Cross-Modal Dual Learning for Sentence-to-Video Generation
Proceedings of the 27th ACM International Conference on Multimedia(2019)
摘要
Automatic content generation has become an attractive while challenging topic in the past decade. Generating videos from sentences particularly poses great challenges to the multimedia community due to its multi-modal characteristics in essence, e.g., difficulties in semantic alignment, and the temporal dependencies in video contents. Existing works resort to Variational AutoEncoder (VAE) or Generative Adversary Network (GAN) for generating videos given sentences, which may suffer from either blurry generated videos or unstable training processes as well as difficulties in converging to optimal solutions. In this paper, we propose a cross-modal dual learning (CMDL) algorithm to tackle the challenges in sentence-to-video generation and address the weaknesses in existing works. The proposed CMDL model adopts a dual learning mechanism to simultaneously learn the bidirectional mappings between sentences and videos such that it is able to generate realistic videos which maintain semantic consistencies with their corresponding textual descriptions. By further capturing both global and contextual structures, CMDL employs a multi-scale sentence-to-visual encoder to produce more sequentially consistent and plausible videos. Extensive experiments on various datasets validate the advantages of our proposed CMDL model against several state-of-the-art benchmarks both visually and quantitatively.
更多查看译文
关键词
dual learning, multi-modal understanding, video generation
AI 理解论文
溯源树
样例
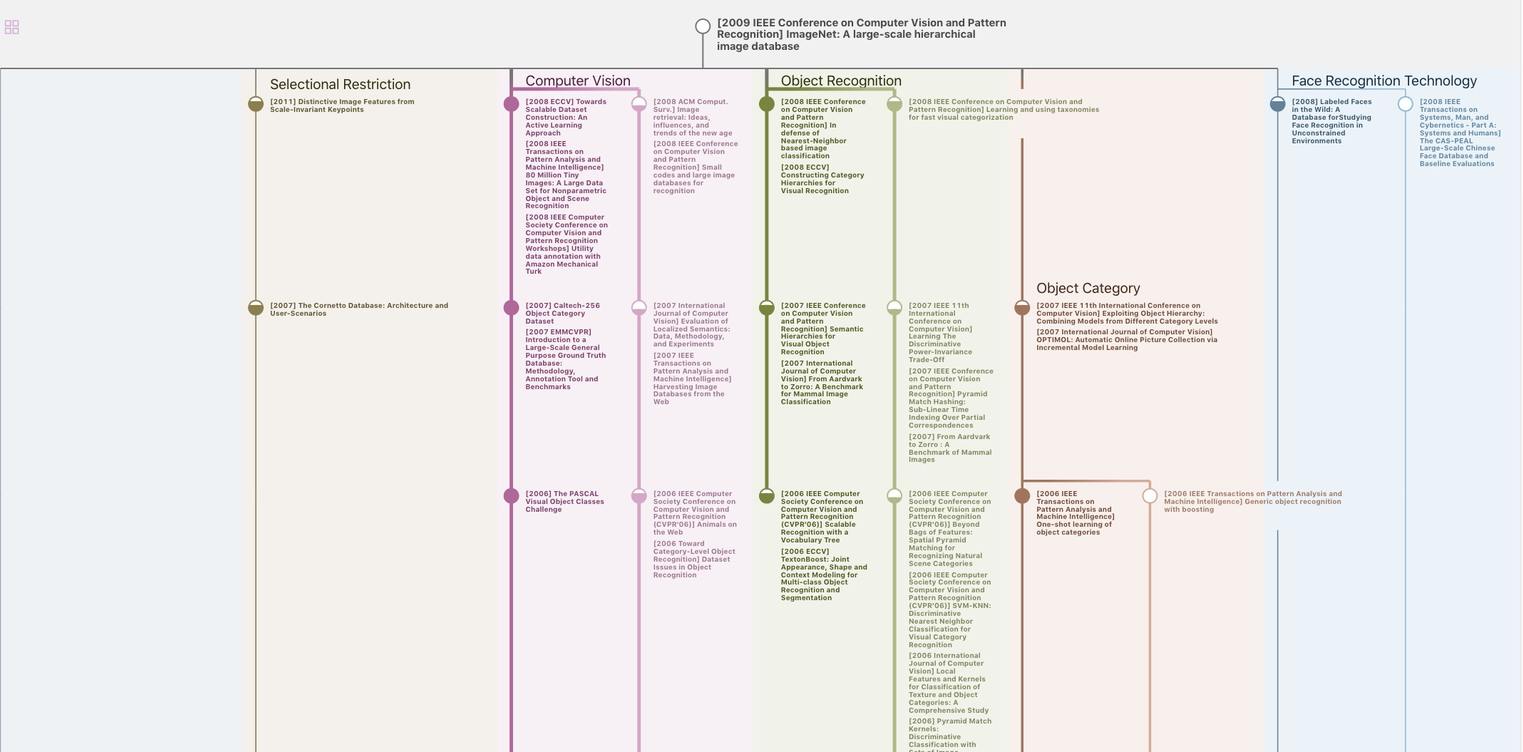
生成溯源树,研究论文发展脉络
Chat Paper
正在生成论文摘要