Object Detection in Satellite Images Based on Active Learning Utilizing Visual Explanation
2019 11th International Symposium on Image and Signal Processing and Analysis (ISPA)(2019)
摘要
Convolutional neural networks (CNNs) have attracted much attention for object detection in satellite images. However, creating an annotated dataset requires a lot of time and user workload due to which the remote sensing domain has insufficient labeled datasets for training CNNs. We exploit an active learning (AL) framework for training CNNs with a small labeled dataset. AL obtains the training dataset by asking a human user to label the samples. For efficient AL, an intelligent query strategy is essential because the performance of a CNN depends on the collected dataset. Thus, in this study, we propose a query strategy to train CNNs effectively; this is done by choosing effective samples for training both the classifier and feature extractor. The strategy selects samples according to the gap between the classifier's prediction and visual explanation, which is the class discriminative part of an image derived from the extracted feature maps. Experimental result shows that a CNN trained with samples queried by our strategy had a 95% reduction in training samples requirement while maintaining 94% detection performance compared to a CNN trained with a complete dataset. Furthermore, the proposed strategy also reduced the required training samples by 30% compared to the conventional strategy to yield the same performance.
更多查看译文
关键词
Active learning,Visual explanation,Annotation,Object detection,Satellite images
AI 理解论文
溯源树
样例
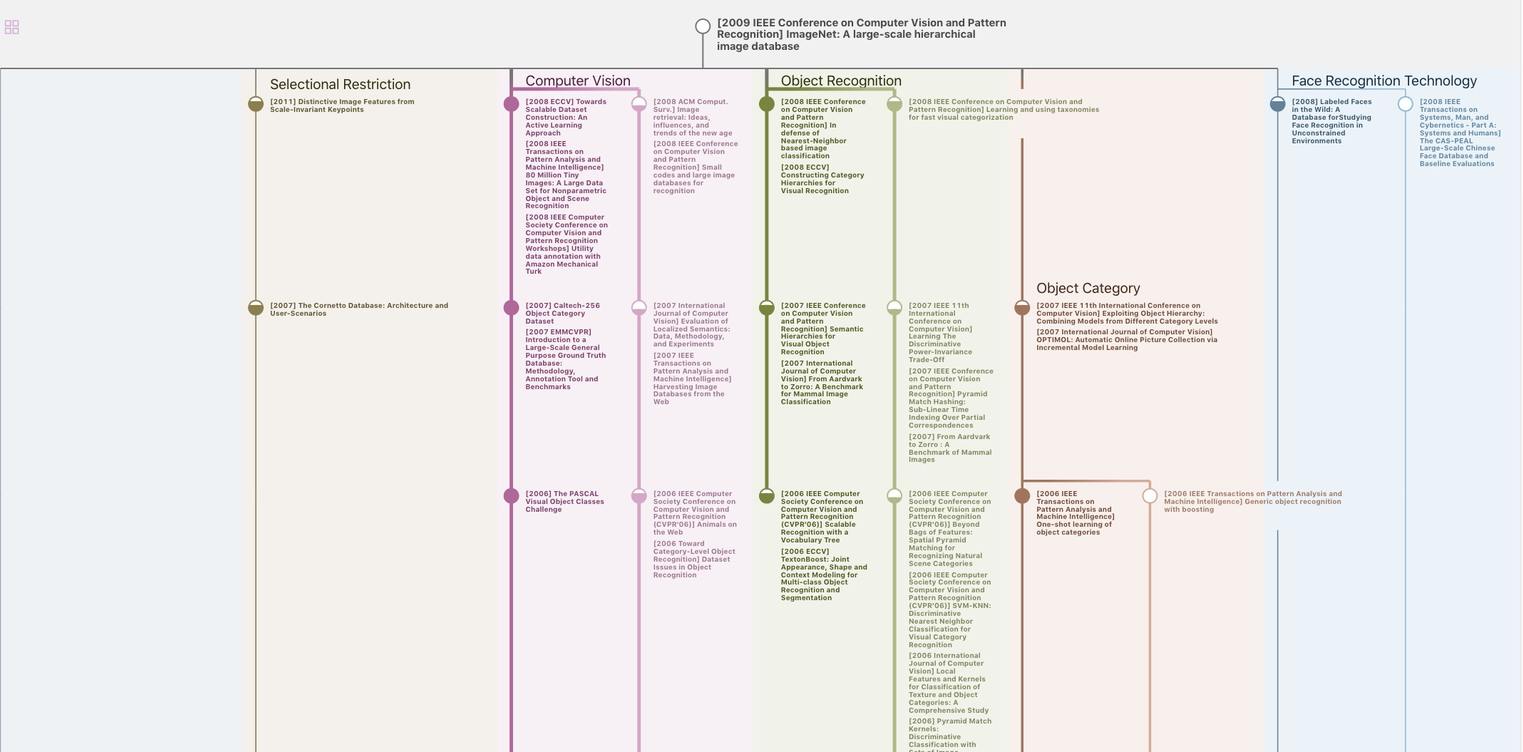
生成溯源树,研究论文发展脉络
Chat Paper
正在生成论文摘要