Predicting Students’ Academic Procrastination in Blended Learning Course Using Homework Submission Data
IEEE access(2019)
摘要
Academic procrastination has been reported affecting students' performance in computer-supported learning environments. Studies have shown that students who demonstrate higher procrastination tendencies achieve less than the students with lower procrastination tendencies. It is important for a teacher to be aware of the students' behaviors especially their procrastination trends. EDM techniques can be used to analyze data collected through computer-supported learning environments and to predict students' behaviors. In this paper, we present an algorithm called students' academic performance enhancement through homework late/non-submission detection (SAPE) for predicting students' academic performance. This algorithm is designed to predict students with learning difficulties through their homework submission behaviors. First, students are labeled as procrastinators or non-procrastinators using k-means clustering algorithm. Then, different classification methods are used to classify students using homework submission feature vectors. We use ten classification methods, i.e., ZeroR, OneR, ID3, J48, random forest, decision stump, JRip, PART, NBTree, and Prism. A detailed analysis is presented regarding performance of different classification methods for different number of classes. The analysis reveals that in general the prediction accuracy of all methods decreases with increase in the number of classes. However, different methods perform best or worst for different number of classes.
更多查看译文
关键词
Blended learning,computer-assisted learning,educational data mining as an inquiry method,e-learning,higher education,learning management systems,online learning
AI 理解论文
溯源树
样例
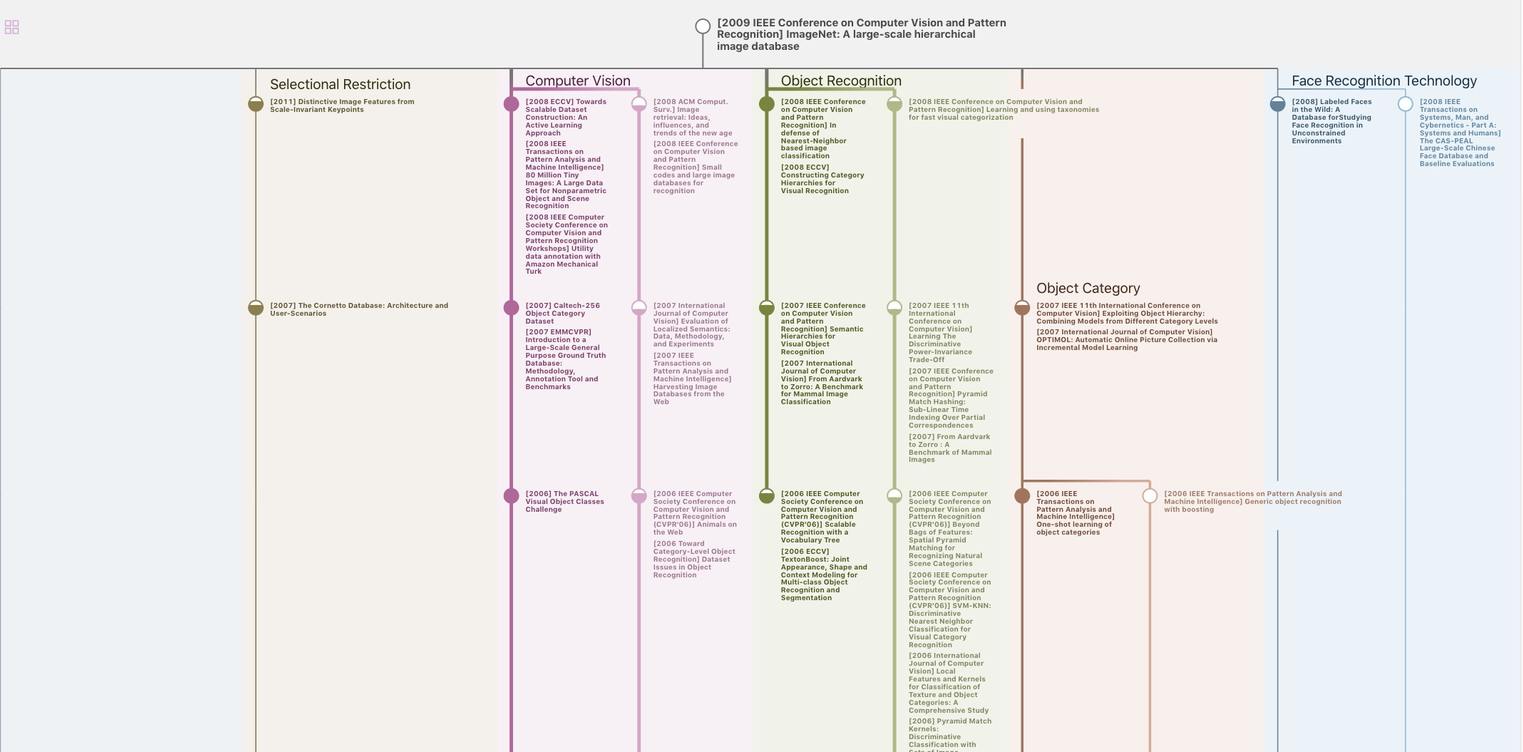
生成溯源树,研究论文发展脉络
Chat Paper
正在生成论文摘要