Patterns And Outliers In Temporal Point Processes
INTELLIGENT SYSTEMS AND APPLICATIONS, VOL 1(2020)
摘要
The behavior of users of Web sites or services is usually analyzed on the population level because data as to individual activities is typically sparse and time discrete. And while Poisson processes provide models for such data, they often lack the flexibility to cope with wide ranges of different behaviors. In this paper, we propose a clustering algorithm for temporal point process intensities that has this flexibility as we incorporate a fast spline interpolation method. We use a scalable kernel approach to handle local translation invariance and employ maximum entropy principles for model selection and outlier detection. This way, our framework allows us to uncover fine grained dynamical classes of behavior and we apply it to user answer time-stamps crawled from the question answering site StackOverflow, push events in the Github version control repository, and to transactions in the BitCoin network. Results indicate that on StackOverflow sites the user's classes are divided into bursty dynamics and into weekly dynamics with low replica activity. For the BitCoin data, we find patterns of growing activity reflecting economic bubble behavior. The Github data reveals patterns of concentrated and periodic activity.
更多查看译文
关键词
Point processes, Clustering, Data mining, User behavior
AI 理解论文
溯源树
样例
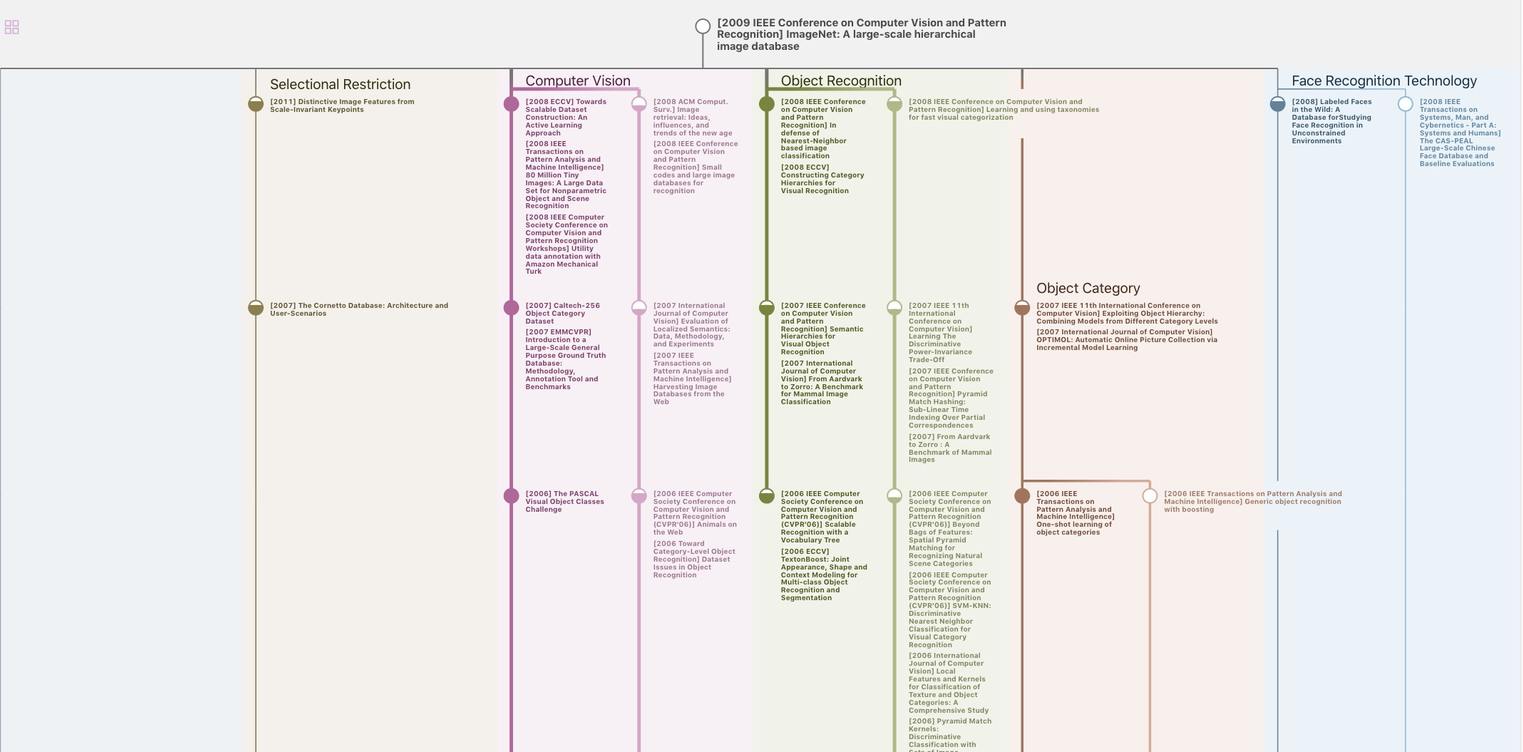
生成溯源树,研究论文发展脉络
Chat Paper
正在生成论文摘要