Graph Embedding-Based Dimension Reduction With Extreme Learning Machine
IEEE Transactions on Systems, Man, and Cybernetics: Systems(2021)
摘要
Dimension reduction (DR)-based on extreme learning machine auto-encoder (ELM-AE) has achieved many successes in recent years. By minimizing the self-reconstruction error, the ELM-AE-based DR algorithms learn the compressed representations which facilitate the subsequent classification. However, the existing ELM-AEs only consider the DR problem in an unsupervised manner and ignore the valuable supervised information when these information is available. To find discriminative features of the original data, in this paper, we propose a graph embedding-based DR framework with ELM (GDR-ELM) for DR problems. Instead of self-reconstruction, the proposed GDR-ELM reconstructs all samples according to the weights in a graph matrix containing the supervised information. Furthermore, GDR-ELM can be stacked as building blocks to construct a multilayer framework like other ELM-AEs for more complicated representation learning tasks. Experiments on various datasets demonstrate the effectiveness of the proposed GDR-ELM and its multilayer framework.
更多查看译文
关键词
Dimension reduction (DR),extreme learning machine (ELM),feature extraction,graph embedding,representation learning
AI 理解论文
溯源树
样例
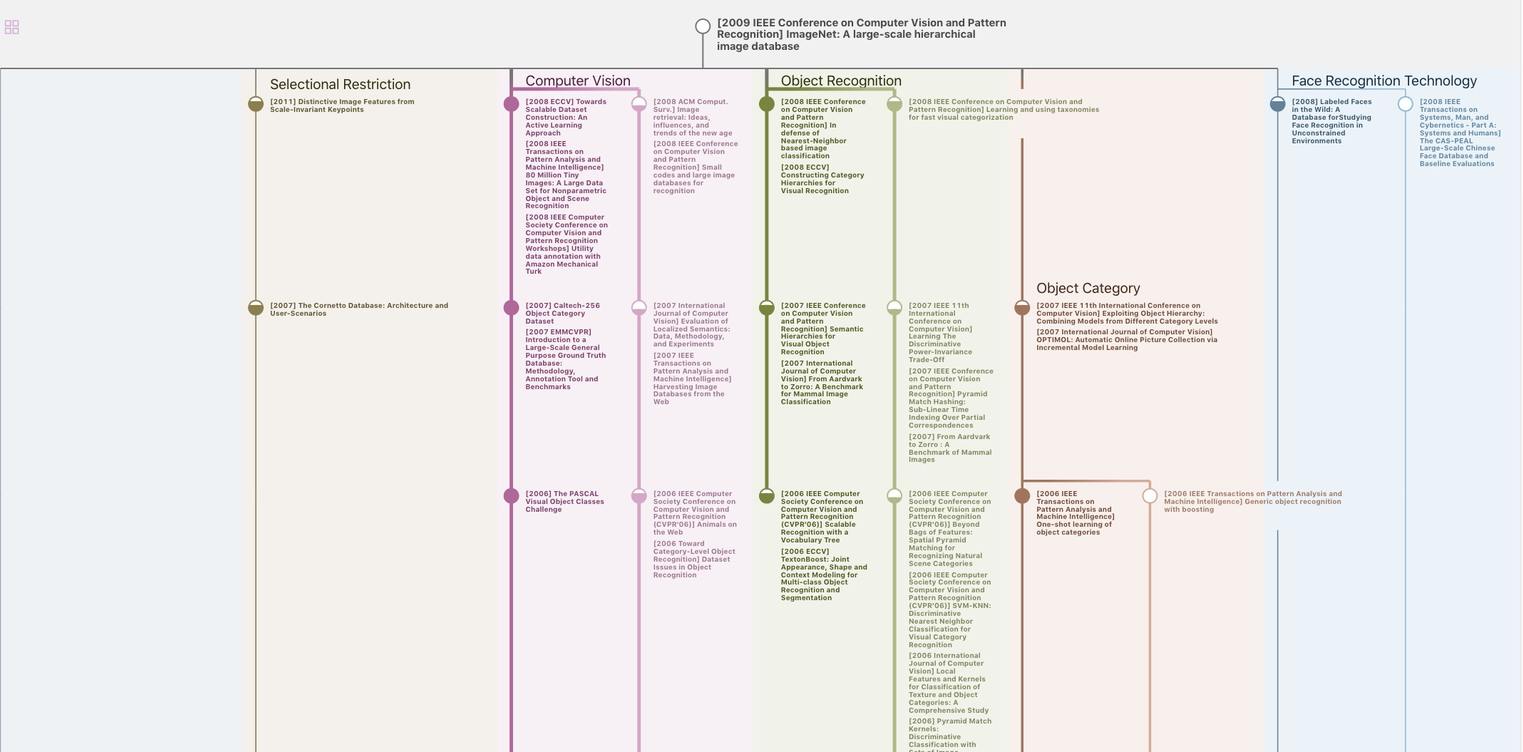
生成溯源树,研究论文发展脉络
Chat Paper
正在生成论文摘要