Stacked dense networks for single-image snow removal.
Neurocomputing(2019)
摘要
Single image snow removal is important since snowy images usually degrade the performance of computer vision systems. In this paper, we deduce a physics-based snow model and propose a novel snow removal method based on the snow model and deep neural networks. Our model decomposes a snowy image into a nonlinear combination of a snow-free image and dynamic snowflakes. Inspired by our model and DenseNet connectivity pattern, we design a novel Multi-scale Stacked Densely Connected Convolutional Network (MS-SDN) to simultaneously detect and remove snowflakes in an image. The MS-SDN is composed of a multi-scale convolutional sub-net for extracting feature maps and two stacked modified DenseNets for snowflakes detection and removal. The snowflake detection sub-net guides snow removal through forward transmission, and the snowflake removal sub-net adjusts snow detection through back transmission. In this way, snowflake detection and removal mutually improve the final results. For training and testing our method, we constructed a large-scale benchmark synthesis dataset which contains 3000 triplets of snowy images, snowflakes, and snow-free images. Specifically, the snow-free images are captured from snow scenes, and the snowy images are synthesized by using our deduced snow model. Our extensive quantitative and qualitative experimental results show that our MS-SDN performs better than several state-of-the-art methods, and the stacked structure is better than multi-branch structures in terms of snow removal.
更多查看译文
关键词
Snow removal,Single image,Stacked dense networks,Image restoration
AI 理解论文
溯源树
样例
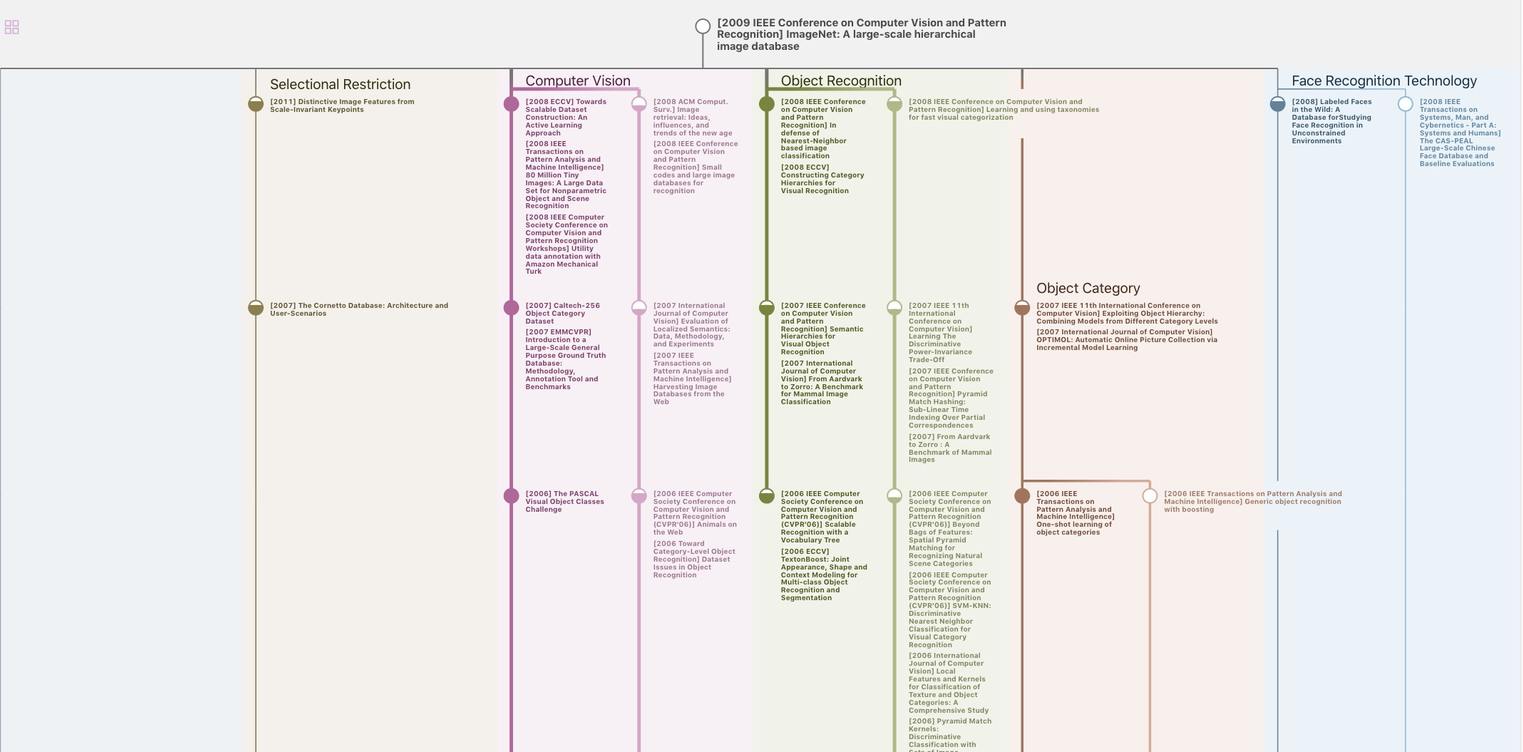
生成溯源树,研究论文发展脉络
Chat Paper
正在生成论文摘要