A Gibbs sampler for a class of random convex polytopes.
Journal of the American Statistical Association(2021)
摘要
We present a Gibbs sampler for the Dempster-Shafer (DS) approach to statistical inference for Categorical distributions. The DS framework extends the Bayesian approach, allows in particular the use of partial prior information, and yields three-valued uncertainty assessments representing probabilities "for", "against", and "don't know" about formal assertions of interest. The proposed algorithm targets the distribution of a class of random convex polytopes which encapsulate the DS inference. The sampler relies on an equivalence between the iterative constraints of the vertex configuration and the non-negativity of cycles in a fully connected directed graph. Illustrations include the testing of independence in 2 × 2 contingency tables and parameter estimation of the linkage model.
更多查看译文
关键词
Algorithms,Bayesian methods,Categorical data analysis,Simulation
AI 理解论文
溯源树
样例
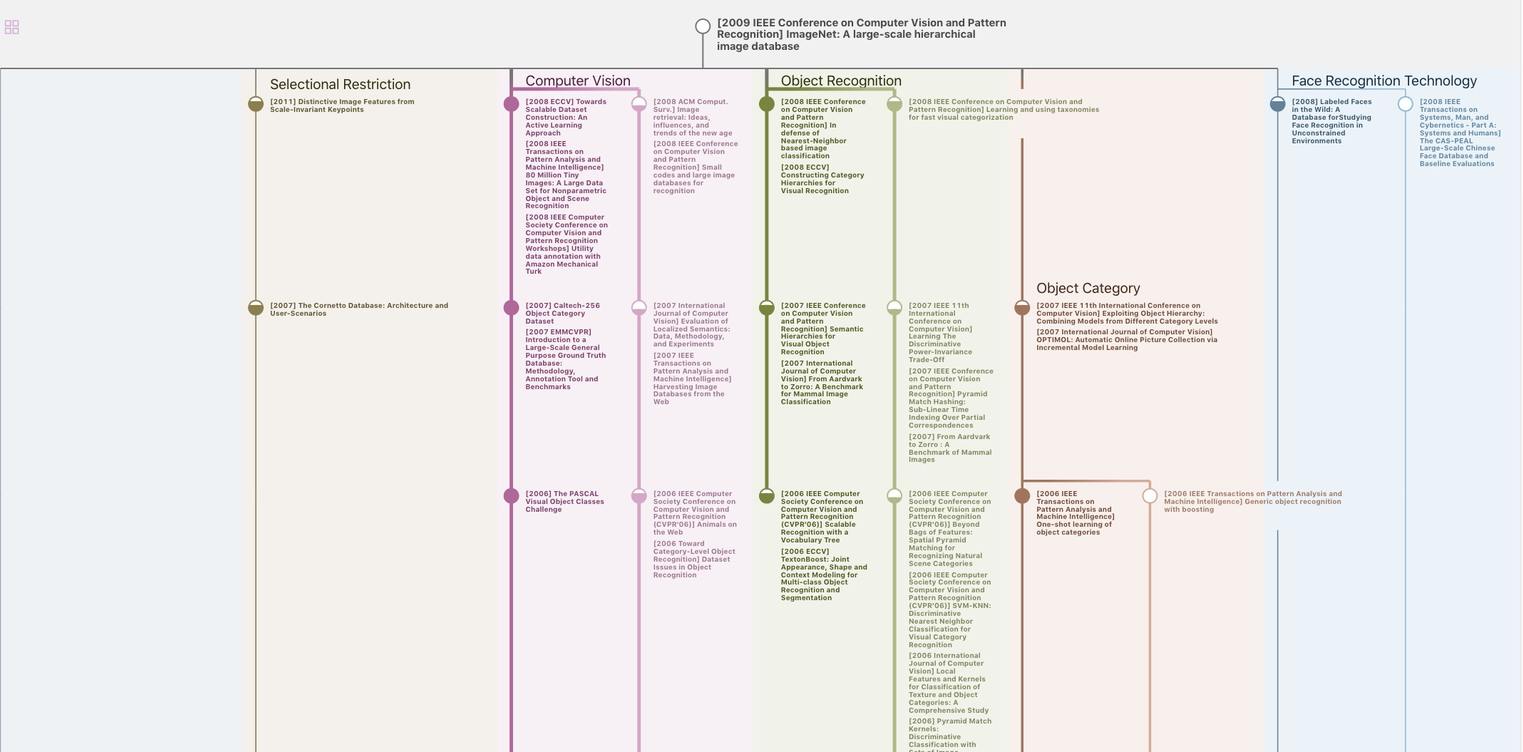
生成溯源树,研究论文发展脉络
Chat Paper
正在生成论文摘要