A Novel Classification Algorithm for MI-EEG based on Deep Learning
2019 IEEE 8th Joint International Information Technology and Artificial Intelligence Conference (ITAIC)(2019)
摘要
A key issue in brain-computer interface systems (BCI) based on motor-imagery electroencephalogram signals (MI-EEG) is the classification accuracy of EEG signals. Although deep learning (DL) methods have achieved great success in many research fields, only a limited number of works investigate its potential in BCI application research. In order to optimize the classification performance of MI-EEG signals, we propose a deep learning end-to-end classification model which is combined with convolutional neural network (CNN) and stacked autoencoders (SAE). A new type of CNN is introduced into the model for learning generalized features from time and spatial domains and for dimension reduction. Finally, the features extracted in the CNN are classified by a deep network SAE. The effectiveness of the proposed approach has been evaluated by using datasets of BCI competition data III and BCI competition data IV. Our results show that DL should be considered as an alternative to other state of art approaches, if the amount of data is large enough.
更多查看译文
关键词
motor imagery,electroencephalogram,brain-computer interface,convolutional neural network,stacked autoencoders
AI 理解论文
溯源树
样例
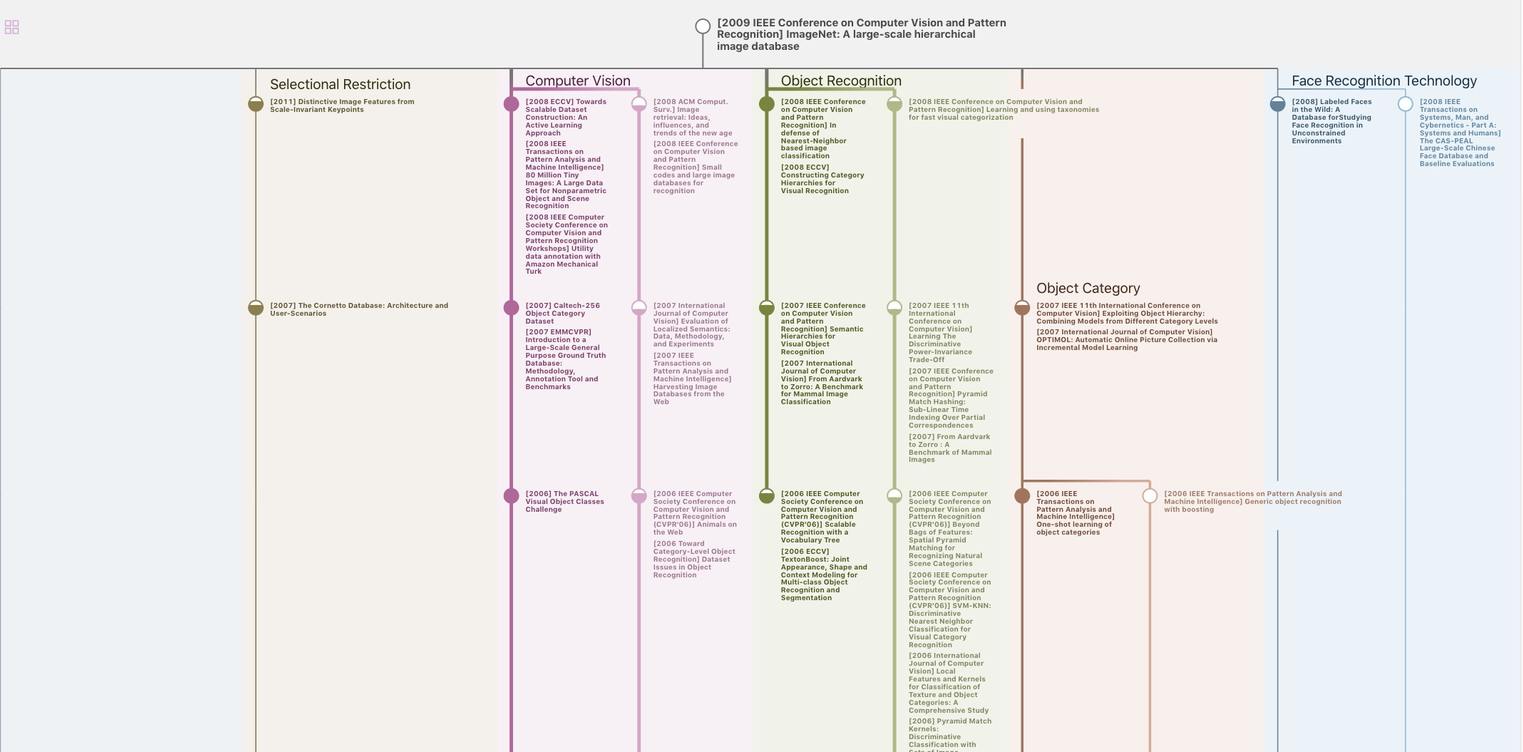
生成溯源树,研究论文发展脉络
Chat Paper
正在生成论文摘要