Fractal Features Combined with Local Phase Information in Texture Analysis
2019 11th International Symposium on Image and Signal Processing and Analysis (ISPA)(2019)
摘要
This paper concerns processing, analysis and classification of fully-textured images, which contain both natural stochastic texture (NST) and structured information (SI). Whereas the fractal attributes of Gaussianity and self-similarity, that characterize purely NST, are fully represented by the spatial spectrum, and the corresponding spatial phase of the NST is random, the structural, edge-and-curve-type, information is mostly represented by the local spatial phase. We therefore decompose textured images into two layers, corresponding to the NST and SI, respectively. We show that the extent to which the texture obeys the fractal property of self-similarity, can be assessed by the mutual information that exists between scales. The phase of this layer, in the spatial Fourier domain, is random and exhibit approximately uniform distribution. We confirm this random behavior using the Kolmagorov-Smirnov hypothesis testing framework. We proceed to construct a classification space that combines features/dimensions that characterize the two layers of the stochastic (fBm-type spectrum) and deterministic (phase) attributes of partial spatial information, and address the implementation of this space in classification of fully-textured images encountered in modeling and analysis of images in various fields by providing an example.
更多查看译文
关键词
Classification,fractals,Hurst parameter,local phase,stochastic textures
AI 理解论文
溯源树
样例
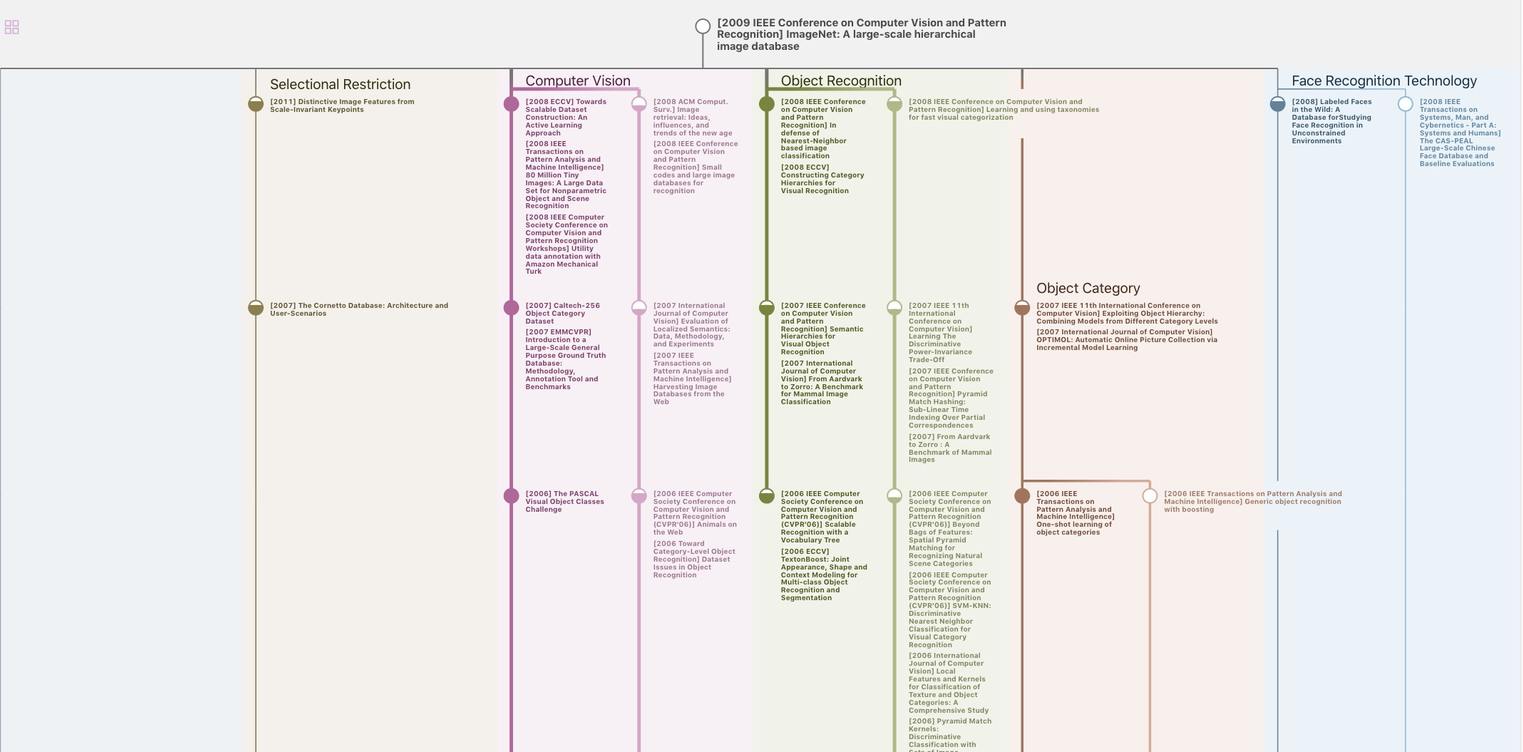
生成溯源树,研究论文发展脉络
Chat Paper
正在生成论文摘要