Occlusions for Effective Data Augmentation in Image Classification
2019 IEEE/CVF INTERNATIONAL CONFERENCE ON COMPUTER VISION WORKSHOPS (ICCVW)(2019)
摘要
Deep networks for visual recognition are known to leverage "easy to recognise" portions of objects such as faces and distinctive texture patterns. The lack of a holistic understanding of objects may increase fragility and overfitting. In recent years, several papers have proposed to address this issue by means of occlusions as a form of data augmentation. However, successes have been limited to tasks such as weak localization and model interpretation, but no benefit was demonstrated on image classification on large-scale datasets. In this paper, we show that, by using a simple technique based on batch augmentation, occlusions as data augmentation can result in better performance on ImageNet for high-capacity models (e.g., ResNet50). We also show that varying amounts of occlusions used during training can be used to study the robustness of different neural network architectures.
更多查看译文
关键词
interpretability,explainability,classification,computer-vision,deep-learning
AI 理解论文
溯源树
样例
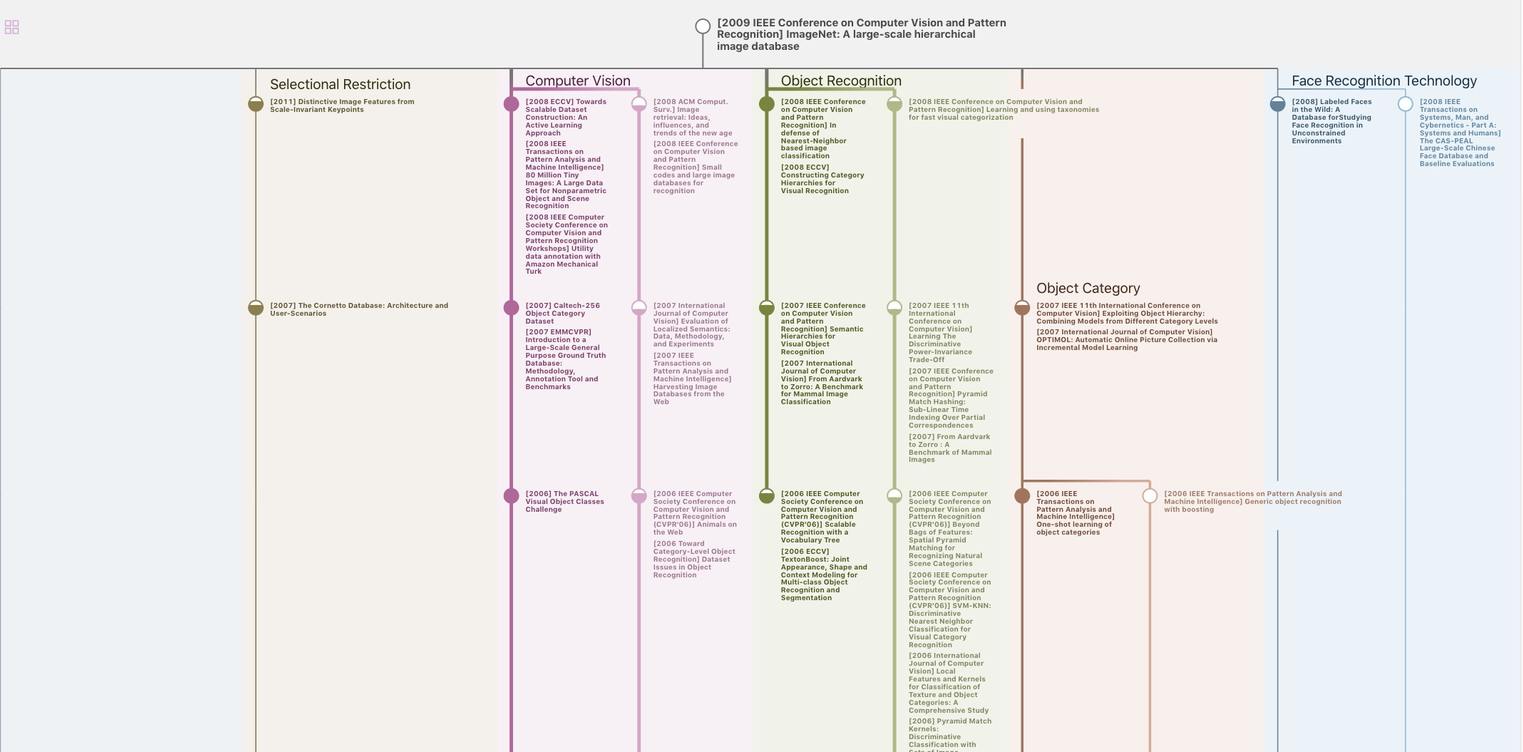
生成溯源树,研究论文发展脉络
Chat Paper
正在生成论文摘要