Sampling random graph homomorphisms and applications to network data analysis
JOURNAL OF MACHINE LEARNING RESEARCH(2023)
摘要
A graph homomorphism is a map between two graphs that preserves adjacency relations. We consider the problem of sampling a random graph homomorphism from a graph into a large network. We propose two complementary MCMC algorithms for sampling random graph homomorphisms and establish bounds on their mixing times and the concentration of their time averages. Based on our sampling algorithms, we propose a novel framework for network data analysis that circumvents some of the drawbacks in methods based on independent and neighborhood sampling. Various time averages of the MCMC trajectory give us various computable observables, including well-known ones such as homomorphism density and average clustering coefficient and their generalizations. Furthermore, we show that these network observables are stable with respect to a suitably renormalized cut dis-tance between networks. We provide various examples and simulations demonstrating our framework through synthetic networks. We also demonstrate the performance of our frame-work on the tasks of network clustering and subgraph classification on the Facebook100 dataset and on Word Adjacency Networks of a set of classic novels.
更多查看译文
关键词
Networks,sampling,graph homomorphism,MCMC,graphons,stability inequalities,hierarchical clustering,subgraph classification
AI 理解论文
溯源树
样例
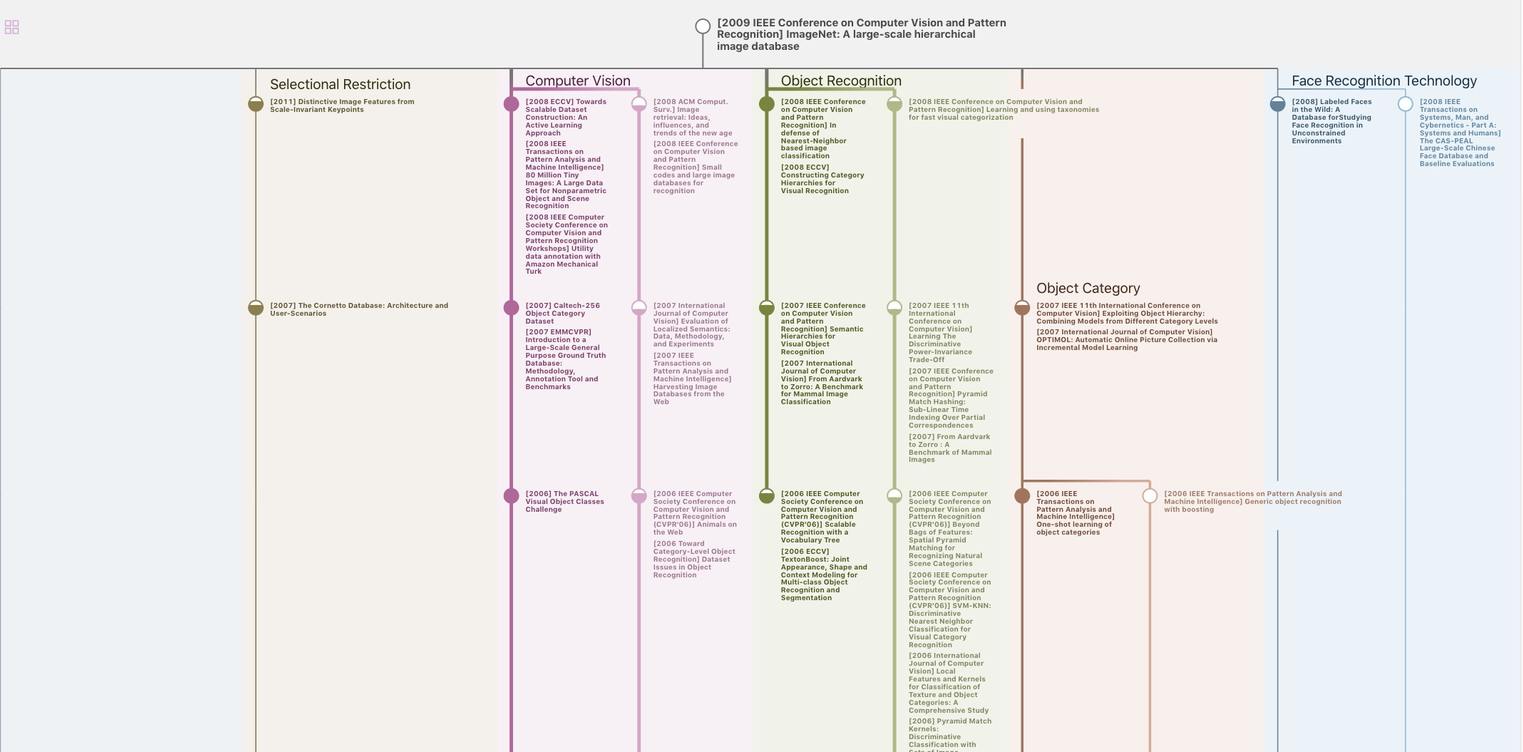
生成溯源树,研究论文发展脉络
Chat Paper
正在生成论文摘要