End-to-end deep neural network for seismic inversion
Seg Technical Program Expanded Abstracts(2019)
摘要
PreviousNext No AccessSEG Technical Program Expanded Abstracts 2019End-to-end deep neural network for seismic inversionAuthors: Ke WangLaura BanduraDimitri BevcShuxing ChengJim DiSienaAdam HalpertKonstantin OsypovBruce PowerEllen XuKe WangChevron Energy Technology CompanySearch for more papers by this author, Laura BanduraChevron Energy Technology CompanySearch for more papers by this author, Dimitri BevcChevron Energy Technology CompanySearch for more papers by this author, Shuxing ChengChevron Energy Technology CompanySearch for more papers by this author, Jim DiSienaChevron Energy Technology CompanySearch for more papers by this author, Adam HalpertChevron Energy Technology CompanySearch for more papers by this author, Konstantin OsypovChevron Energy Technology CompanySearch for more papers by this author, Bruce PowerChevron Energy Technology CompanySearch for more papers by this author, and Ellen XuChevron Energy Technology CompanySearch for more papers by this authorhttps://doi.org/10.1190/segam2019-3216464.1 SectionsAboutPDF/ePub ToolsAdd to favoritesDownload CitationsTrack CitationsPermissions ShareFacebookTwitterLinked InRedditEmail AbstractIn this paper, we introduce a deep neural network method to solve the seismic inversion problem. After standard processing steps on acquired seismic data, the resulting low frequency seismic traces can be considered as filtered version of the earth’s high frequency reflectivity by convolving reflectivity with a wavelet. This wavelet is not a physical source. Instead, it is a filter that accounts for the difference between reflectivity and seismic trace. Seismic inversion involves removing the imprints of the wavelet in the seismic data and then converting the results into acoustic or elastic impedance. For this reason, seismic inversion often starts with estimating wavelet from wells where reflectivity is known. The quality of wavelet estimation directly impacts the quality of inversion products. In the latest machine learning approaches for seismic inversion, wavelet estimation is also required to generate pairs of synthetic reflectivity and seismic trace to train a neural network. Not surprisingly, predictions made by the network are sensitive to the wavelet used to generate training data. In field applications, wavelets often vary vertically and spatially, due to attenuation, change of geology and rock properties. This non-stationarity nature imposes additional challenge in estimating wavelet from seismic data and wells. To overcome all these obstacles related to wavelet, we propose a new training strategy to train deep neural networks (DNN) to solve the seismic inversion problem. The biggest advantage of our method is that it does not need wavelet estimation. As a result, it represents a true end-to-end machine learning approach to seismic inversion.Presentation Date: Wednesday, September 18, 2019Session Start Time: 1:50 PMPresentation Start Time: 2:15 PMLocation: 301BPresentation Type: OralKeywords: inversion, machine learning, seismic impedance, artificial intelligence, waveletPermalink: https://doi.org/10.1190/segam2019-3216464.1FiguresReferencesRelatedDetailsCited byIntelligent AVA Inversion Using a Convolution Neural Network Trained with Pseudo-Well Datasets30 January 2023 | Surveys in Geophysics, Vol. 86Reflection and diffraction separation in the dip-angle common-image gathers using convolutional neural networkJiaxing Sun, Jidong Yang, Zhenchun Li, Jianping Huang, Jie Xu, and Subin Zhuang28 December 2022 | GEOPHYSICS, Vol. 88, No. 1Dual Wasserstein generative adversarial network condition: A generative adversarial network-based acoustic impedance inversion methodZixu Wang, Shoudong Wang, Chen Zhou, and Wanli Cheng22 September 2022 | GEOPHYSICS, Vol. 87, No. 6Seismic interpretation using AI (Artificial Intelligence) methods - going beyond machine learning to enable automated workflowsRio Oil and Gas Expo and Conference, Vol. 22, No. 2022Building subsurface models with horizon-guided interpolation and deep learning: Application to the Volve field dataShangsheng Yan, Xiaoming Sun, Xinming Wu, Sibo Zhang, and Hongjie Si16 May 2022 | GEOPHYSICS, Vol. 87, No. 4Seismic inversion via closed-loop fully convolutional residual network and transfer learningLingling Wang, Delin Meng, and Bangyu Wu12 August 2021 | GEOPHYSICS, Vol. 86, No. 5Deep learning for multidimensional seismic impedance inversionXinming Wu, Shangsheng Yan, Zhengfa Bi, Sibo Zhang, and Hongjie Si7 September 2021 | GEOPHYSICS, Vol. 86, No. 5Deep Neural Network-Based Permittivity Inversions for Ground Penetrating Radar DataIEEE Sensors Journal, Vol. 21, No. 6Theory-guided data science-based reservoir prediction of a North Sea oil fieldJonathan E. Downton, Olivia Collet, Daniel P. Hampson, and Tanya Colwell1 October 2020 | The Leading Edge, Vol. 39, No. 10 SEG Technical Program Expanded Abstracts 2019ISSN (print):1052-3812 ISSN (online):1949-4645Copyright: 2019 Pages: 5407 publication data© 2019 Published in electronic format with permission by the Society of Exploration GeophysicistsPublisher:Society of Exploration Geophysicists HistoryPublished Online: 01 Aug 2019 CITATION INFORMATION Ke Wang, Laura Bandura, Dimitri Bevc, Shuxing Cheng, Jim DiSiena, Adam Halpert, Konstantin Osypov, Bruce Power, and Ellen Xu, (2019), "End-to-end deep neural network for seismic inversion," SEG Technical Program Expanded Abstracts : 4982-4986. https://doi.org/10.1190/segam2019-3216464.1 Plain-Language Summary Keywordsinversionmachine learningseismic impedanceartificial intelligencewaveletPDF DownloadLoading ...
更多查看译文
关键词
deep neural network,neural network,inversion,end-to-end
AI 理解论文
溯源树
样例
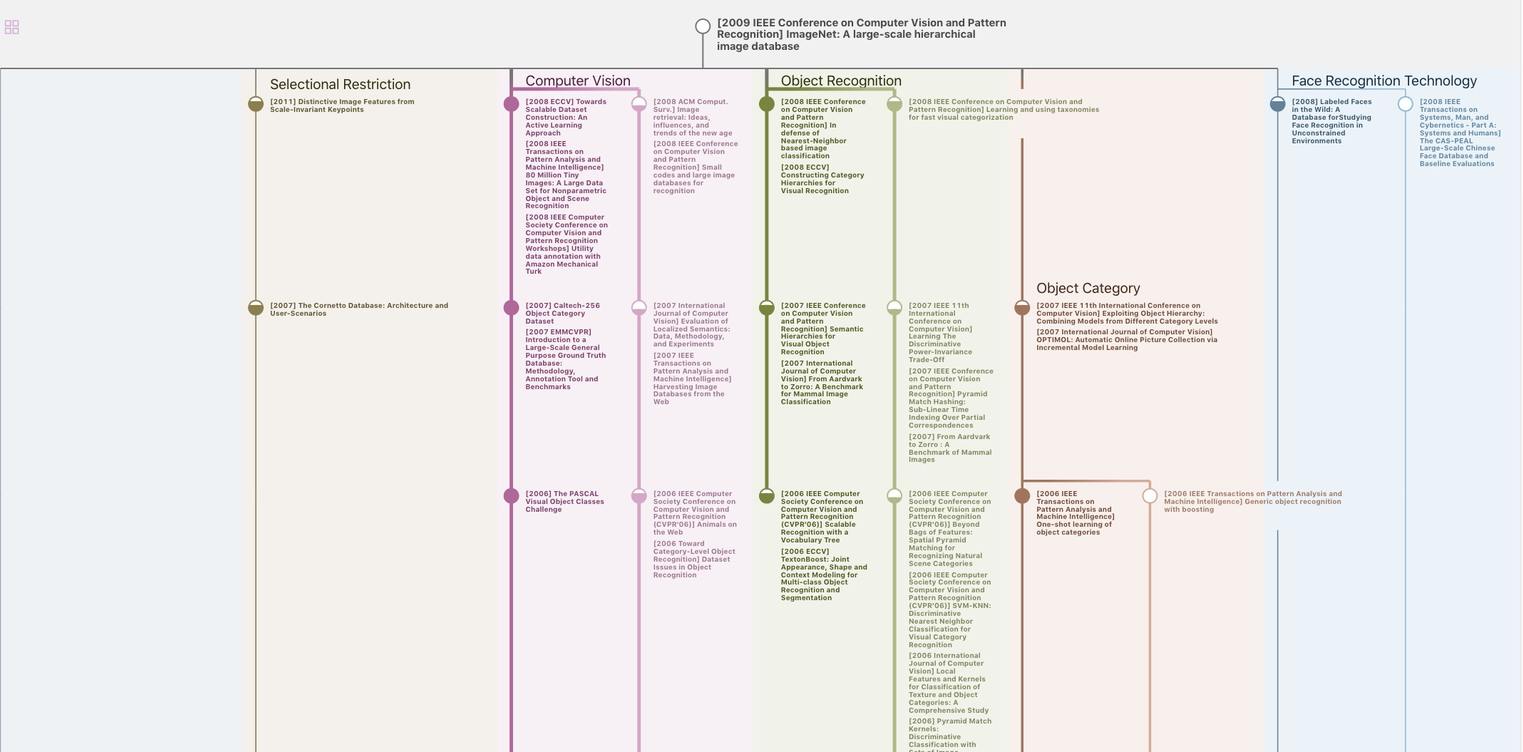
生成溯源树,研究论文发展脉络
Chat Paper
正在生成论文摘要