Adaptive and Iteratively Improving Recurrent Lateral Connections
arxiv(2019)
摘要
The current leading computer vision models are typically feed forward neural models, in which the output of one computational block is passed to the next one sequentially. This is in sharp contrast to the organization of the primate visual cortex, in which feedback and lateral connections are abundant. In this work, we propose a computational model for the role of lateral connections in a given block, in which the weights of the block vary dynamically as a function of its activations, and the input from the upstream blocks is iteratively reintroduced. We demonstrate how this novel architectural modification can lead to sizable gains in performance, when applied to visual action recognition without pretraining and that it outperforms the literature architectures with recurrent feedback processing on ImageNet.
更多查看译文
AI 理解论文
溯源树
样例
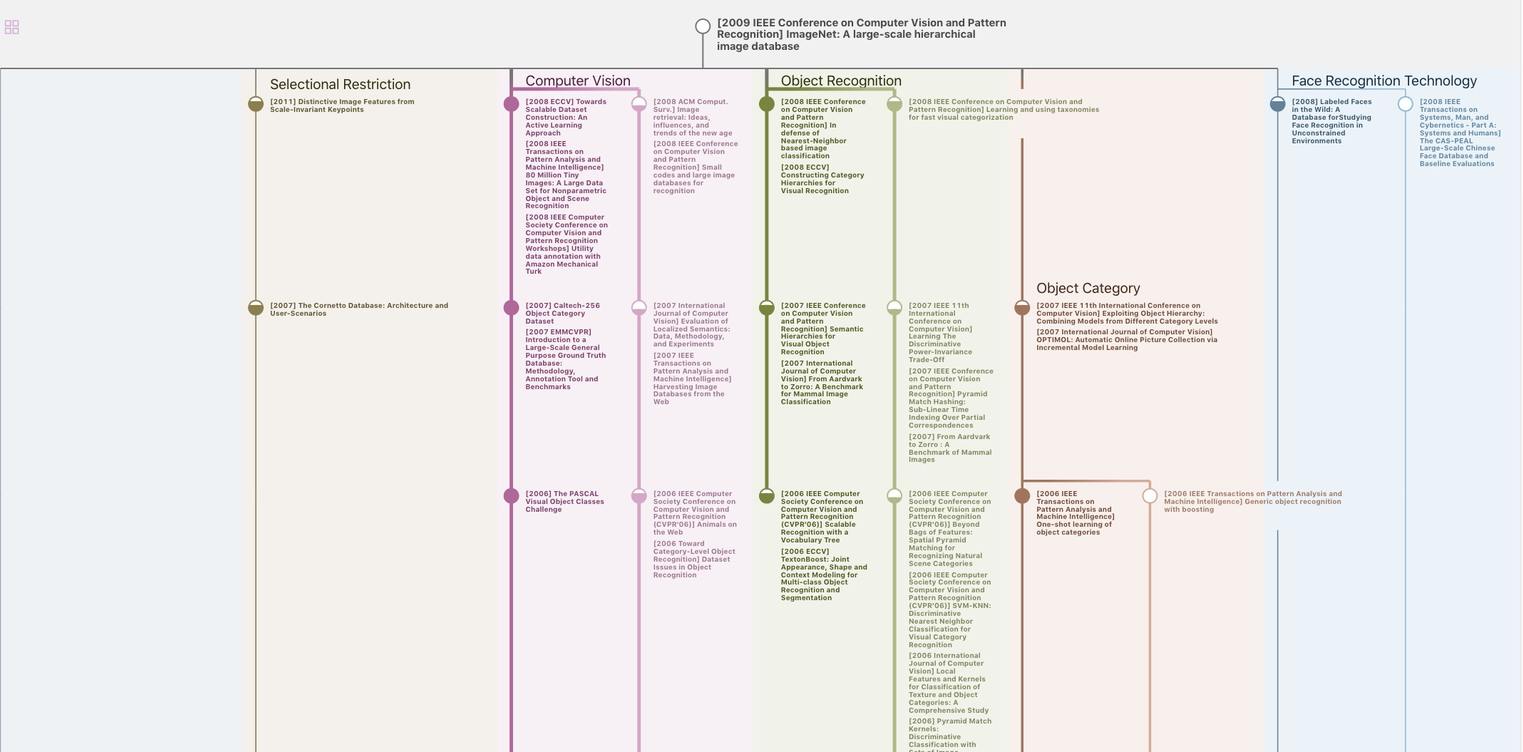
生成溯源树,研究论文发展脉络
Chat Paper
正在生成论文摘要