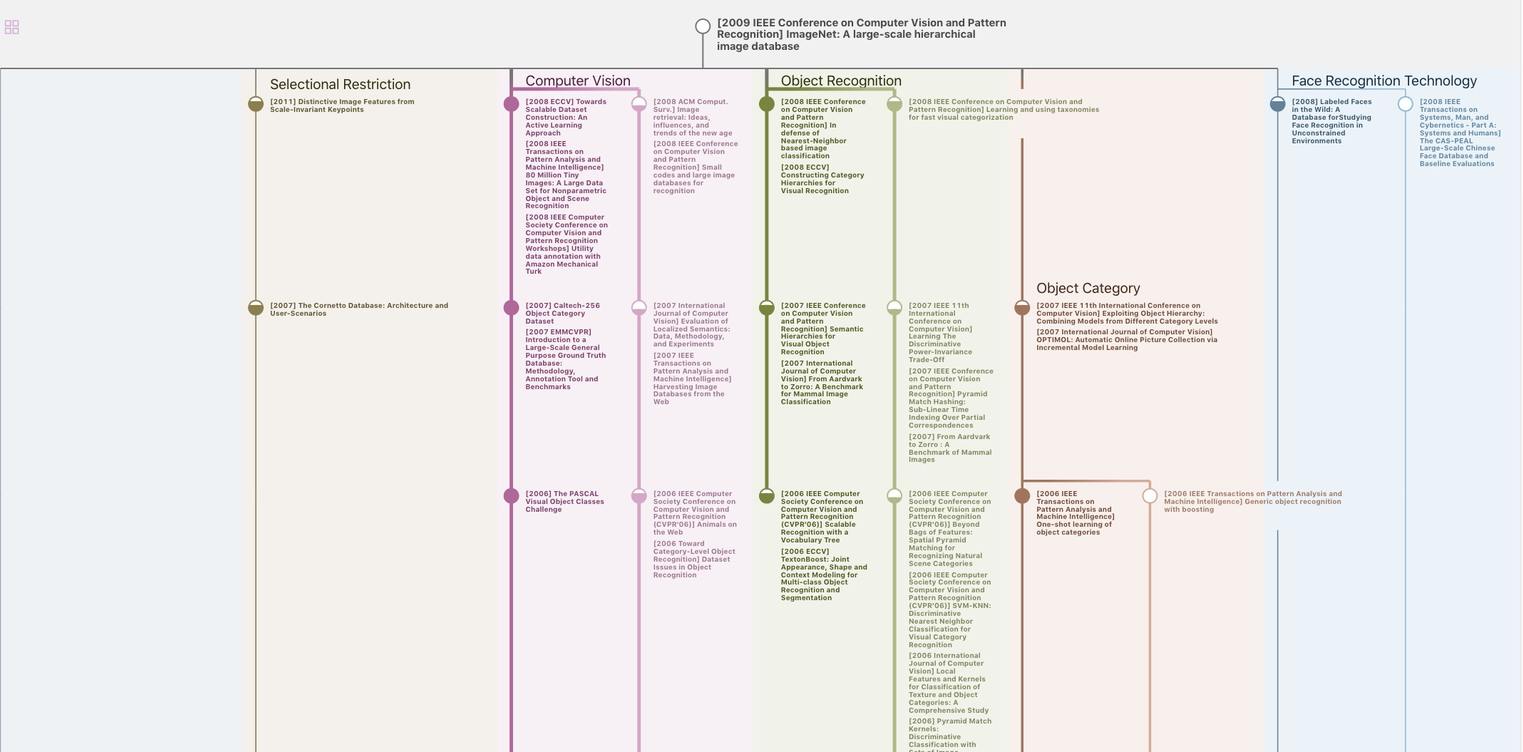
生成溯源树,研究论文发展脉络
Chat Paper
正在生成论文摘要