Hyperspectral and LiDAR Classification With Semisupervised Graph Fusion
IEEE Geoscience and Remote Sensing Letters(2020)
摘要
To fuse hyperspectral and Light Detection And Ranging (LiDAR), we propose a semisupervised graph fusion (SSGF) approach. We apply morphological filters to LiDAR and the first few components of hyperspectral data to model the height and spatial information, respectively. Then, the proposed SSGF is used to project the spectral, elevation, and spatial features onto a lower subspace to obtain the new features. In particular, the objective of SSGF is to maximize the class separation ability and preserve the local neighborhood structure by using both labeled and unlabeled samples. Experimental results on the hyperspectral and LiDAR data from the 2013 IEEE Geoscience and Remote Sensing Society (GRSS) Data Fusion Contest demonstrated the superiority of the SSGF.
更多查看译文
关键词
Feature extraction,Hyperspectral imaging,Laser radar,Training,Data mining,Distance measurement
AI 理解论文
溯源树
样例
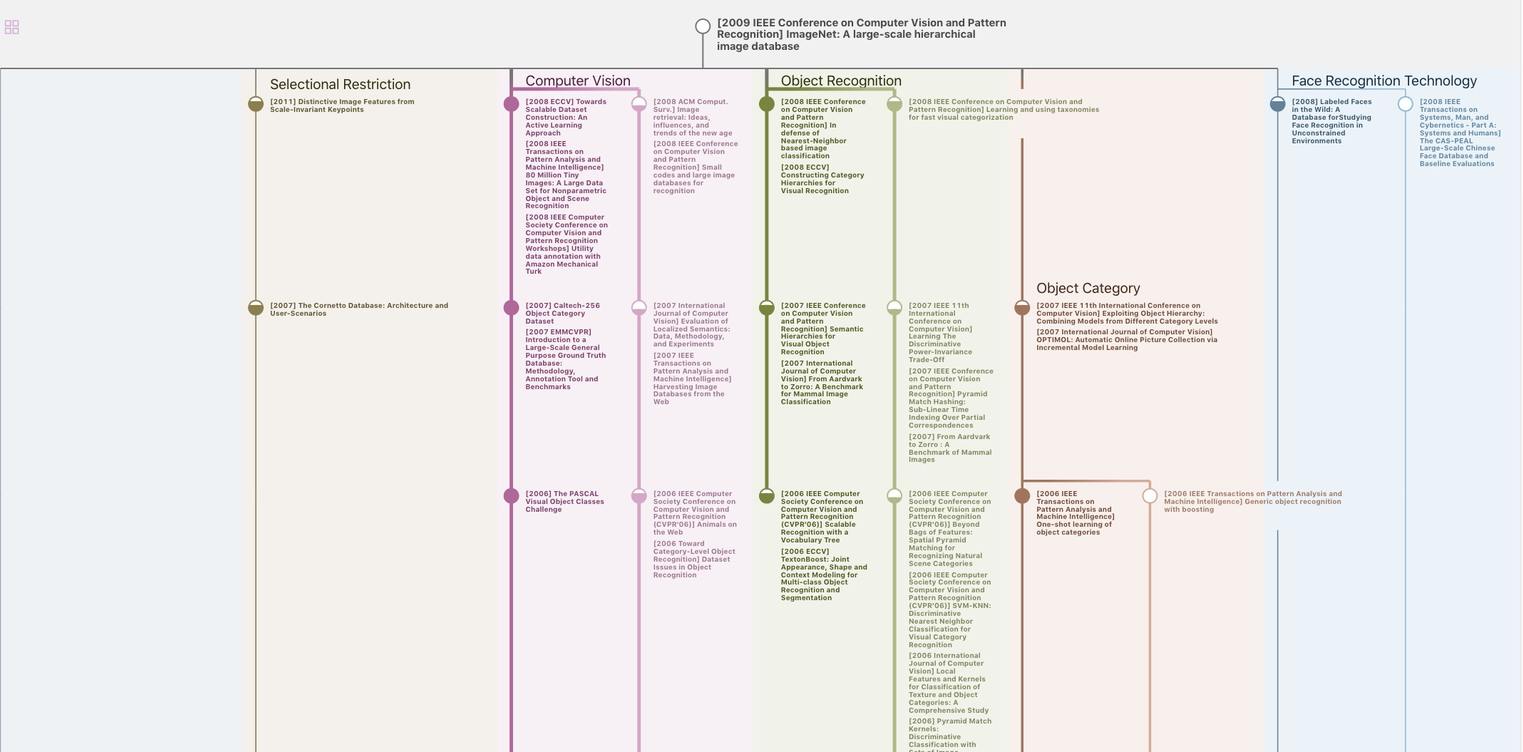
生成溯源树,研究论文发展脉络
Chat Paper
正在生成论文摘要