Matched Forest: Supervised Learning for High-Dimensional Matched Case-Control Studies.
Bioinformatics(2019)
摘要
MOTIVATION Matched case-control analysis is widely used in biomedical studies to identify exposure variables associated with health conditions. The matching is used to improve the efficiency. Existing variable selection methods for matched case-control studies are challenged in high-dimensional settings where interactions among variables are also important. We describe a quite different method for high-dimensional matched case-control data, based on the potential outcome model, which is not only flexible regarding the number of matching and exposure variables, but also able to detect interaction effects. RESULTS We present Matched Forest (MF), an algorithm for variable selection in matched case-control data. The method preserves the case and control values in each instance, but transforms the matched case-control data with added counterfactuals. A modified variable importance score from a supervised learner is used to detect important variables. The method is conceptually simple and can be applied with widely available software tools. Simulation studies show the effectiveness of MF in identifying important variables. MF is also applied to data from the biomedical domain and its performance is compared with alternative approaches. AVAILABILITY R code for implementing MF is available at https://github.com/NooshinSh/Matched_Forest. SUPPLEMENTARY INFORMATION The four biomedical data sets are available at Bioinformatics online.
更多查看译文
AI 理解论文
溯源树
样例
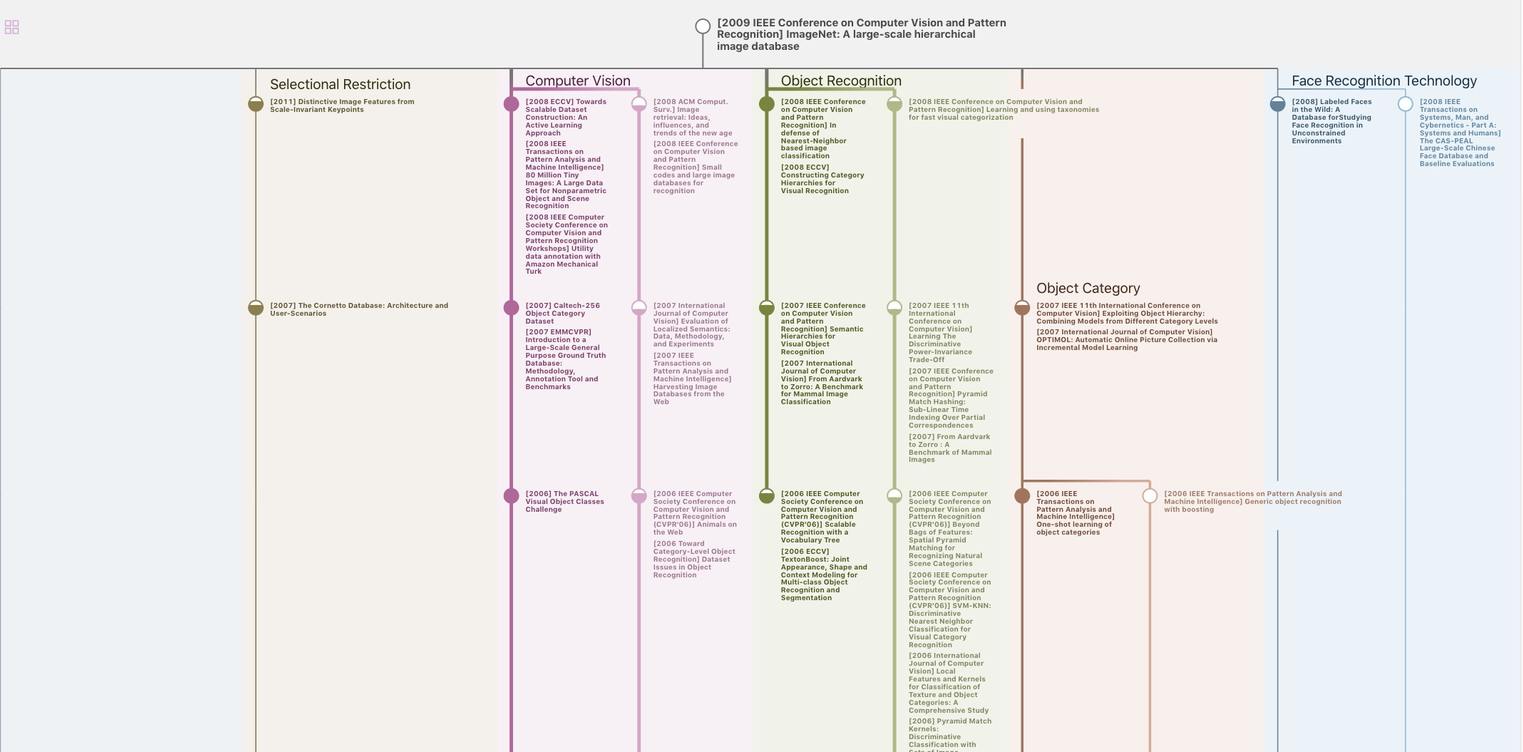
生成溯源树,研究论文发展脉络
Chat Paper
正在生成论文摘要