A Novel Method for Highly Imbalanced Classification with Weighted Support Vector Machine.
KSEM (1)(2019)
摘要
In real life, the problem of imbalanced data classification is unavoidable and difficult to solve. Traditional SVMs based classification algorithms usually cannot classify highly imbalanced data accurately, and sampling strategies are widely used to help settle the matter. In this paper, we put forward a novel undersampling method i.e., granular weighted SVMs-repetitive under-sampling (GWSVM-RU) for highly imbalanced classification, which is a weighted SVMs version of the granular SVMs-repetitive undersampling (GSVM-RU) once proposed by Yuchun Tang et al. We complete the undersampling operation by extracting the negative information granules repetitively which are obtained through the naive SVMs algorithm, and then combine the negative and positive granules again to compose the new training data sets. Thus we rebalance the original imbalanced data sets and then build new models by weighted SVMs to predict the testing data set. Besides, we explore four other rebalance heuristic mechanisms including cost-sensitive learning, undersampling, oversampling and GSVM-RU, our approach holds the higher classification performance defined by new evaluation metrics including G-Mean, F-Measure and AUC-ROC. Theories and experiments reveal that our approach outperforms other methods.
更多查看译文
关键词
Highly imbalanced classification, Undersampling, GWSVM-RU, Information granules, Weighted SVMs
AI 理解论文
溯源树
样例
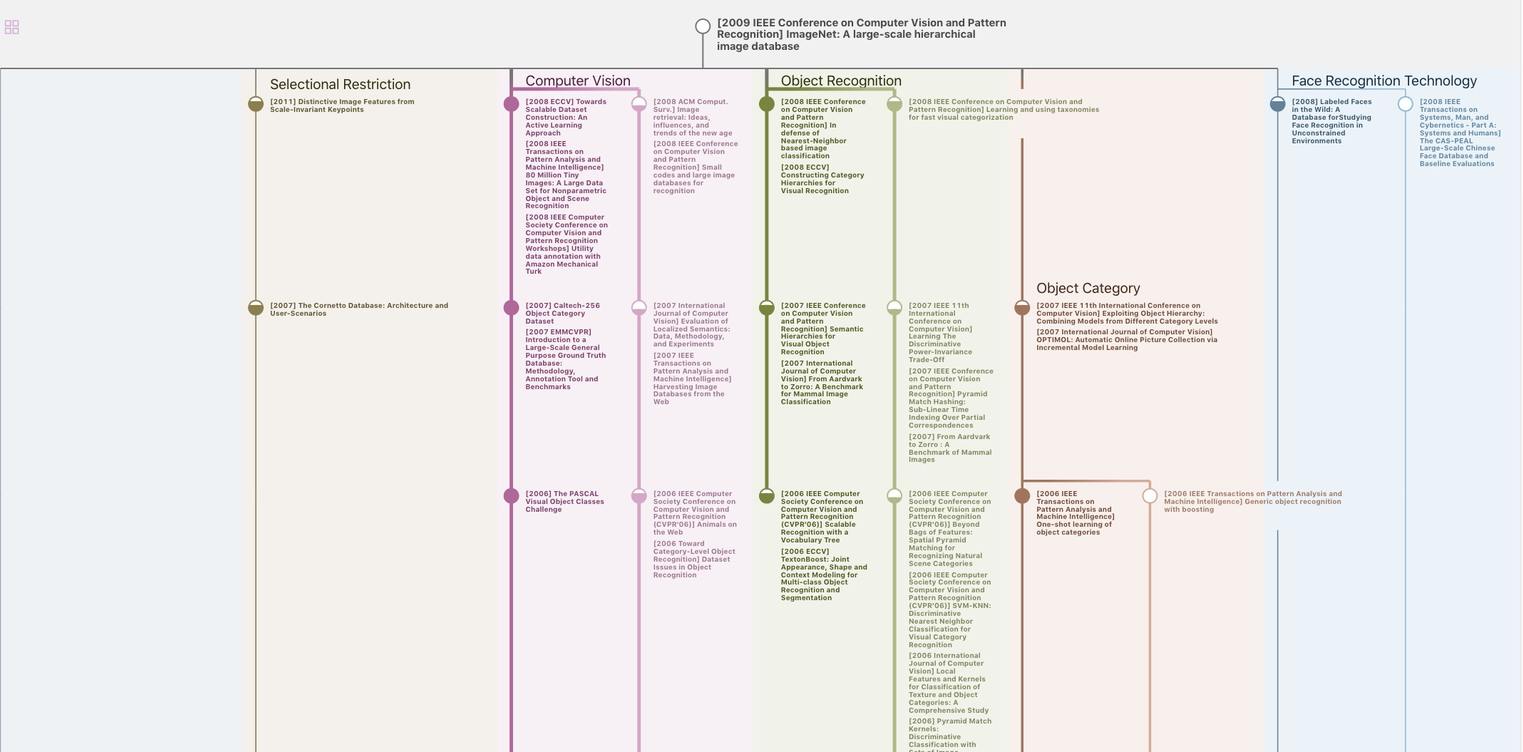
生成溯源树,研究论文发展脉络
Chat Paper
正在生成论文摘要