Large scale model predictive control with neural networks and primal active sets
Automatica(2022)
摘要
This work presents an explicit–implicit procedure to compute a model predictive control (MPC) law with guarantees on recursive feasibility and asymptotic stability. The approach combines an offline-trained fully-connected neural network with an online primal active set solver. The neural network provides a control input initialization while the primal active set method ensures recursive feasibility and asymptotic stability. The neural network is trained with a primal–dual loss function, aiming to generate control sequences that are primal feasible and meet a desired level of suboptimality. Since the neural network alone does not guarantee constraint satisfaction, its output is used to warm start the primal active set method online. We demonstrate that this approach scales to large problems with thousands of optimization variables, which are challenging for current approaches. Our method achieves a 2× reduction in online inference time compared to the best method in a benchmark suite of different solver and initialization strategies.
更多查看译文
关键词
Fully connected neural network,Primal active set method,Model predictive control,Receding horizon control
AI 理解论文
溯源树
样例
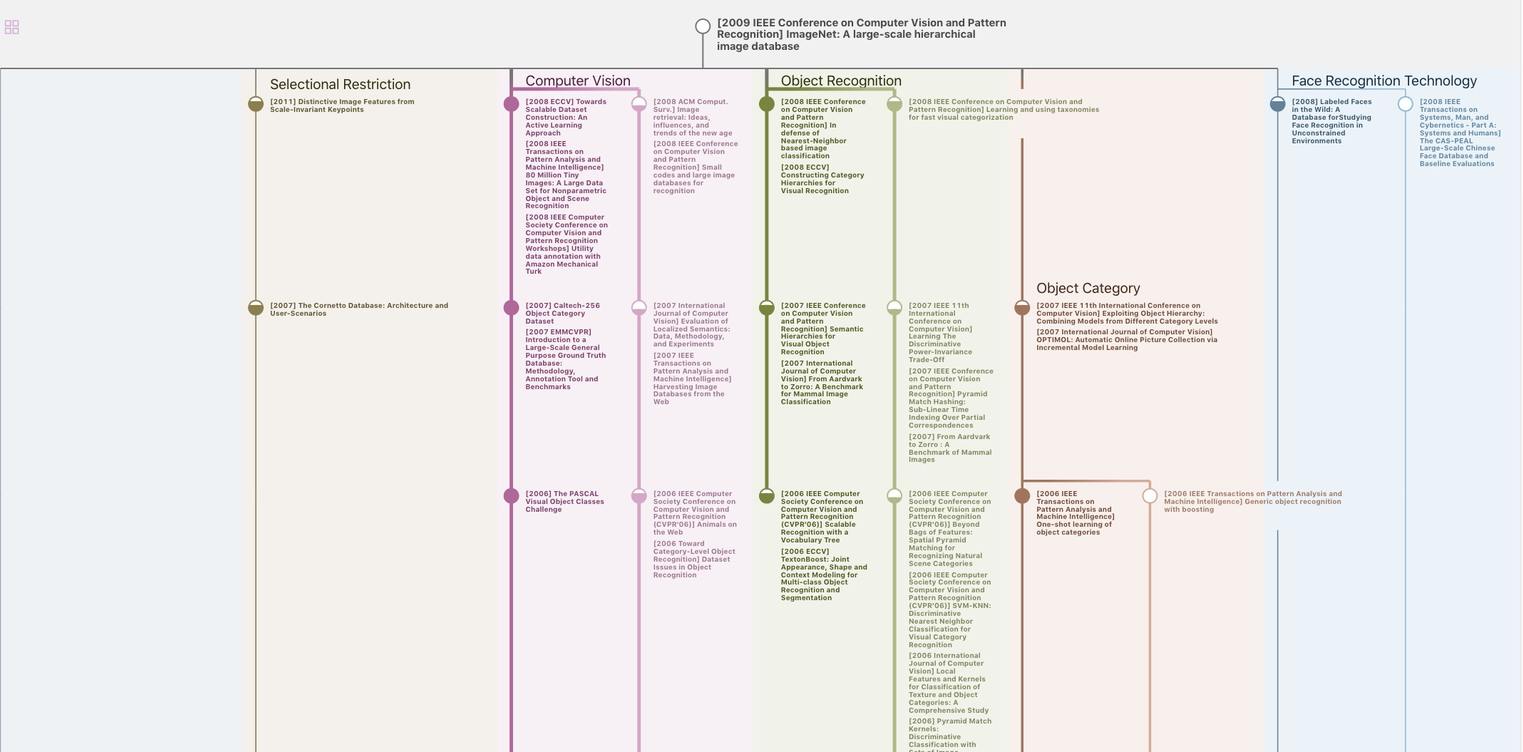
生成溯源树,研究论文发展脉络
Chat Paper
正在生成论文摘要