Multi-view Locality Preserving Embedding with View Consistent Constraint for Dimension Reduction
KSEM (1)(2019)
摘要
With the diversification of data sources, the multi-view data with multiple expressions have been appeared in various application scenarios. These multi-view data generally have high dimensions, large amounts and often lack of label information. Therefore, it is very important to learn multi-view data in an unsupervised way so as to analyze and excavate the potential valuable information. In this paper, we propose a multi-view locality preserving embedding algorithm with view similarity constraint for data dimension reduction. This algorithm not only preserves the local structure into low-dimensional space for each view, but also implements the similarity constraints between different views. On this basis, the algorithm looks for a joint embedding of low-dimensional subspace, so that the neighborhood among samples in original high-dimensional space can be maintained in the subspace, and the structures corresponding to different views are consistent with each other. This algorithm achieves good experimental results both in artificial data sets and multi-view data sets, which prove the correctness and feasibility of the algorithm.
更多查看译文
关键词
Multi-view learning, Multi-view dimensional reduction, Subspace learning
AI 理解论文
溯源树
样例
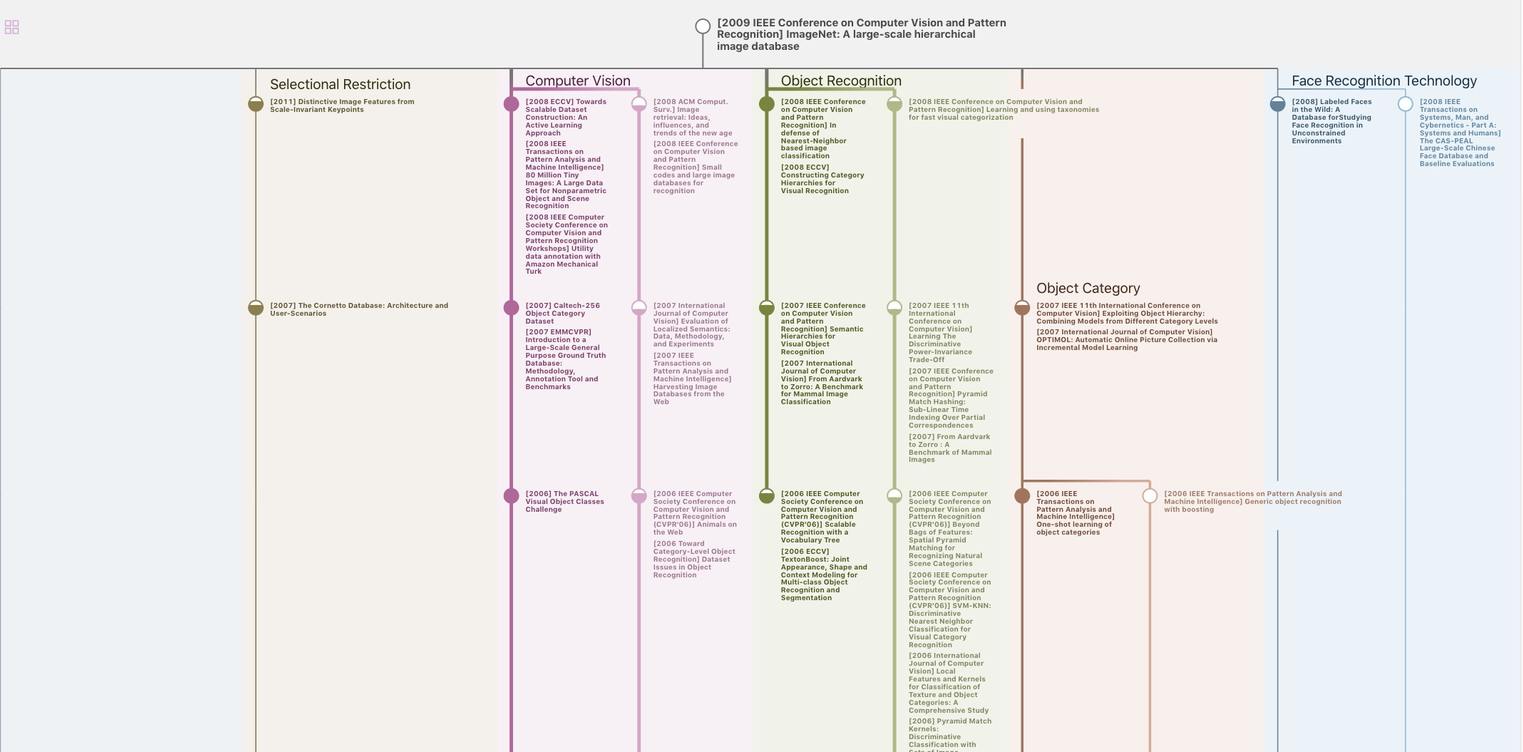
生成溯源树,研究论文发展脉络
Chat Paper
正在生成论文摘要