Machine learning-based motor assessment of Parkinson’s disease using postural sway, gait and lifestyle features on crowdsourced smartphone data
BIOMEDICAL PHYSICS & ENGINEERING EXPRESS(2020)
摘要
Objectives: Remote assessment of gait in patients' homes has become a valuable tool for monitoring the progression of Parkinson's disease (PD). However, these measurements are often not as accurate or reliable as clinical evaluations because it is challenging to objectively distinguish the unique gait characteristics of PD. We explore the inference of patients' stage of PD from their gait using machine learning analyses of data gathered from their smartphone sensors. Specifically, we investigate supervised machine learning (ML) models to classify the severity of the motor part of the UPDRS (MDS-UPDRS 2.10-2.13). Our goals are to facilitate remote monitoring of PD patients and to answer the following questions: (1) What is the patient PD stage based on their gait? (2) Which features are best for understanding and classifying PD gait severities? (3) Which ML classifier types best discriminate PD patients from healthy controls (HC)? and (4) Which ML classifier types can discriminate the severity of PD gait anomalies? Methodology: Our work uses smartphone sensor data gathered from 9520 patients in the mPower study, of whom 3101 participants uploaded gait recordings and 344 subjects and 471 controls uploaded at least 3 walking activities. We selected 152 PD patients who performed at least 3 recordings before and 3 recordings after taking medications and 304 HC who performed at least 3 walking recordings. From the accelerometer and gyroscope sensor data, we extracted statistical, time, wavelet and frequency domain features, and other lifestyle features were derived directly from participants' survey data. We conducted supervised classification experiments using 10-fold cross-validation and measured the model precision, accuracy, and area under the curve (AUC). Results: The best classification model, best feature, highest classification accuracy, and AUC were (1) random forest and entropy rate, 93% and 0.97, respectively, for walking balance (MDS-UPDRS-2.12); (2) bagged trees and MinMaxDiff, 95% and 0.92, respectively, for shaking/tremor (MDS-UPDRS-2.10); (3) bagged trees and entropy rate, 98% and 0.98, respectively, for freeze of gait; and (4) random forest and MinMaxDiff, 95% and 0.99, respectively, for distinguishing PD patients from HC. Conclusion: Machine learning classification was challenging due to the use of data that were subjectively labeled based on patients' answers to the MDS-UPDRS survey questions. However, with use of a significantly larger number of subjects than in prior work and clinically validated gait features, we were able to demonstrate that automatic patient classification based on smartphone sensor data can be used to objectively infer the severity of PD and the extent of specific gait anomalies.
更多查看译文
关键词
Parkinson's disease,gait analysis,smartphone sensing,machine learning,remote monitoring
AI 理解论文
溯源树
样例
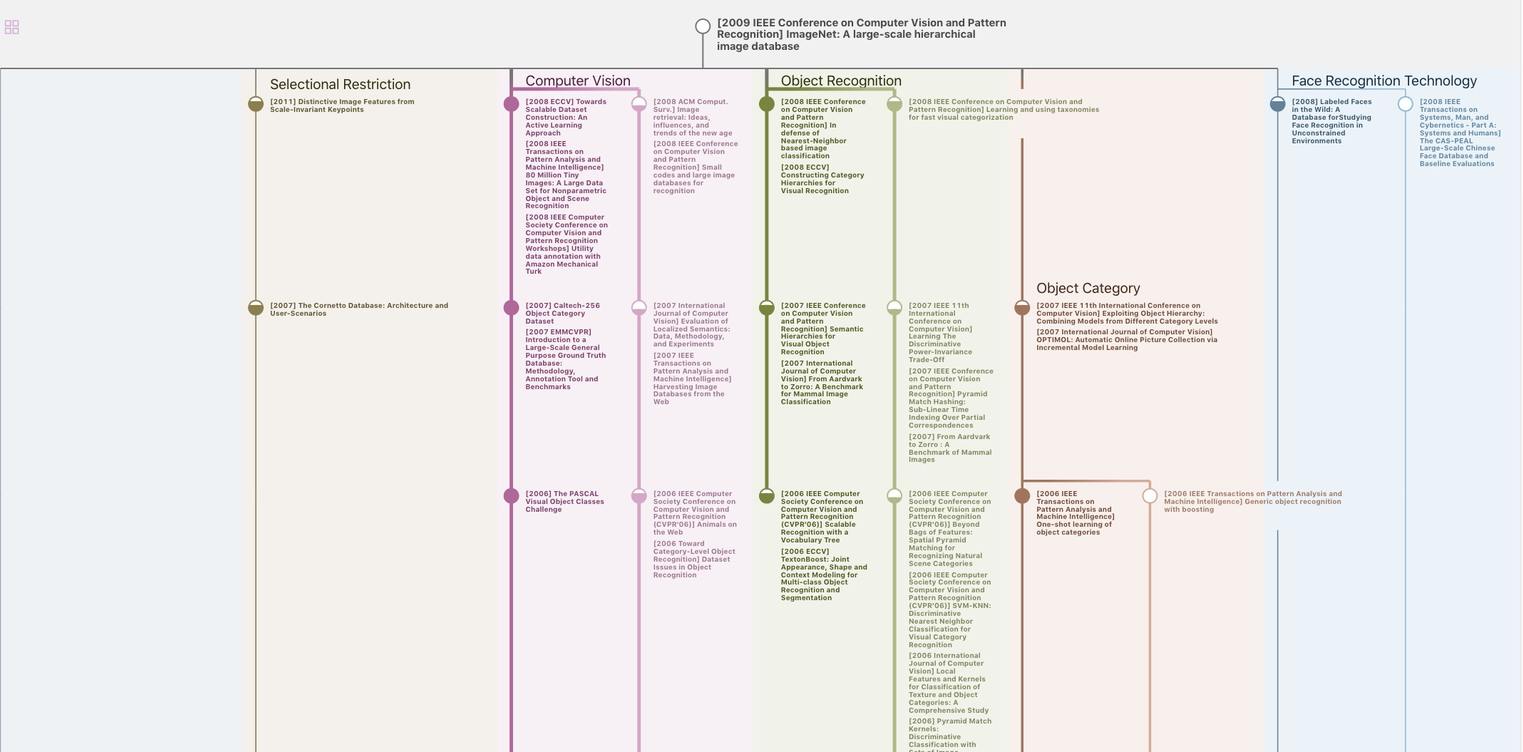
生成溯源树,研究论文发展脉络
Chat Paper
正在生成论文摘要