Discernible neighborhood counting based incremental feature selection for heterogeneous data
International Journal of Machine Learning and Cybernetics(2019)
摘要
Incremental feature selection refreshes a subset of information-rich features from added-in samples without forgetting the previously learned knowledge. However, most existing algorithms for incremental feature selection have no explicit mechanisms to handle heterogeneous data with symbolic and real-valued features. Therefore, this paper presents an incremental feature selection method for heterogeneous data with the sequential arrival of samples in group. Discernible neighborhood counting that measures different types of features, is first introduced to establish a framework for feature selection from heterogeneous data. With the arrival of new samples, the discernible neighborhood counting of a feature subset is then updated to reveal the incremental feature selection scheme. This scheme determines the criterion for efficiently adding informative features and deleting redundant features. Based on the incremental scheme, our incremental feature selection algorithm is further formulated to select valuable features from heterogeneous data. Extensive experiments are finally conducted to demonstrate the effectiveness and the efficiency of the proposed incremental feature selection algorithm.
更多查看译文
关键词
Incremental feature selection, Feature selection, Neighborhood rough set, Heterogeneous data
AI 理解论文
溯源树
样例
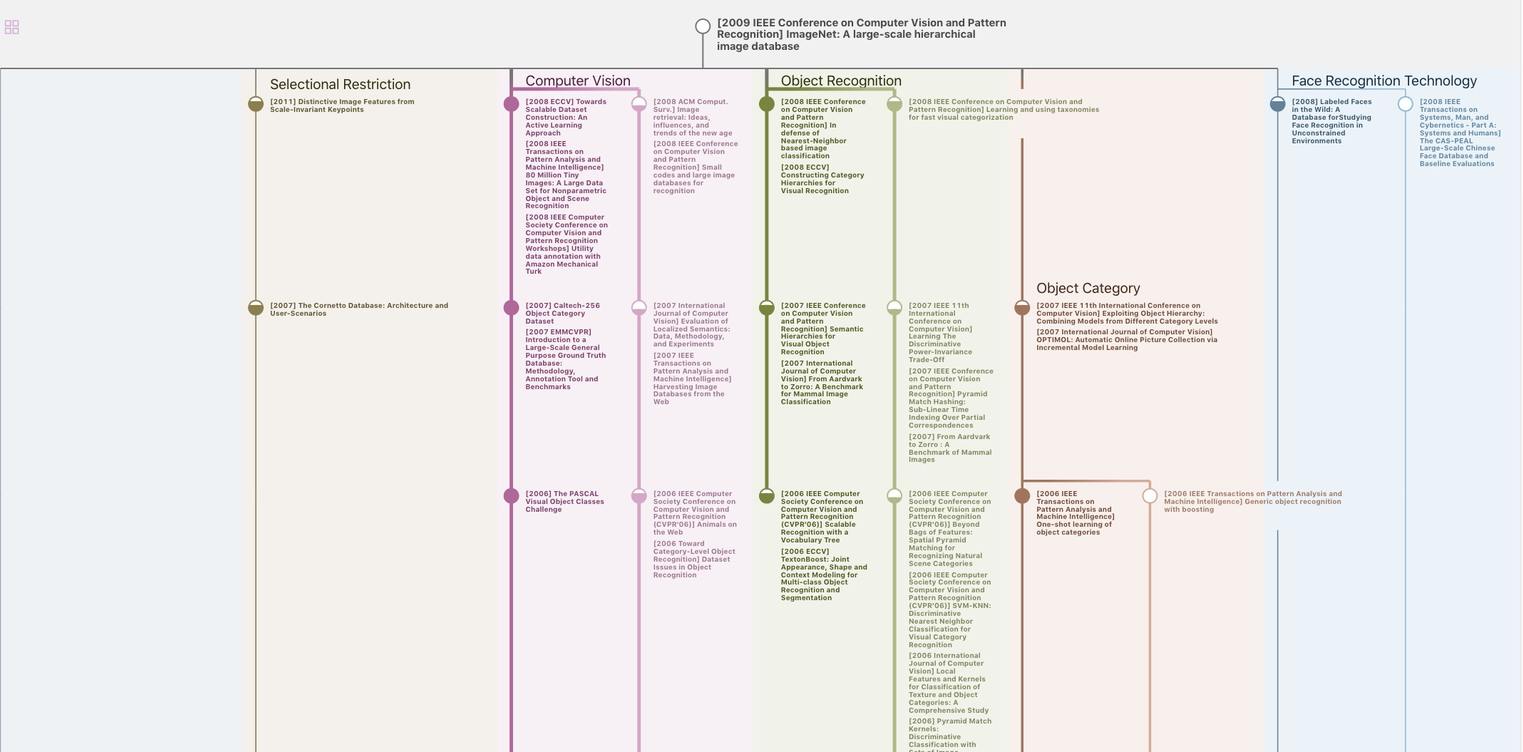
生成溯源树,研究论文发展脉络
Chat Paper
正在生成论文摘要