Objective Bayesian Analysis Of A Cokriging Model For Hierarchical Multifidelity Codes
SIAM-ASA JOURNAL ON UNCERTAINTY QUANTIFICATION(2020)
摘要
Autoregressive cokriging models have been widely used to emulate multiple computer models with different levels of fidelity. The dependence structures are modeled via Gaussian processes at each level of fidelity, where covariance structures are often parameterized up to a few parameters. The predictive distributions typically required intensive Monte Carlo approximations in previous works. This article derives new closed-form formulas to compute the means and variances of predictive distributions in autoregressive cokriging models that only depend on correlation parameters. For parameter estimation, we consider objective Bayesian analysis of such autoregressive cokriging models. We show that common choices of prior distributions, such as the constant prior and inverse correlation prior, typically lead to improper posteriors. We also develop several objective priors such as the independent reference prior and the independent Jeffreys prior that are shown to yield proper posterior distributions. This development is illustrated with a borehole function in an eight-dimensional input space and applied to an engineering application in a six-dimensional input space.
更多查看译文
关键词
autoregressive cokriging, multifidelity computer models, objective priors, recursive prediction
AI 理解论文
溯源树
样例
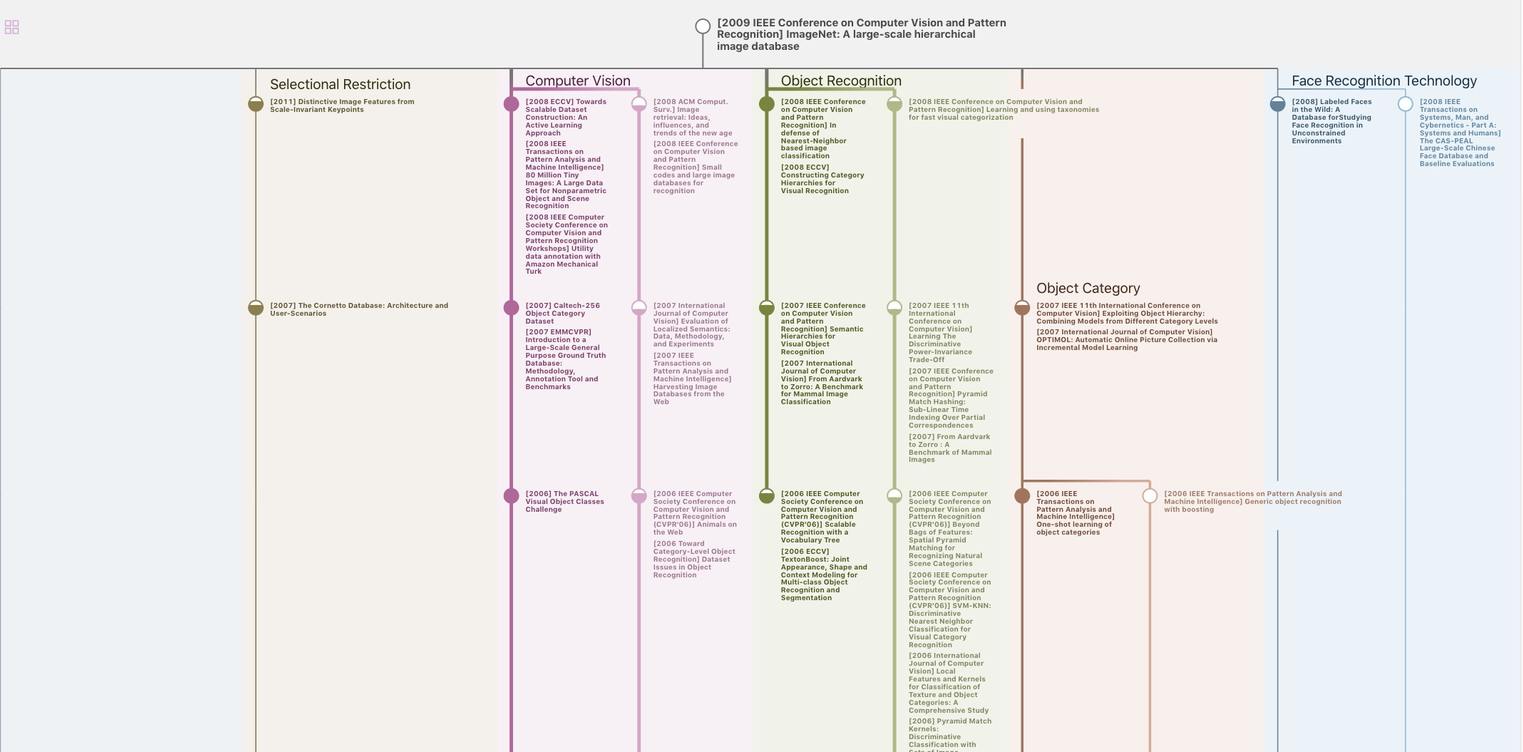
生成溯源树,研究论文发展脉络
Chat Paper
正在生成论文摘要