Mother Wavelet Selection In The Discrete Wavelet Transform For Condition Monitoring Of Wind Turbine Drivetrain Bearings
WIND ENERGY(2019)
摘要
Although the discrete wavelet transform has been used for diagnosing bearing faults for two decades, most work in this field has been done with test rig data. Since field data starts to be made more available, there is a need to shift into application studies. The choice of mother wavelet, ie, the predefined shape used to analyse the signal, has previously been investigated with simulated and test rig data without consensus of optimal choice in literature. Common between these investigations is the use of the wavelet coefficients' Shannon entropy to find which mother wavelet can yield the most useful features for condition monitoring. This study attempts to find the optimal mother wavelet selection using the discrete wavelet transform. Datasets from wind turbine gearbox accelerometers, consisting of enveloped vibration measurements monitoring both healthy and faulty bearings, have been analysed. The bearing fault frequencies' excitation level has been analysed with 130 different mother wavelets, yielding a definitive measure on their performance. Also, the applicability of Shannon entropy as a ranking method of mother wavelets has been investigated. The results show the discrete wavelet transforms ability to identify faults regardless of mother wavelet used, with the excitation level varying no more than 4%. By analysing the Shannon entropy, broad predictions to the excitation level could be drawn within the mother wavelet families but no direct correlation to the main results. Also, the high computational effort of high order Symlet wavelets, without increased performance, makes them unsuitable.
更多查看译文
关键词
bearing failure, condition monitoring, discrete wavelet transform, mother wavelet selection, wind turbine field measurements
AI 理解论文
溯源树
样例
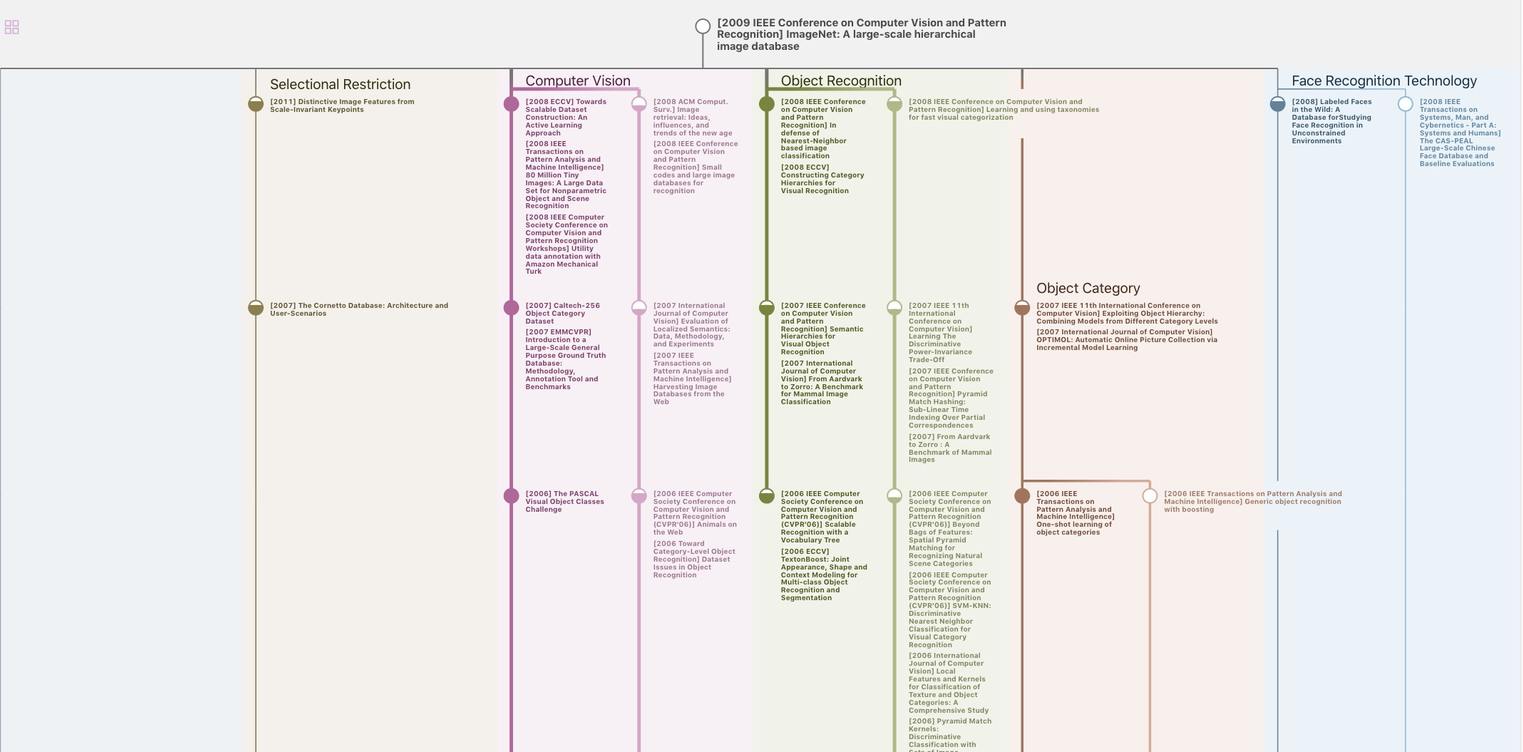
生成溯源树,研究论文发展脉络
Chat Paper
正在生成论文摘要