Regression-Based Three-Dimensional Pose Estimation for Texture-Less Objects
IEEE Transactions on Multimedia(2019)
摘要
3-D pose estimation for texture-less objects remains a challenging problem. Previous works either focus on a template matching method to find the nearest template as a candidate, or construct a Hough forest, which utilizes the offset of patches to vote for the object location and pose. By contrast, in this paper, we propose a comprehensive framework to directly regress 3-D poses for the candidates, in which a convolutional neural network-based triplet network is trained to extract discriminating features from the binary images. To make the features suitable for the regression task, a pose-guided method and a regression constraint are employed with the constructed triplet network. We show that the constraint reaches the goal of creating the correlation between the features and 3-D poses. Once the expected features are obtained, the object pose could be efficiently regressed, by training a regression network with a simple structure. For symmetric objects, depth images are treated as an additional channel to feed the triplet network. Experiments on the LineMOD and our own datasets demonstrate our method with high regression precision and efficiency.
更多查看译文
关键词
Three-dimensional displays,Pose estimation,Feature extraction,Training,Image edge detection,Correlation,Cost function
AI 理解论文
溯源树
样例
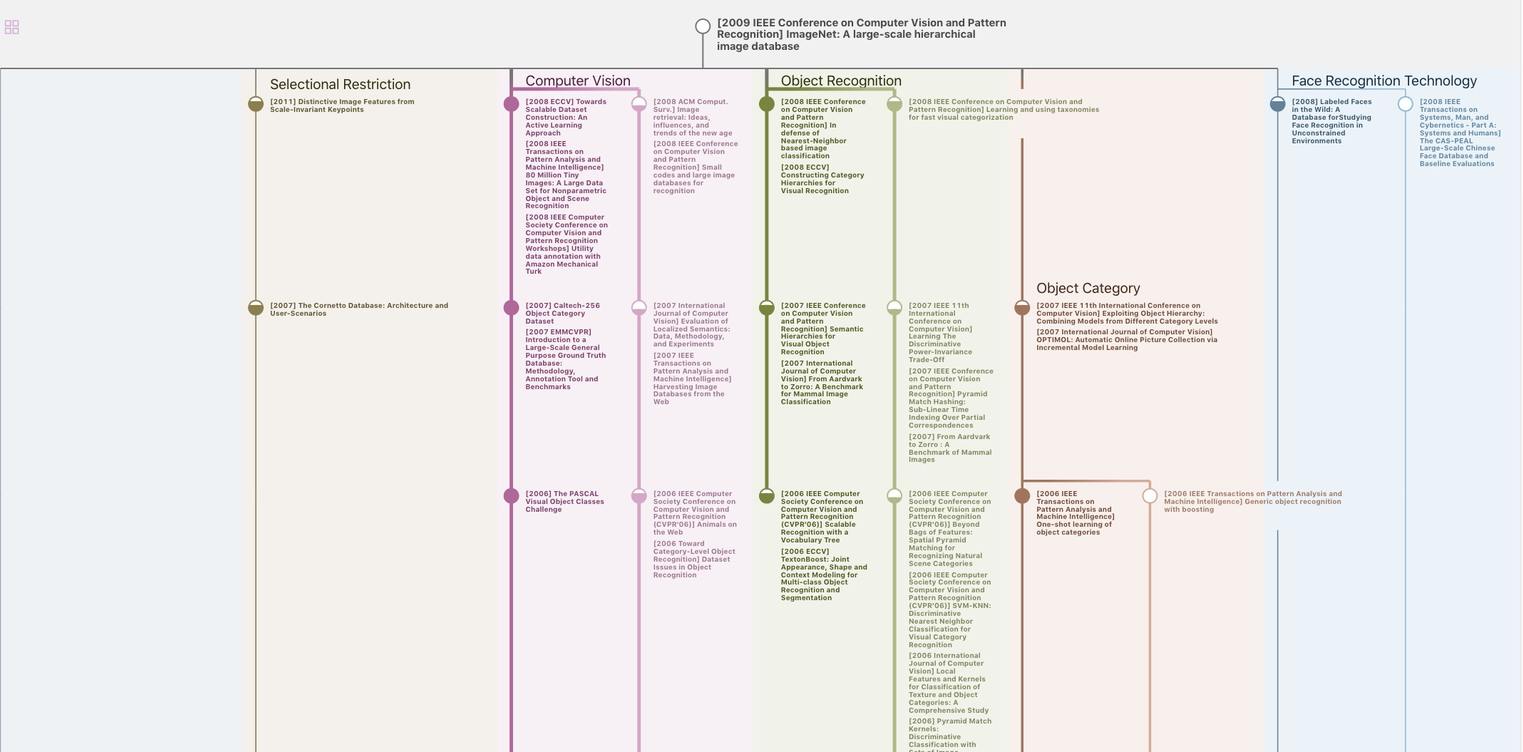
生成溯源树,研究论文发展脉络
Chat Paper
正在生成论文摘要