Leveraging Hierarchical Representations for Preserving Privacy and Utility in Text
2019 IEEE International Conference on Data Mining (ICDM)(2019)
摘要
Guaranteeing a certain level of user privacy in an arbitrary piece of text is a challenging issue. However, with this challenge comes the potential of unlocking access to vast data stores for training machine learning models and supporting data driven decisions. We address this problem through the lens of dx-privacy, a generalization of Differential Privacy to non Hamming distance metrics. In this work, we explore word representations in Hyperbolic space as a means of preserving privacy in text. We provide a proof satisfying dx-privacy, then we define a probability distribution in Hyperbolic space and describe a way to sample from it in high dimensions. Privacy is provided by perturbing vector representations of words in high dimensional Hyperbolic space to obtain a semantic generalization. We conduct a series of experiments to demonstrate the tradeoff between privacy and utility. Our privacy experiments illustrate protections against an authorship attribution algorithm while our utility experiments highlight the minimal impact of our perturbations on several downstream machine learning models. Compared to the Euclidean baseline, we observe > 20x greater guarantees on expected privacy against comparable worst case statistics.
更多查看译文
关键词
privacy,data sanitization,document redaction,privacy analysis
AI 理解论文
溯源树
样例
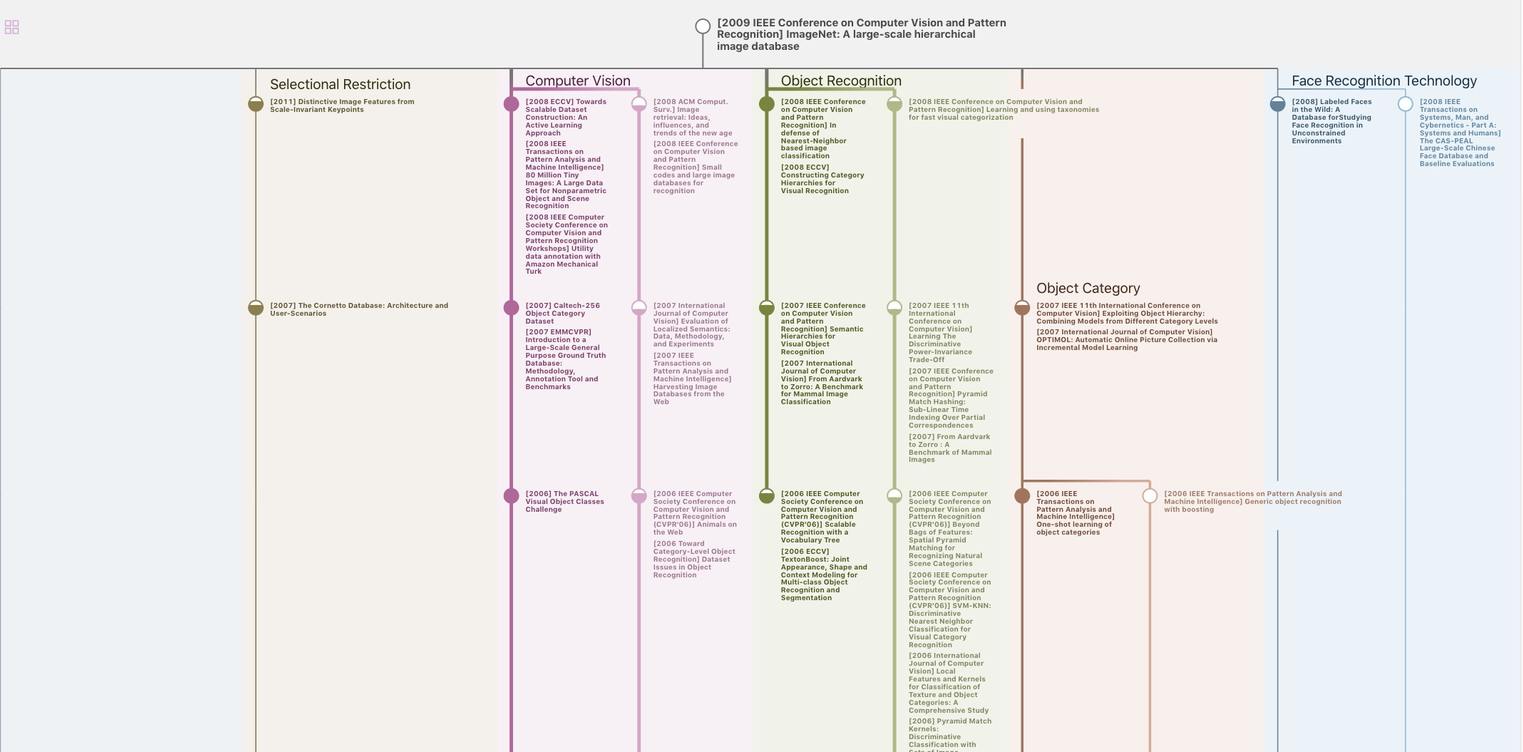
生成溯源树,研究论文发展脉络
Chat Paper
正在生成论文摘要