Ecological Regression with Partial Identification
POLITICAL ANALYSIS(2020)
摘要
Ecological inference (EI) is the process of learning about individual behavior from aggregate data. We relax assumptions by allowing for "linear contextual effects," which previous works have regarded as plausible but avoided due to nonidentification, a problem we sidestep by deriving bounds instead of point estimates. In this way, we offer a conceptual framework to improve on the Duncan-Davis bound, derived more than 65 years ago. To study the effectiveness of our approach, we collect and analyze 8,430 2\times 2 EI datasets with known ground truth from several sources-thus bringing considerably more data to bear on the problem than the existing dozen or so datasets available in the literature for evaluating EI estimators. For the 88% of real data sets in our collection that fit a proposed rule, our approach reduces the width of the Duncan-Davis bound, on average, by about 44%, while still capturing the true district-level parameter about 99% of the time. The remaining 12% revert to the Duncan-Davis bound.
更多查看译文
关键词
asymptotics,bounds,confidence intervals,contextual models,ecological inference,linear regression,partial identification
AI 理解论文
溯源树
样例
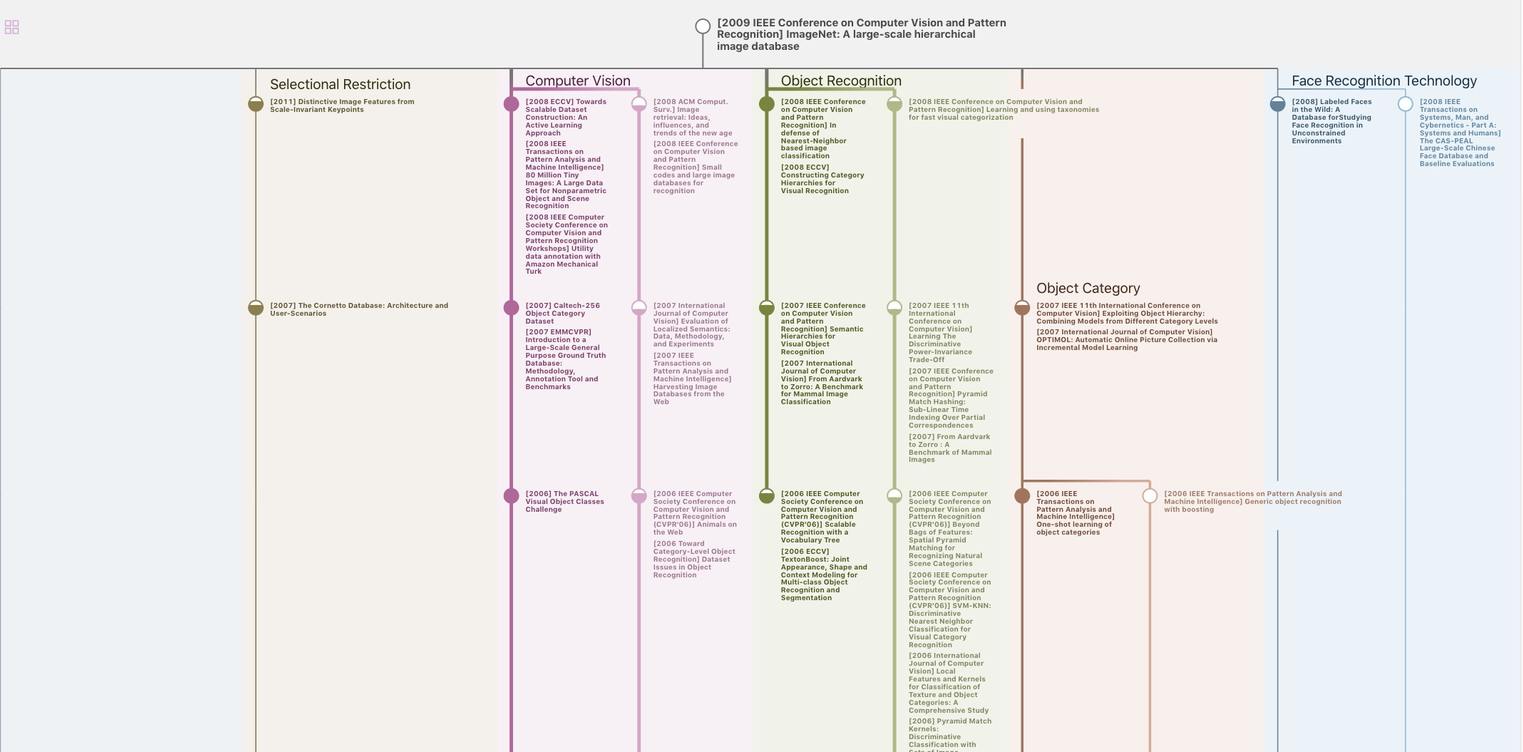
生成溯源树,研究论文发展脉络
Chat Paper
正在生成论文摘要