DeepSTD: Mining Spatio-Temporal Disturbances of Multiple Context Factors for Citywide Traffic Flow Prediction
IEEE Transactions on Intelligent Transportation Systems(2020)
摘要
Deep learning techniques have been widely applied to traffic flow prediction, considering underlying routine patterns, and multiple context factors (e.g., time and weather). However, the complex spatio-temporal dependencies between inherent traffic patterns and multiple disturbances have not been fully addressed. In this paper, we propose a two-phase end-to-end deep learning framework, namely DeepSTD to uncover the spatio-temporal disturbances (STD) to predict the citywide traffic flow. In the STD Modeling phase, we propose an STD modeling method to model both the different regional disturbances caused by various region functions and the spatio-temporal propagating effects. In the Prediction phase, we eliminate the STD from the historical traffic flow to enhance the leaning of inherent traffic patterns and combine the STD at the prediction time interval to consider the future disturbances. The experimental results on two real-world datasets demonstrate that DeepSTD outperforms the state-of-the-art methods.
更多查看译文
关键词
Traffic flow prediction,spatio-temporal disturbances,deep learning,intelligent transportation systems
AI 理解论文
溯源树
样例
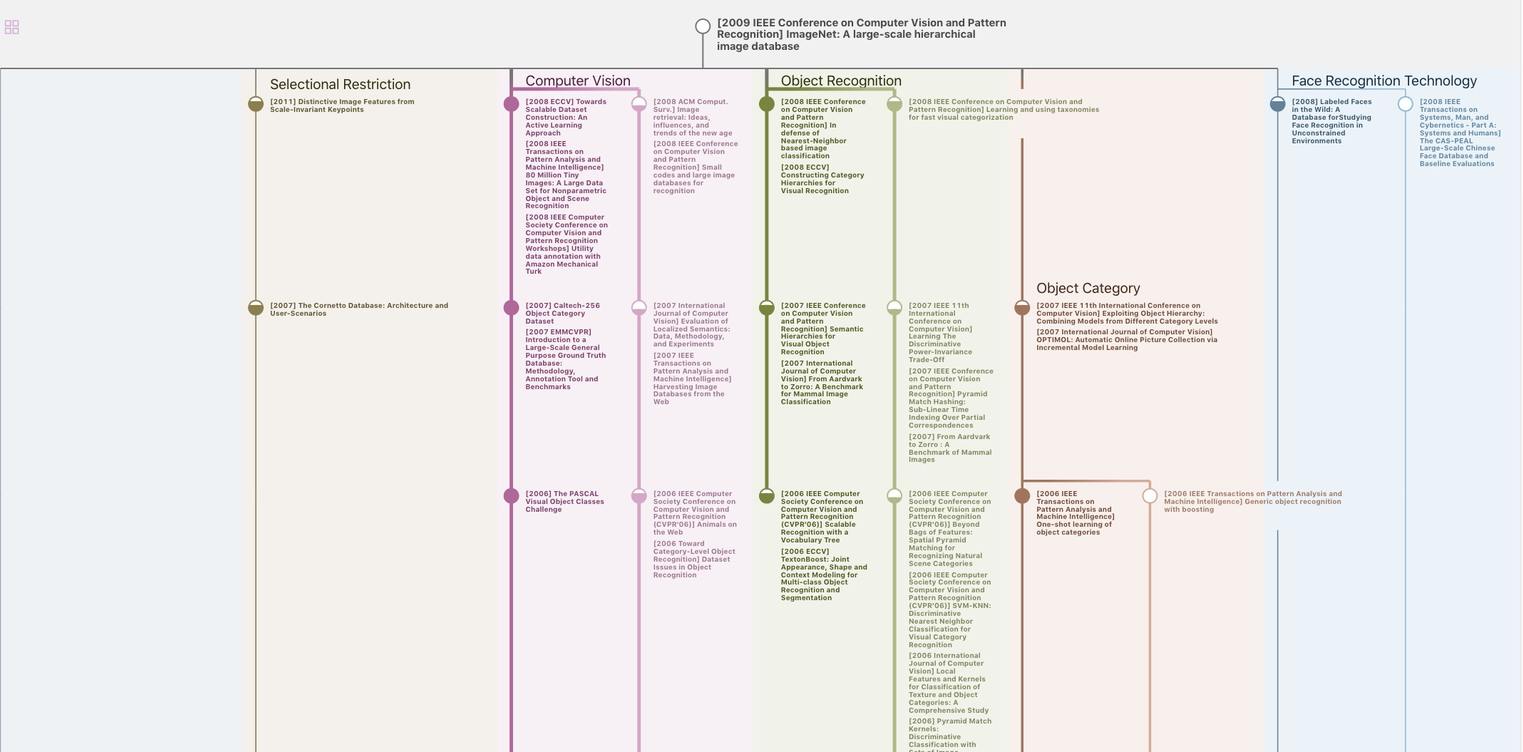
生成溯源树,研究论文发展脉络
Chat Paper
正在生成论文摘要