Quality-aware online task assignment mechanisms using latent topic model
Theoretical Computer Science(2020)
摘要
Crowdsourcing has been proven to be a useful tool for the tasks which are hard for computers. Unfortunately, workers with uneven expertise are likely to provide low-quality or even deliberately wrong data. A reliability model that precisely describes workers' performance on the tasks can benefit the development of both task assignment mechanism and truth discovery method. However, existing methods cannot model workers' fine-grained reliability levels accurately. In this paper, we consider dividing tasks into clusters (i.e., topics) based on workers' behaviors and propose a novel latent topic model to describe the topic structure and workers' topical-level expertise. Then, we develop two online task assignment mechanisms that dynamically assign each incoming worker a set of tasks where he can achieve the Maximum Expected Gain (MEG) or Maximum Expected and Potential Gain (MEPG). The experimental results demonstrate that our methods can significantly decrease the number of task assignments and achieve higher accuracy and macro-averaging F1-score than the state-of-the-art approaches.
更多查看译文
关键词
Crowdsourcing,Latent topic model,Online task assignment,Truth discovery
AI 理解论文
溯源树
样例
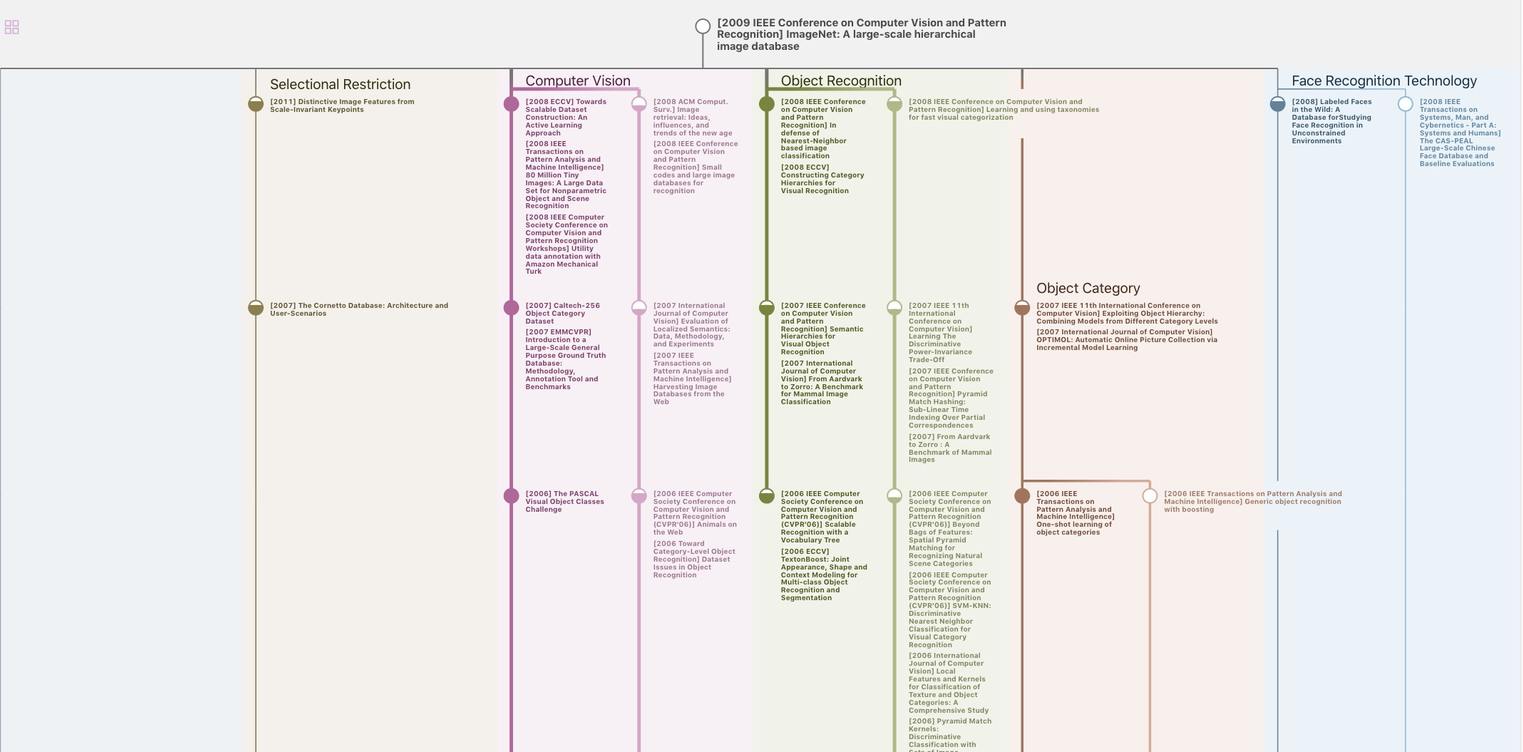
生成溯源树,研究论文发展脉络
Chat Paper
正在生成论文摘要