BraidNet: Braiding Semantics and Details for Accurate Human Parsing
Proceedings of the 27th ACM International Conference on Multimedia(2019)
摘要
This paper focuses on fine-grained human parsing in images. This is a very challenging task due to the diverse person appearance, semantic ambiguity of different body parts and clothing, and extremely small parsing targets. Although existing approaches can achieve significant improvement by pyramid feature learning, multi-level supervision, and joint learning with pose estimation, human parsing is still far from being solved. Different from existing approaches, we propose a Braiding Network, named as BraidNet, to learn complementary semantics and details for fine-grained human parsing. The BraidNet contains a two-stream braid-like architecture. The first stream is a semantic abstracting net with a deep yet narrow structure which can learn semantic knowledge by a hierarchy of fully convolution layers to overcome the challenges of diverse person appearance. To capture low-level details of small targets, the detail-preserving net is designed to exploit a shallow yet wide network without down-sampling, which can retain sufficient local structures for small objects. Moreover, we design a group of braiding modules across the two sub-nets, by which complementary information can be exchanged during end-to-end training. Besides, in the end of BraidNet, a Pairwise Hard Region Embedding strategy is propose to eliminate the semantic ambiguity of different body parts and clothing. Extensive experiments show that the proposed BraidNet achieves better performance than the state-of-the-art methods for fine-grained human parsing.
更多查看译文
关键词
braiding network, fine-grained human parsing, pairwise hard region embedding, semantic segmentation
AI 理解论文
溯源树
样例
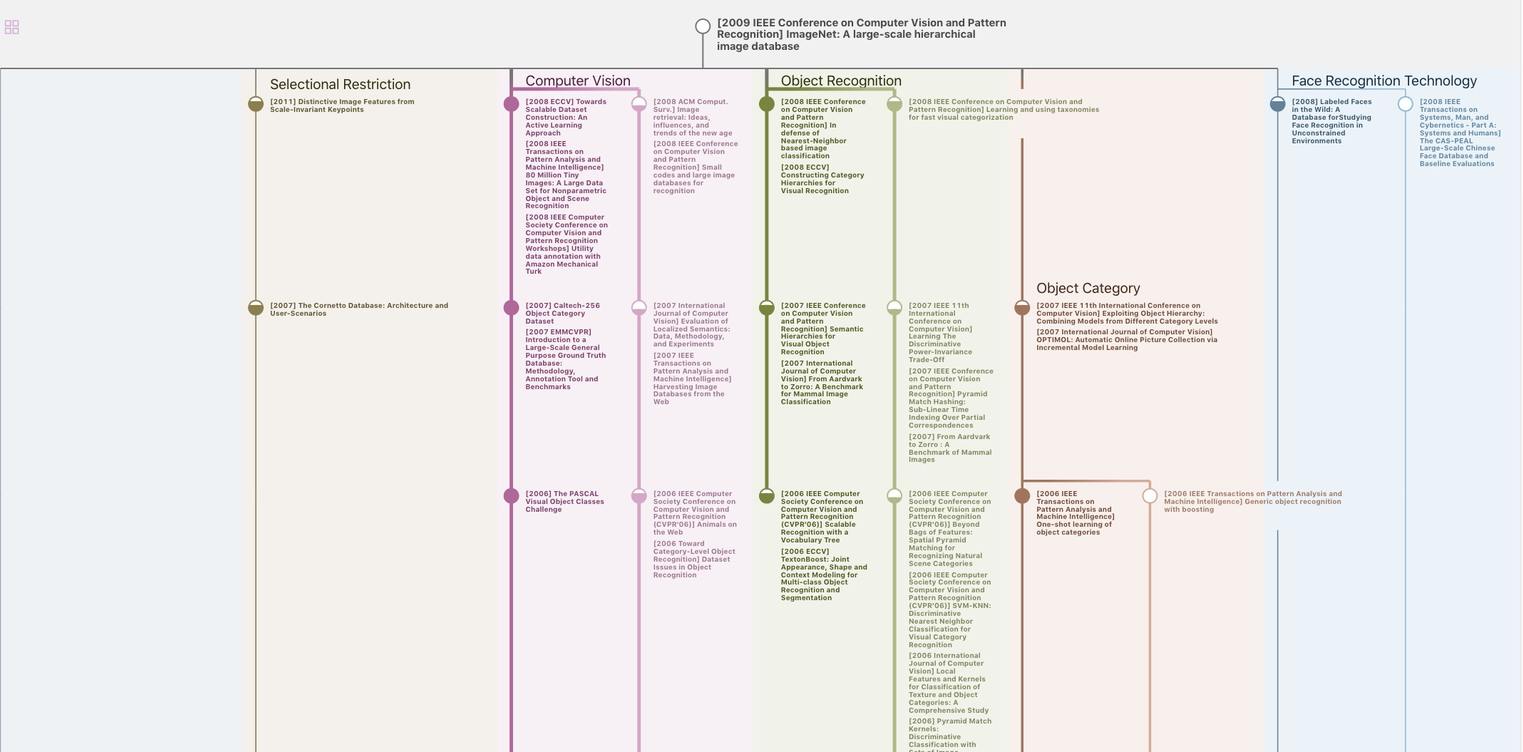
生成溯源树,研究论文发展脉络
Chat Paper
正在生成论文摘要