Self-supervised Representation Learning Using 360° Data
Proceedings of the 27th ACM International Conference on Multimedia(2019)
摘要
The amount of 360-degree panoramas shared online has been rapidly increasing due to the availability of affordable and compact omnidirectional cameras, which offers huge amount of new information unavailable before. In this paper, we present the first work to exploit unlabeled 360-degree data for image representation learning. We propose middle-out, a new self-supervised learning task, which leverages the spatial configuration of normal field-of-view images sampled from a 360-degree image as supervisory signal. We train a Siamese ConvNet model to identify the middle image among three shuffled images sampled from a panorama by perspective projection. Compared to previous self-supervised methods that train models using image patches or video frames with limited field-of-view, our method leverages the rich semantic information contained in 360-degree images and enforces the model to not only learn about objects, but also develop a higher-level understanding about object relationships and scene structures. We quantitatively demonstrate that the feature representation learned using the proposed task is useful for a wide range of vision tasks including object classification, object detection, scene classification, semantic segmentation, and geometry estimation. We also qualitatively show that the proposed method can enforce the ConvNet to extract high-level semantic concepts, an ability which previous self-supervised learning methods have not acquired.
更多查看译文
关键词
360 images, representation learning, self-supervised learning
AI 理解论文
溯源树
样例
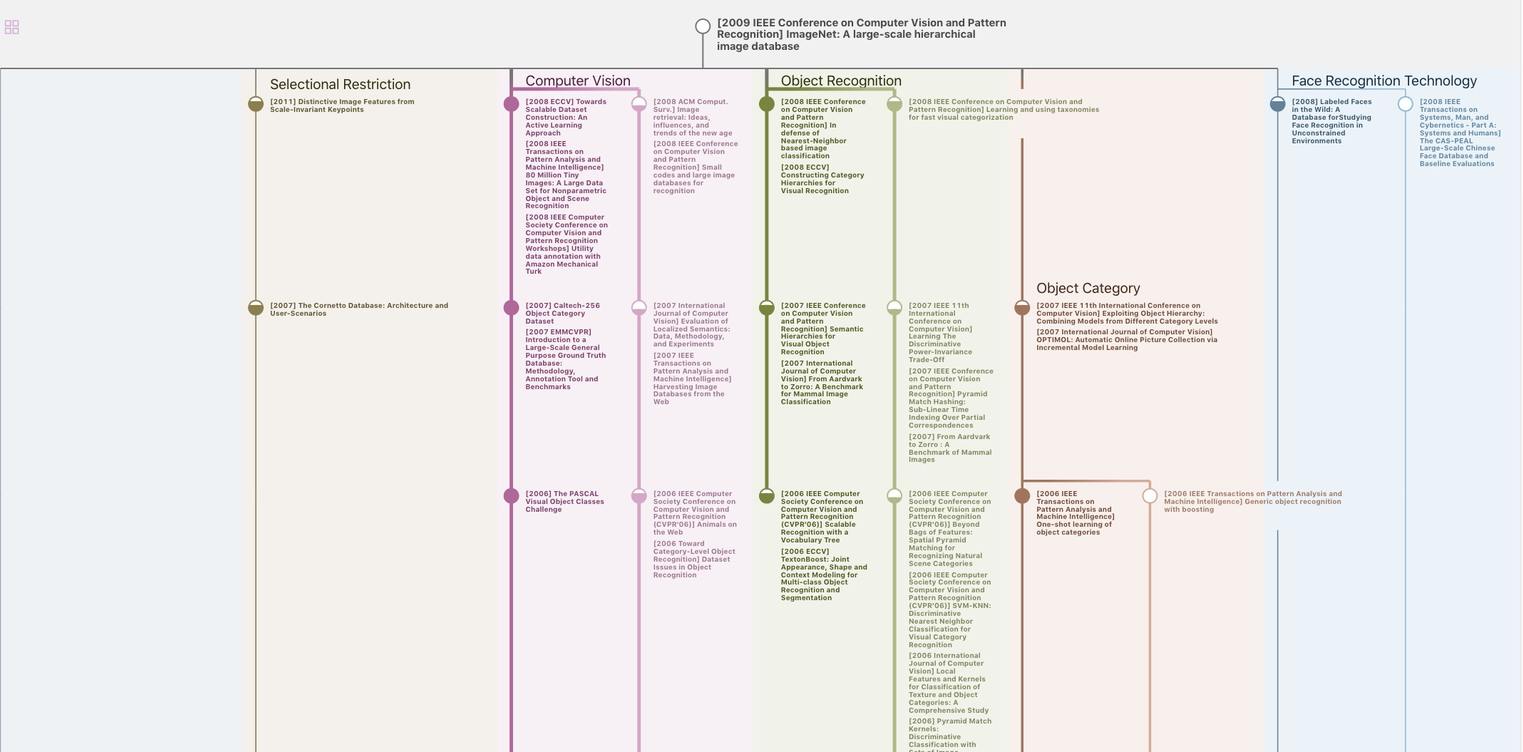
生成溯源树,研究论文发展脉络
Chat Paper
正在生成论文摘要