Spatial Predictability of Heavy Rainfall Events in East China and the Application of Spatial-Based Methods of Probabilistic Forecasting
ATMOSPHERE(2019)
摘要
One of the major issues in developing convective-scale ensemble forecasts is what is widely known as under-dispersion. This can be addressed through the consideration of spatial uncertainties via post-processing, motivating the development of various techniques to represent the spatial uncertainties of ensembles. In this study, a recently developed fraction-based approach (the ensemble agreement scale, EAS) is applied to characterize the spatial predictability and spread-skill performances of precipitation forecasts using a WRF-EnKF convective-scale ensemble forecast system over the Yangtze and Huai river valleys, China. Fourteen heavy rainfall events during the Meiyu season of 2013 and 2014 were classified into two categories-strong forcing (SF) and weak forcing (WF)-using the convective adjustment timescale. The results show that the spatial predictability and spread-skill relationship are highly regime-dependent and that both exhibit lower values under WF. Furthermore, a new object-based probabilistic approach (OBJ_NEP) is proposed as a supplement to traditional neighborhood ensemble probability (NEP) and a recently proposed fraction-based approach (EAS_NEP). The results of the application of OBJ_NEP are evaluated, and a comparison is made between NEP and EAS_NEP for the analysis of experiments involving both idealized and 'real' events by using objective verification methods. The results imply that OBJ_NEP can be effectively employed under different large-scale forcings. Consequently, these results can aid the understanding of spatial-based approaches to probabilistic forecasting, which has been widely applied to post-processing processes of convective-scale ensemble forecast systems (CSEFs) in recent years.
更多查看译文
关键词
convective-scale ensemble forecast,spatial predictability,probabilistic forecast
AI 理解论文
溯源树
样例
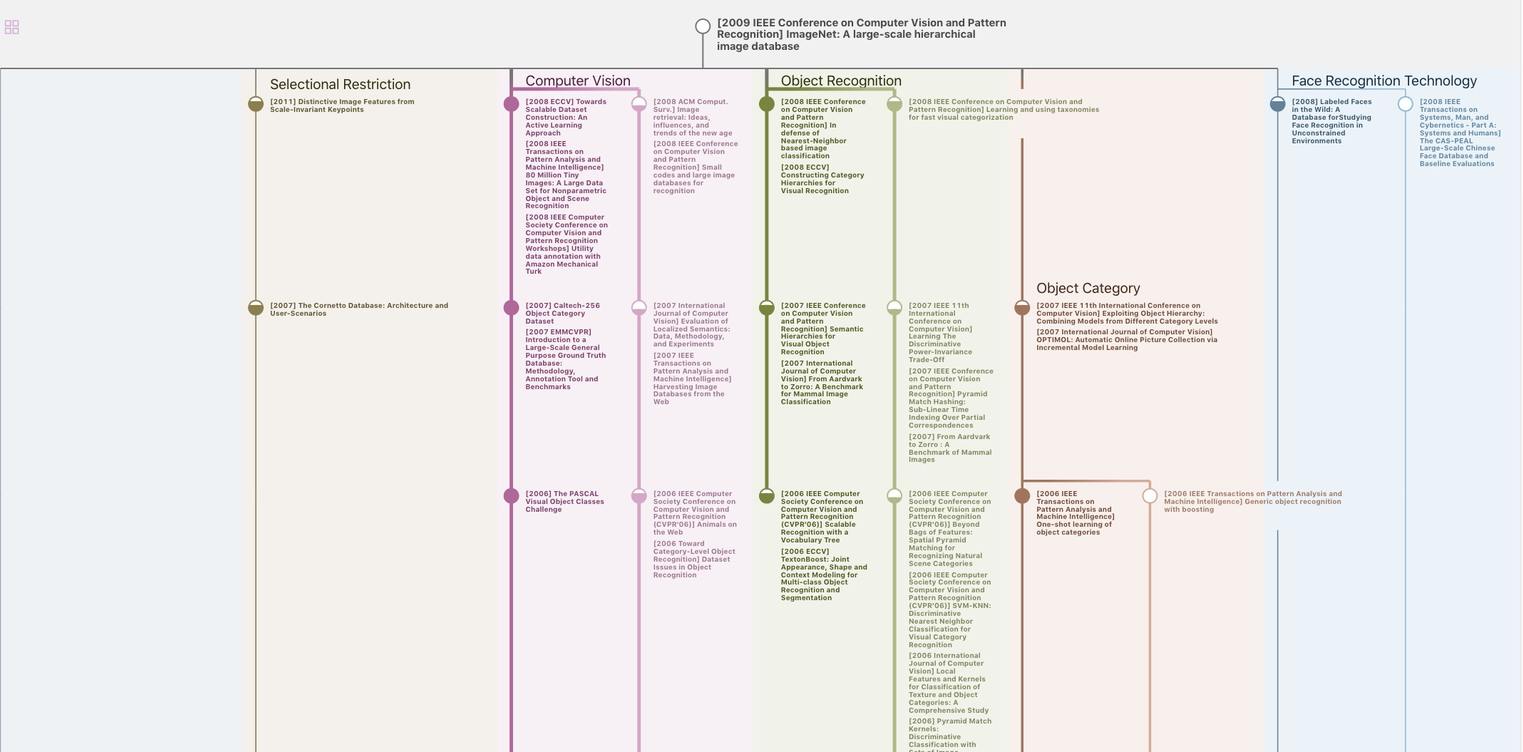
生成溯源树,研究论文发展脉络
Chat Paper
正在生成论文摘要