Identification of linear models from quantized data: a midpoint-projection approach
IEEE Transactions on Automatic Control(2020)
摘要
In this article, we consider the identification of linear models from quantized output data. We develop a variational approximation of the likelihood function, which allows us to find variationally optimal approximations of the maximum-likelihood and maximum
a posteriori
estimates. We show that these estimates are obtained by projecting the midpoint in the quantization interval of each output measurement onto the column space of the input regression matrix. Interpreting the quantized output as a random variable, we derive its moments for generic noise distributions. For the case of Gaussian noise and Gaussian independent identically distributed input, we give an analytical characterization of the bias, which we use to build a bias-compensation scheme that leads to consistent estimates.
更多查看译文
关键词
Quantization (signal),Maximum likelihood estimation,Random variables,Data models,Finite impulse response filters,Linear systems
AI 理解论文
溯源树
样例
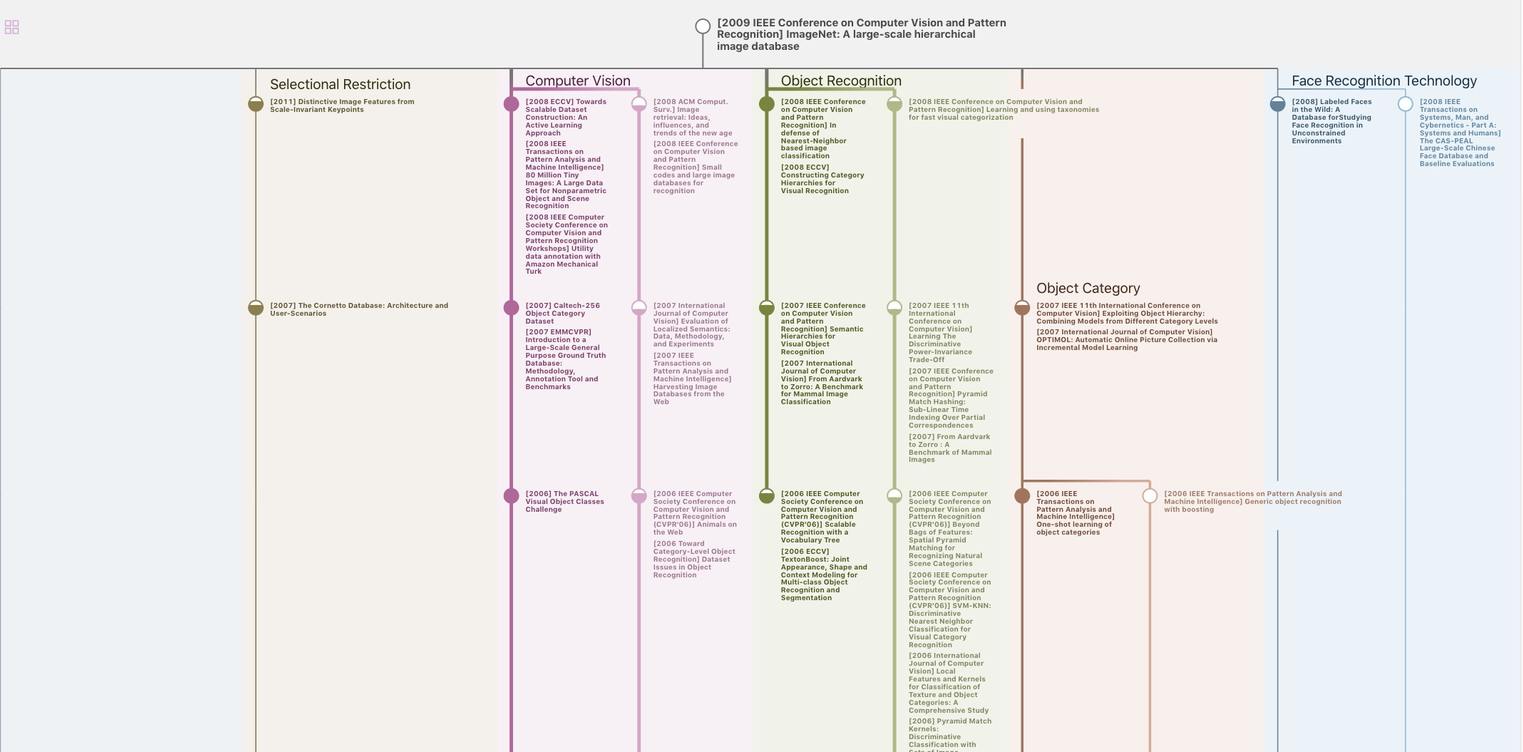
生成溯源树,研究论文发展脉络
Chat Paper
正在生成论文摘要