Instance-Based Model Adaptation For Direct Speech Translation
2020 IEEE INTERNATIONAL CONFERENCE ON ACOUSTICS, SPEECH, AND SIGNAL PROCESSING(2020)
摘要
Despite recent technology advancements, the effectiveness of neural approaches to end-to-end speech-to-text translation is still limited by the paucity of publicly available training corpora. We tackle this limitation with a method to improve data exploitation and boost the system's performance at inference time. Our approach allows us to customize "on the fly" an existing model to each incoming translation request. At its core, it exploits an instance selection procedure to retrieve, from a given pool of data, a small set of samples similar to the input query in terms of latent properties of its audio signal. The retrieved samples are then used for an instance-specific fine-tuning of the model. We evaluate our approach in three different scenarios. In all data conditions (different languages, in/out-of-domain adaptation), our instance-based adaptation yields coherent performance gains over static models.
更多查看译文
关键词
End-to-end neural speech translation
AI 理解论文
溯源树
样例
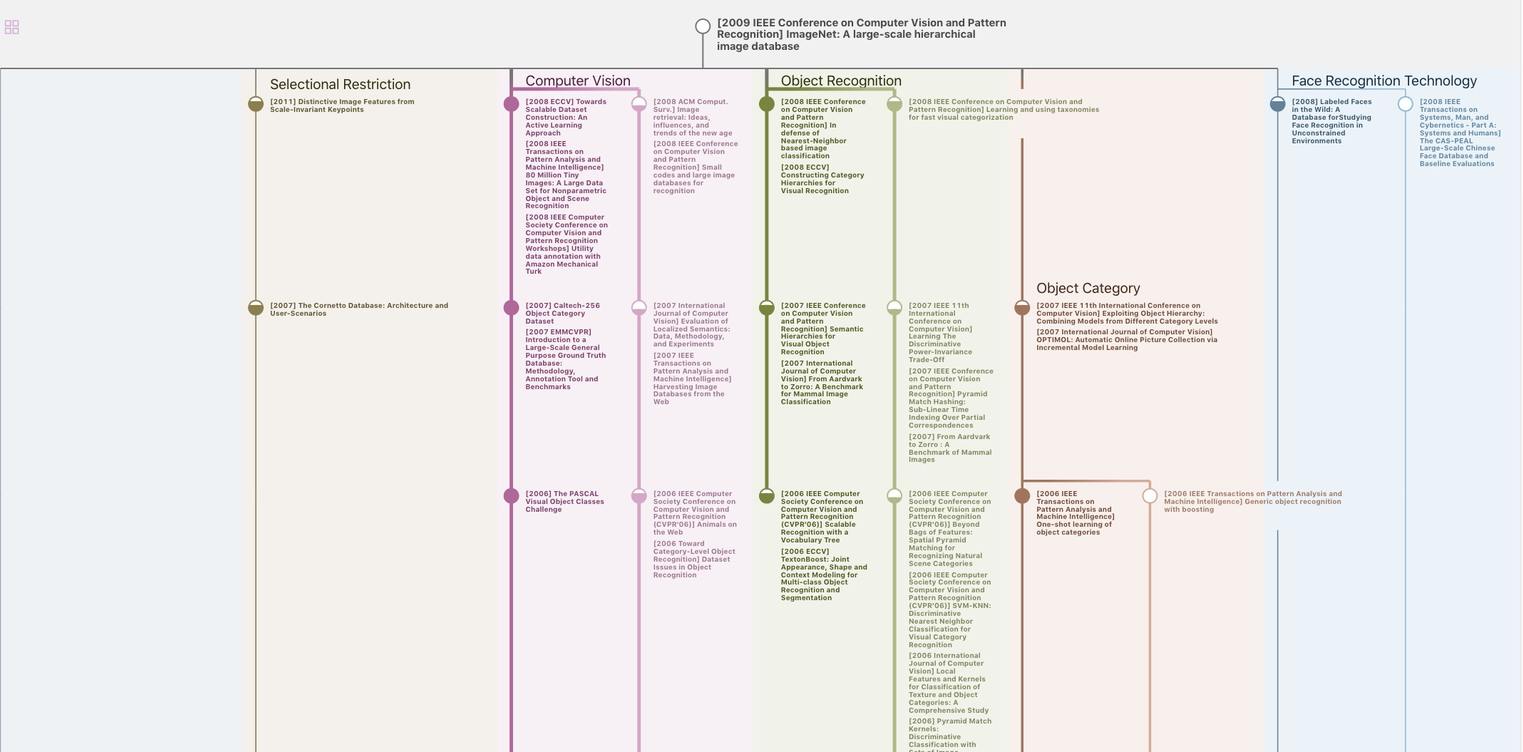
生成溯源树,研究论文发展脉络
Chat Paper
正在生成论文摘要