Convolutional Neural Networks for Food Image Recognition: An Experimental Study
Proceedings of the 5th International Workshop on Multimedia Assisted Dietary Management(2019)
摘要
The global rising trend of consumer health awareness has increasingly drawn attention towards the development of nutrition tracking applications. A key component to enable large scale, low cost and personalized nutrition analysis is a food image recognition engine based on convolutional neural network (CNN). While there is a strong demand for many variants of such applications to serve different localized needs around the world, it remains an ongoing challenge to piece together a complete solution involving (i) dataset preparation (2) choice of CNN architecture (3) CNN optimization strategies in the context of food classification. In this work, we address this problem by conducting a series of experimental study to aid engineers in quickly preparing datasets, deploying and optimizing CNN based solutions for food image recognition tasks. Based on our results, we recommend that (i) larger networks such as Xception shall be used; (ii) minimum of 300 images per category need to be available to kick-start training for optimal performance; (iii) image augmentation techniques that do not alter shapes are more beneficial; various augmentation techniques can also be combined for better results; (iv) dataset balancing strategy might not be required to address class imbalance in food datasets below an imbalance ratio of 7; (v) higher native image resolution during training is beneficial to classifier performance, especially for networks requiring larger input size.
更多查看译文
关键词
class imbalance, convolutional neural networks, food dataset, image augmentation, image recognition
AI 理解论文
溯源树
样例
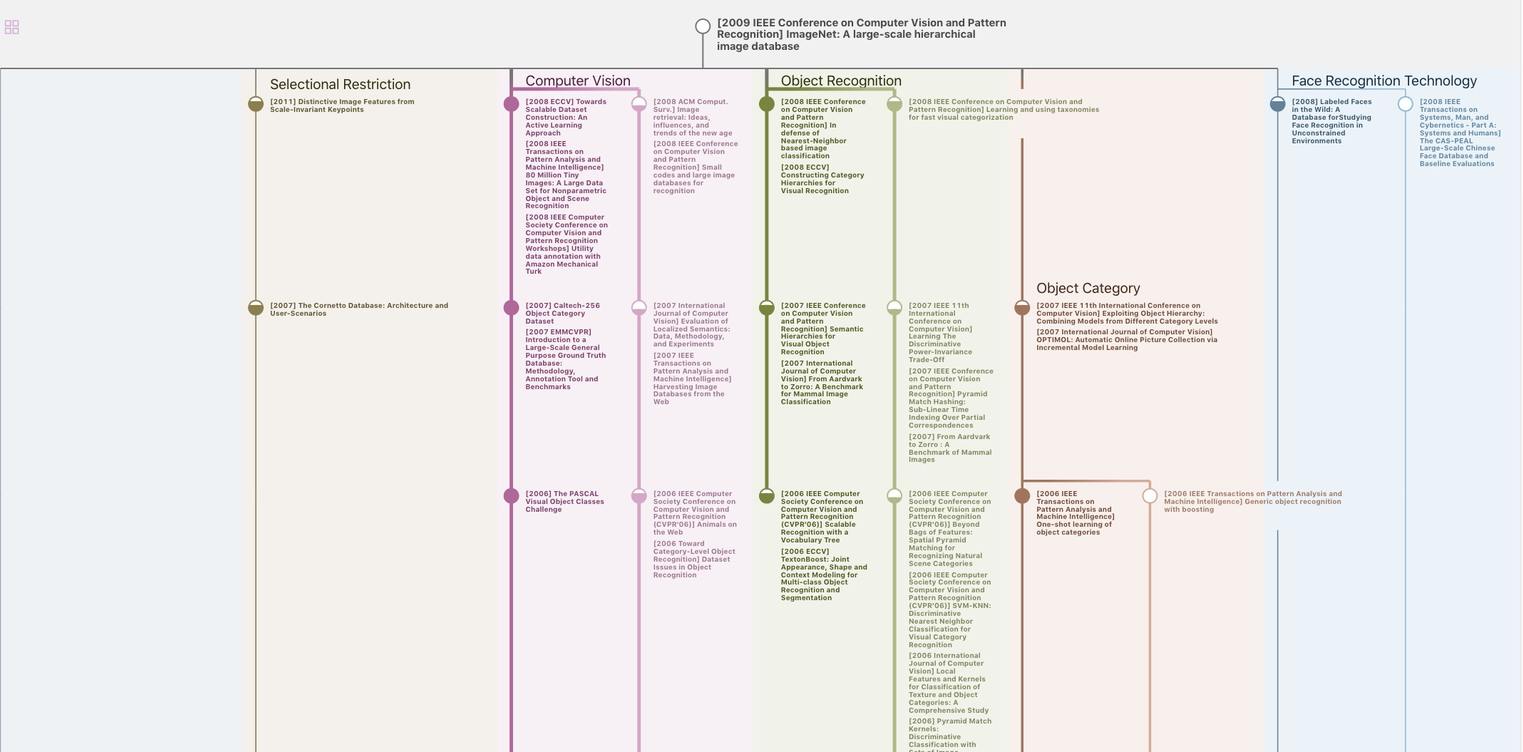
生成溯源树,研究论文发展脉络
Chat Paper
正在生成论文摘要