Inverse Modeling of Hydrologic Parameters in CLM4 via Generalized Polynomial Chaos in the Bayesian Framework
COMPUTATION(2022)
摘要
In this work, generalized polynomial chaos (gPC) expansion for land surface model parameter estimation is evaluated. We perform inverse modeling and compute the posterior distribution of the critical hydrological parameters that are subject to great uncertainty in the Community Land Model (CLM) for a given value of the output LH. The unknown parameters include those that have been identified as the most influential factors on the simulations of surface and subsurface runoff, latent and sensible heat fluxes, and soil moisture in CLM4.0. We set up the inversion problem in the Bayesian framework in two steps: (i) building a surrogate model expressing the input-output mapping, and (ii) performing inverse modeling and computing the posterior distributions of the input parameters using observation data for a given value of the output LH. The development of the surrogate model is carried out with a Bayesian procedure based on the variable selection methods that use gPC expansions. Our approach accounts for bases selection uncertainty and quantifies the importance of the gPC terms, and, hence, all of the input parameters, via the associated posterior probabilities.
更多查看译文
关键词
uncertainty quantification, generalized polynomial chaos, Bayesian inversion, US-ARM, inverse modeling
AI 理解论文
溯源树
样例
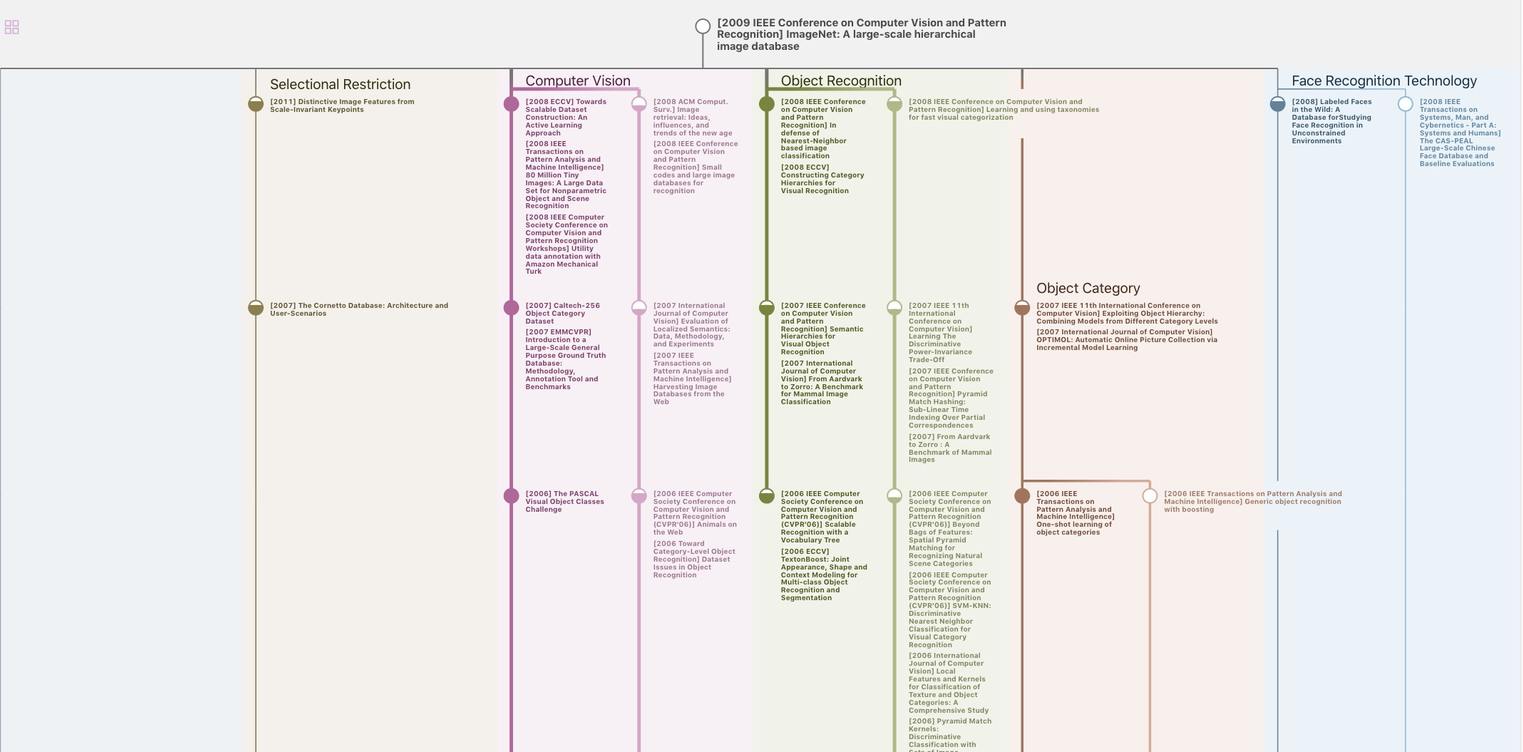
生成溯源树,研究论文发展脉络
Chat Paper
正在生成论文摘要