Subgoal-based Exploration via Bayesian Optimization
arxiv(2022)
摘要
Policy optimization in unknown, sparse-reward environments with expensive and limited interactions is challenging, and poses a need for effective exploration. Motivated by complex navigation tasks that require real-world training (when cheap simulators are not available), we consider an agent that faces an unknown distribution of environments and must decide on an exploration strategy, through a series of training environments, that can benefit policy learning in a test environment drawn from the environment distribution. Most existing approaches focus on fixed exploration strategies, while the few that view exploration as a meta-optimization problem tend to ignore the need for cost-efficient exploration. We propose a cost-aware Bayesian optimization approach that efficiently searches over a class of dynamic subgoal-based exploration strategies. The algorithm adjusts a variety of levers -- the locations of the subgoals, the length of each episode, and the number of replications per trial -- in order to overcome the challenges of sparse rewards, expensive interactions, and noise. Our experimental evaluation demonstrates that, when averaged across problem domains, the proposed algorithm outperforms the meta-learning algorithm MAML by 19%, the hyperparameter tuning method Hyperband by 23%, BO techniques EI and LCB by 24% and 22%, respectively. We also provide a theoretical foundation and prove that the method asymptotically identifies a near-optimal subgoal design from the search space.
更多查看译文
AI 理解论文
溯源树
样例
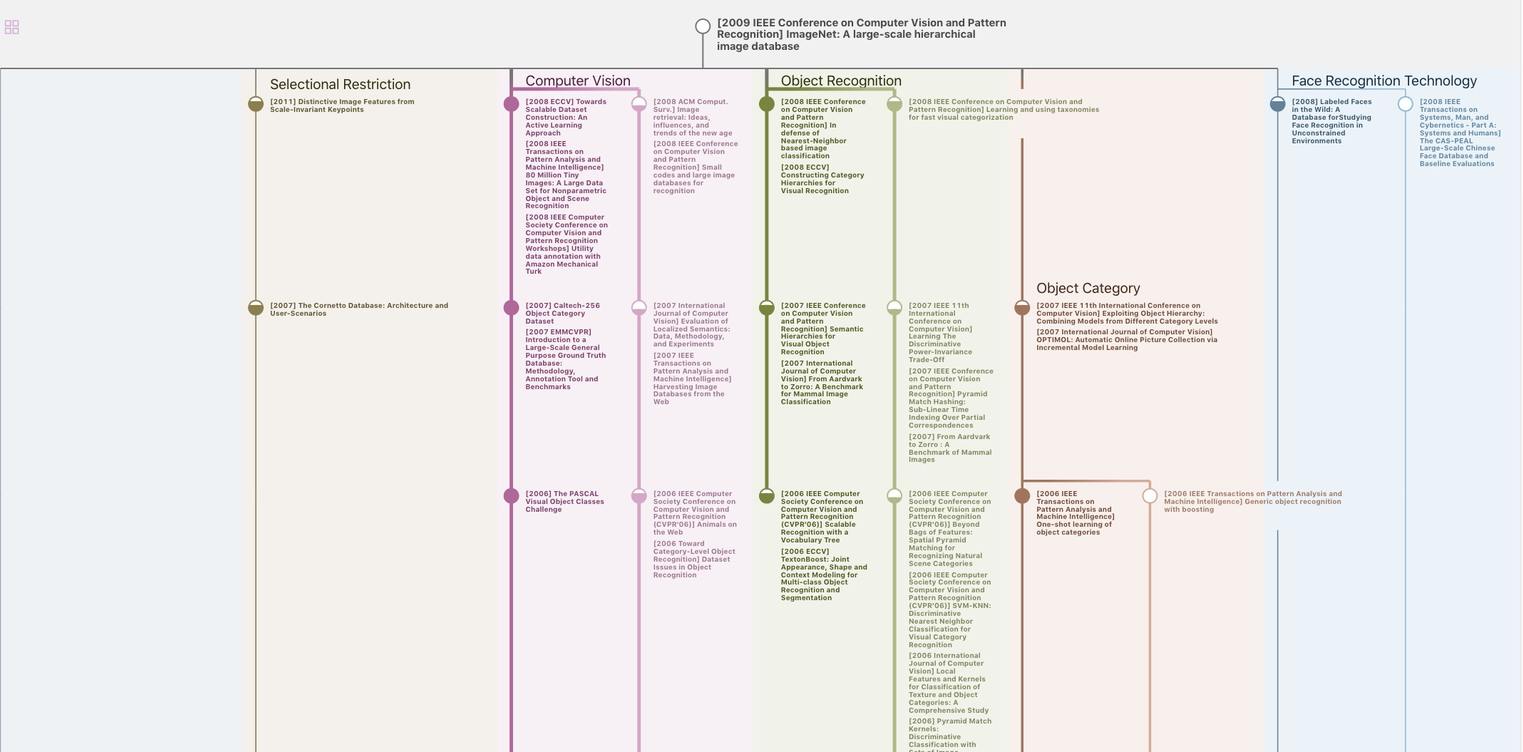
生成溯源树,研究论文发展脉络
Chat Paper
正在生成论文摘要