A probabilistic framework to incorporate mixed-data type features: Matrix factorization with multimodal side information.
Neurocomputing(2019)
摘要
Recommender systems that exclusively rely on past interactions between the users and the items underperform in settings with very few observations. Their performance at such extreme sparsity can be improved by exploiting the side information of the users and the items including the demographics and the item descriptions. However, such side information is mostly heterogeneous and multimodal, including both numerical and categorical features to yield a non-trivial incorporation process. Researchers have addressed this problem mainly by converting the categorical features into numerical ones or forming numerical similarity matrices. This paper presents a different approach in the form of a novel Bayesian probabilistic generative framework which can effectively incorporate multimodal side information into the matrix factorization (MF-MSI) model. With the help of local quadratic bounds on the categorical likelihoods, we derive a scalable and computationally efficient iterative optimization method based on the variational EM to learn the posterior distributions of latent variables for both the users and the items. A comprehensive experimental study on both simulated and real benchmark datasets demonstrate the proof-of-concept where the additional side information improves both the prediction accuracy and the ranking performance over more than a dozen popular baseline models. Finally, the proposed MF-MSI model claims state-of-the-art performance in the majority of the test scenarios when compared to more recently introduced recommender systems which can also utilize the side information via different techniques.
更多查看译文
关键词
Side information,Unsupervised learning,Probabilistic generative models,Recommender systems,Collaborative filtering
AI 理解论文
溯源树
样例
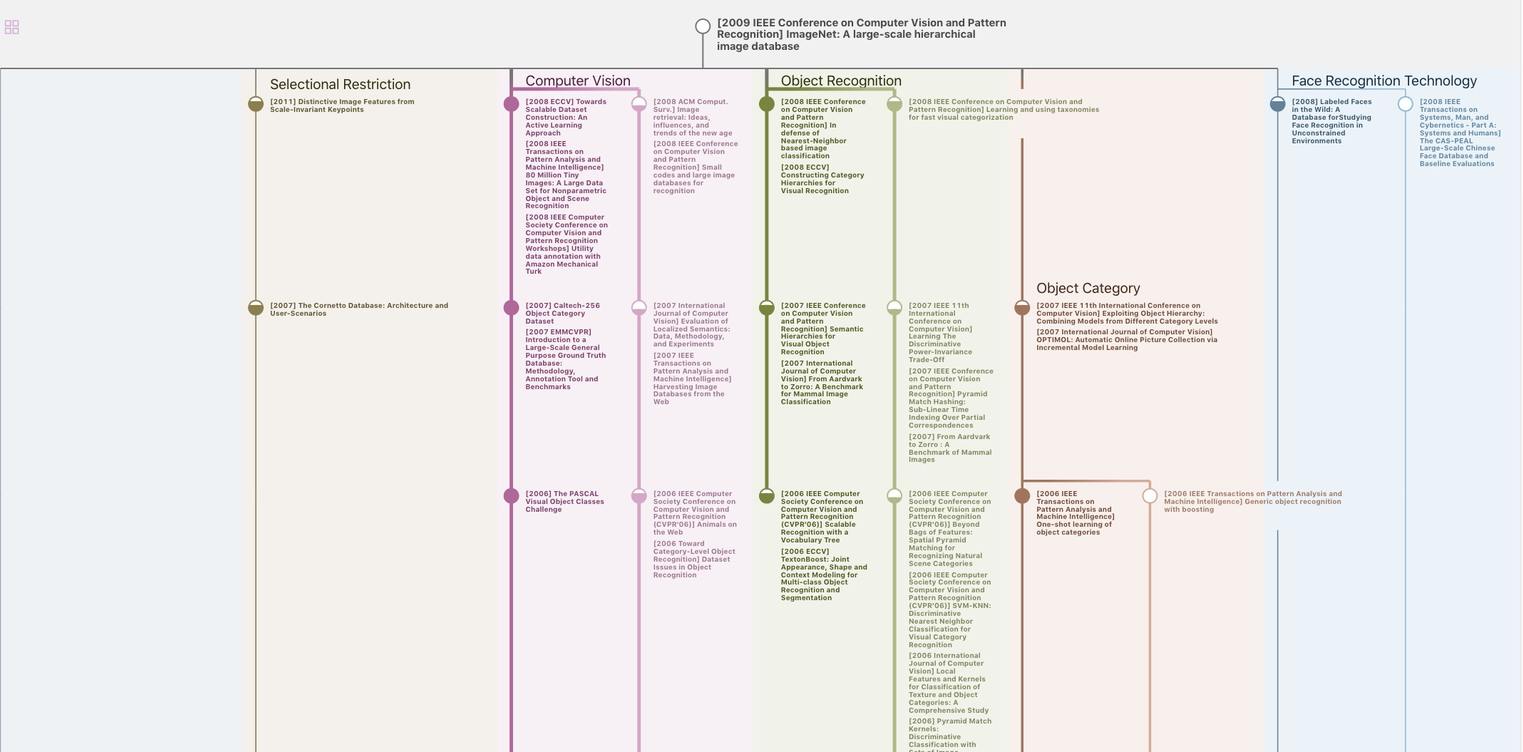
生成溯源树,研究论文发展脉络
Chat Paper
正在生成论文摘要