Close the Gap between Deep Learning and Mobile Intelligence by Incorporating Training in the Loop
Proceedings of the 27th ACM International Conference on Multimedia(2019)
摘要
Pre-trained deep learning models can be deployed on mobile devices to conduct inference. However, they are usually not updated thereafter. In this paper, we take a step further to incorporate training deep neural networks on battery-powered mobile devices and overcome the difficulties from the lack of labeled data. We design and implement a new framework to enlarge sample space via data paring and learn a deep metric under the privacy, memory and computational constraints. A case study of deep behavioral authentication is conducted. Our experiments demonstrate accuracy over 95% on three public datasets, a sheer 15% gain from traditional multi-class classification with less data and robustness against brute-force attacks with 99% success. We demonstrate the training performance on various smartphone models, where training 100 epochs takes less than 10 mins and can be boosted 3-5 times with feature transfer. We also profile memory, energy and computational overhead. Our results indicate that training consumes lower energy than watching videos so can be scheduled intermittently on mobile devices.
更多查看译文
关键词
behavioral authentication, deep metric learning, on-device machine learning, privacy preservation
AI 理解论文
溯源树
样例
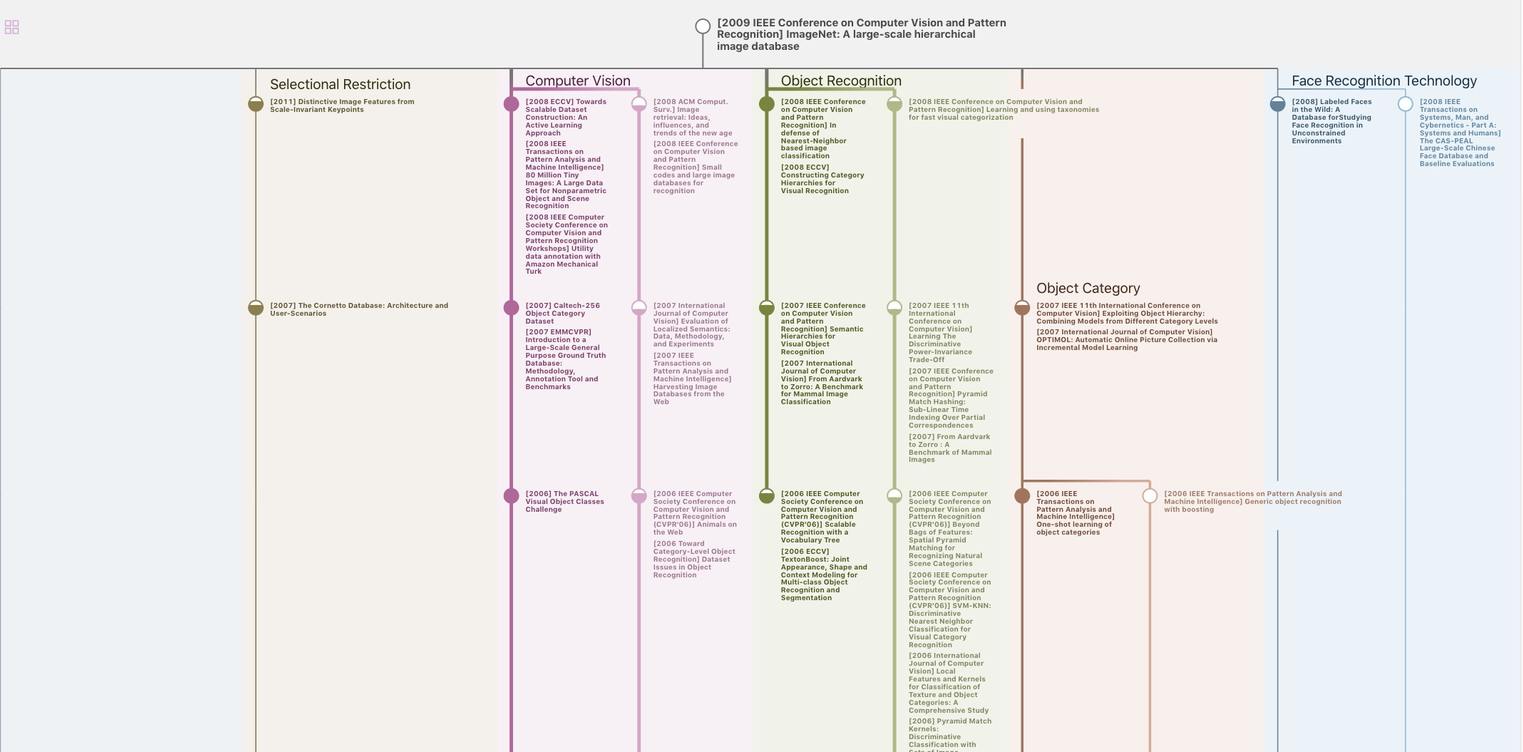
生成溯源树,研究论文发展脉络
Chat Paper
正在生成论文摘要