Rankboost + : an improvement to Rankboost
Machine Learning(2019)
摘要
Rankboost is a well-known algorithm that iteratively creates and aggregates a collection of “weak rankers” to build an effective ranking procedure. Initial work on Rankboost proposed two variants. One variant, that we call Rb-d and which is designed for the scenario where all weak rankers have the binary range {0,1} , has good theoretical properties, but does not perform well in practice. The other, that we call Rb-c , has good empirical behavior and is the recommended variation for this binary weak ranker scenario but lacks a theoretical grounding. In this paper, we rectify this situation by proposing an improved Rankboost algorithm for the binary weak ranker scenario that we call Rankboost + . We prove that this approach is theoretically sound and also show empirically that it outperforms both Rankboost variants in practice. Further, the theory behind Rankboost + helps us to explain why Rb-d may not perform well in practice, and why Rb-c is better behaved in the binary weak ranker scenario, as has been observed in prior work.
更多查看译文
关键词
Ranking, Boosting, Ensemble methods, Rankboost
AI 理解论文
溯源树
样例
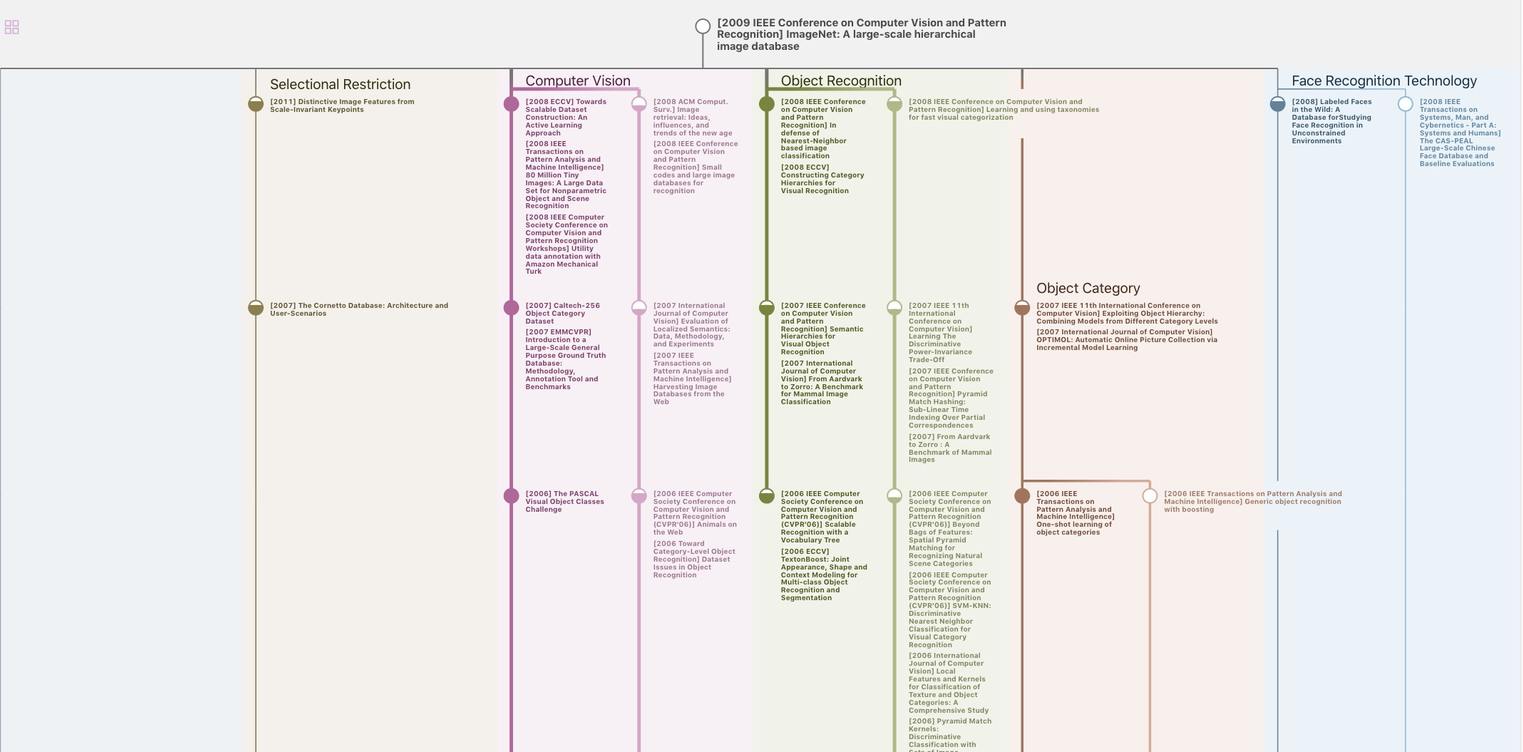
生成溯源树,研究论文发展脉络
Chat Paper
正在生成论文摘要