Don'T Mention It? Analyzing User-Generated Content Signals For Early Adverse Event Warnings
INFORMATION SYSTEMS RESEARCH(2019)
摘要
With greater impetus on broad postmarket surveillance, the Voice of the Customer (VoC) has emerged as an important source of information for understanding consumer experiences and identifying potential issues. In organizations, risk management groups are increasingly interested in working with their information technology teams to develop robust VoC listening platforms. Two key challenges have impeded success. First, prior work has leveraged diverse sets of channels, adverse event types, and modeling methods, resulting in diverging conclusions regarding the viability and efficacy of various user-generated channels and accompanying modeling methods. Second, many existing detection methods rely on "mention models" that have low detection rates, have high false positives, and lack timeliness. Following the information systems design science approach, in this research note we propose a framework for examining key design elements for VoC listening platforms. As part of our framework, we also develop a novel heuristic-based method for detecting adverse events. We evaluate our framework and method on two large test beds each encompassing millions of tweets, forums postings, and search query logs pertaining to hundreds of adverse events related to the pharmaceutical and automotive industries. The results shed light on the interplay between user-generated channels and event types, as well as the potential for more robust event modeling methods that go beyond basic mention models. Our analysis framework reveals that user-generated content channels can facilitate timelier detection of adverse events: on average, two to three years or earlier than commonly used databases. The inclusion of negative sentiment polarity in the models can further reduce false-positive rates. Additionally, we find social media channels provide higher detection rates but lower precision than do search-based signals. The search and web forum channels are timelier than Twitter. The proposed heuristic-based method attains markedly better results than do existing methods-with earlier detection rates of 50%-80% and far fewer false positives across an array of VoC channels and event types. The heuristic method is also well suited for signal fusion across channels. Our note makes several contributions to research. The results also have important implications for various practitioner groups, including regulatory agencies and risk management teams at product manufacturing firms.
更多查看译文
关键词
signal detection, social media, data mining, smart health, predictive analytics, healthcare analytics, healthcare information technologies
AI 理解论文
溯源树
样例
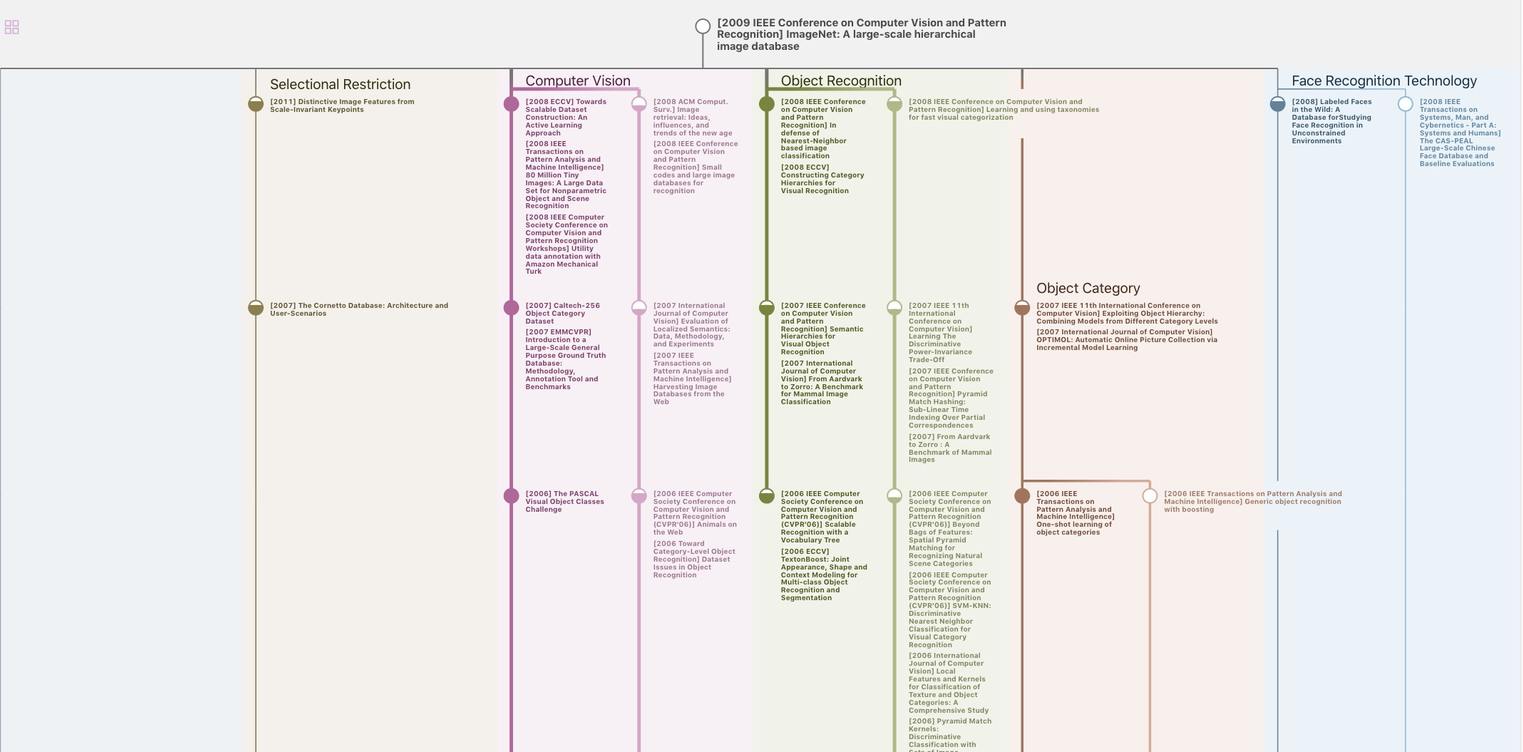
生成溯源树,研究论文发展脉络
Chat Paper
正在生成论文摘要