Feature Enhancement With Deep Feature Losses For Speaker Verification
2020 IEEE INTERNATIONAL CONFERENCE ON ACOUSTICS, SPEECH, AND SIGNAL PROCESSING(2020)
摘要
Speaker Verification still suffers from the challenge of generalization to novel adverse environments. We leverage on the recent advancements made by deep learning based speech enhancement and propose a feature-domain supervised denoising based solution. We propose to use Deep Feature Loss which optimizes the enhancement network in the hidden activation space of a pre-trained auxiliary speaker embedding network. We experimentally verify the approach on simulated and real data. A simulated testing setup is created using various noise types at different SNR levels. For evaluation on real data, we choose BabyTrain corpus which consists of children recordings in uncontrolled environments. We observe consistent gains in every condition over the state-of-the-art augmented Factorized-TDNN x-vector system. On BabyTrain corpus, we observe relative gains of 10.38% and 12.40% in minDCF and EER respectively.
更多查看译文
关键词
Feature Enhancement, Speech Enhancement, Speaker Verification, Deep Feature Loss, Perceptual Loss
AI 理解论文
溯源树
样例
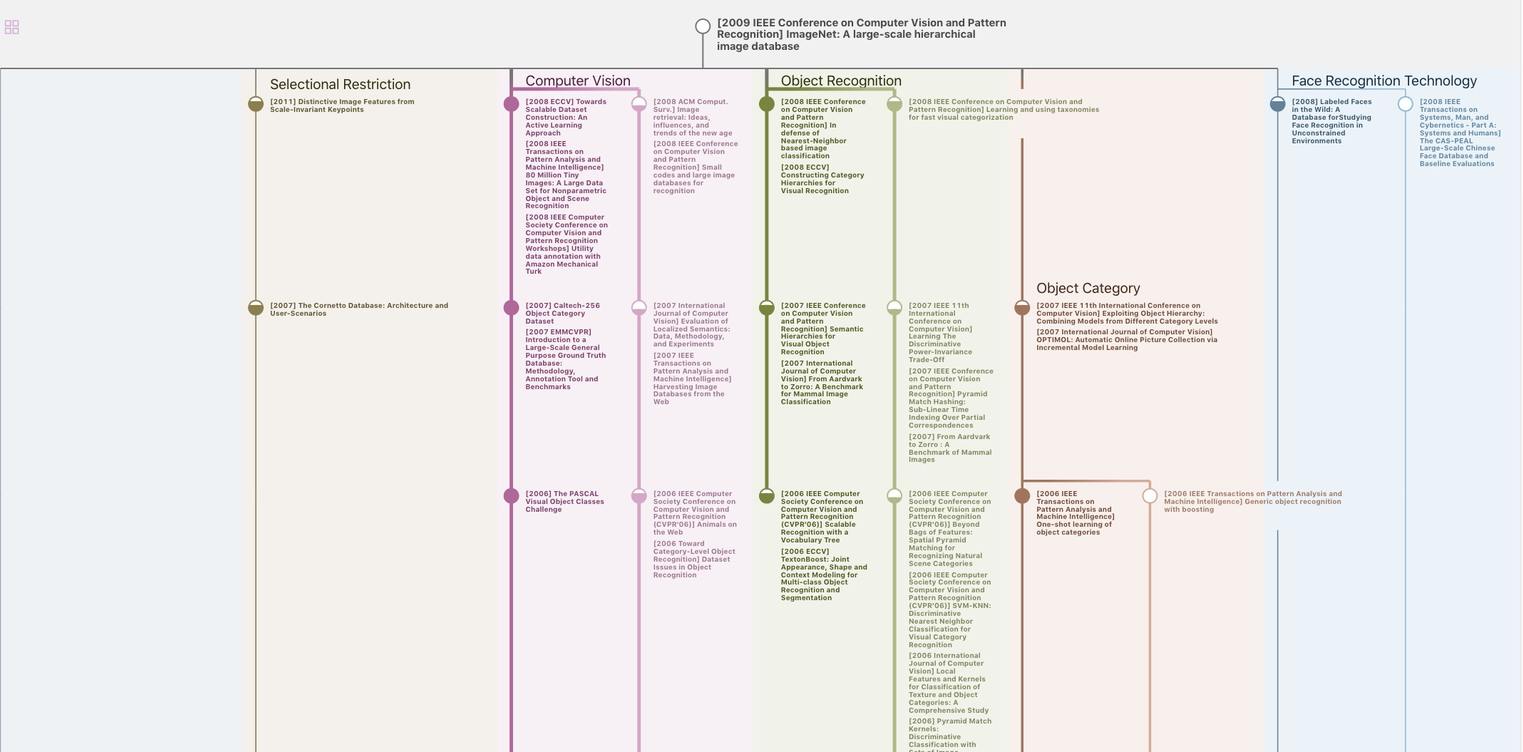
生成溯源树,研究论文发展脉络
Chat Paper
正在生成论文摘要