Kernel Kalman Filtering With Conditional Embedding and Maximum Correntropy Criterion
IEEE Transactions on Circuits and Systems I: Regular Papers(2019)
摘要
The Hilbert space embedding provides a powerful and flexible tool for dealing with the nonlinearity and high-order statistics of random variables in a dynamical system. The kernel Kalman filtering based on the conditional embedding operator (KKF-CEO) shows significant performance improvements over the traditional Kalman filters in the noisy nonlinear time-series prediction. However, KKF-CEO based on the minimum mean-square-error (MMSE) criterion is sensitive to the outliers or heavy-tailed noises. In contrast to the MMSE criterion, the maximum correntropy criterion (MCC) can achieve more robust performance in the presence of outliers. In this paper, we develop a novel kernel Kalman-type filter based on MCC, referred to kernel Kalman filtering with conditional embedding operator and maximum correntropy criterion (KKF-CEO-MCC). The proposed KKF-CEO-MCC can capture higher order statistics of errors and is robust to outliers. In addition, two simplified versions of KKF-CEO-MCC are developed, namely, KKF-CEO-MCC-O and KKF-CEO-MCC-NA. The former is an online approach and the latter is based on Nyström approximation. Simulations on noisy nonlinear time-series prediction confirm the desirable accuracy and robustness of the new filters.
更多查看译文
关键词
Kernel,Kalman filters,Noise measurement,Cost function,Random variables,Predictive models,Estimation
AI 理解论文
溯源树
样例
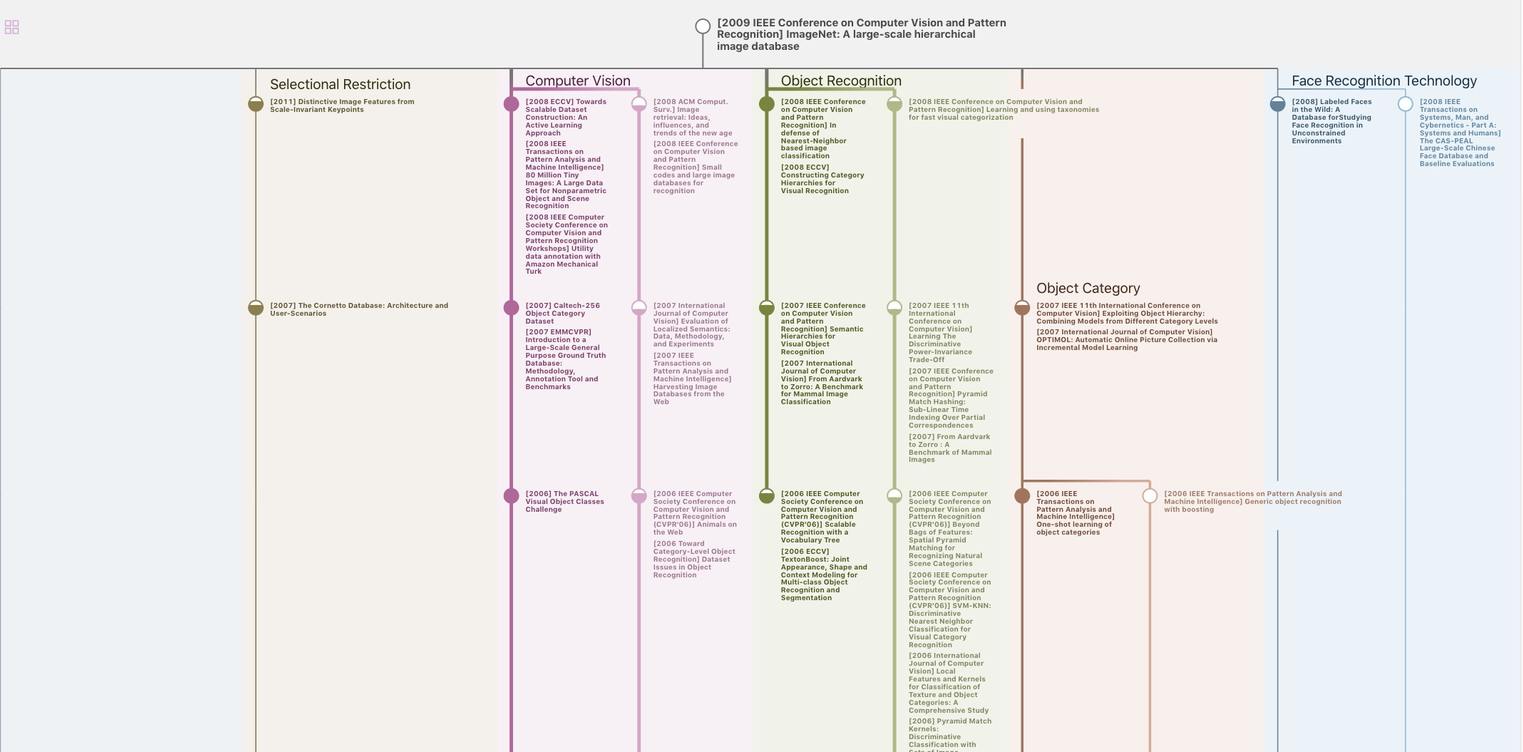
生成溯源树,研究论文发展脉络
Chat Paper
正在生成论文摘要