Mutually Regressive Point Processes
ADVANCES IN NEURAL INFORMATION PROCESSING SYSTEMS 32 (NIPS 2019)(2019)
摘要
Many real-world data represent sequences of interdependent events unfolding over time. They can be modeled naturally as realizations of a point process. Despite many potential applications, existing point process models are limited in their ability to capture complex patterns of interaction. Hawkes processes admit many efficient inference algorithms, but are limited to mutually excitatory effects. Nonlinear Hawkes processes allow for more complex influence patterns, but for their estimation it is typically necessary to resort to discrete-time approximations that may yield poor generative models. In this paper, we introduce the first general class of Bayesian point process models extended with a nonlinear component that allows both excitatory and inhibitory relationships in continuous time. We derive a fully Bayesian inference algorithm for these processes using Polya-Gamma augmentation and Poisson thinning. We evaluate the proposed model on single and multi-neuronal spike train recordings. Results demonstrate that the proposed model, unlike existing point process models, can generate biologically-plausible spike trains, while still achieving competitive predictive likelihoods.
更多查看译文
关键词
continuous time
AI 理解论文
溯源树
样例
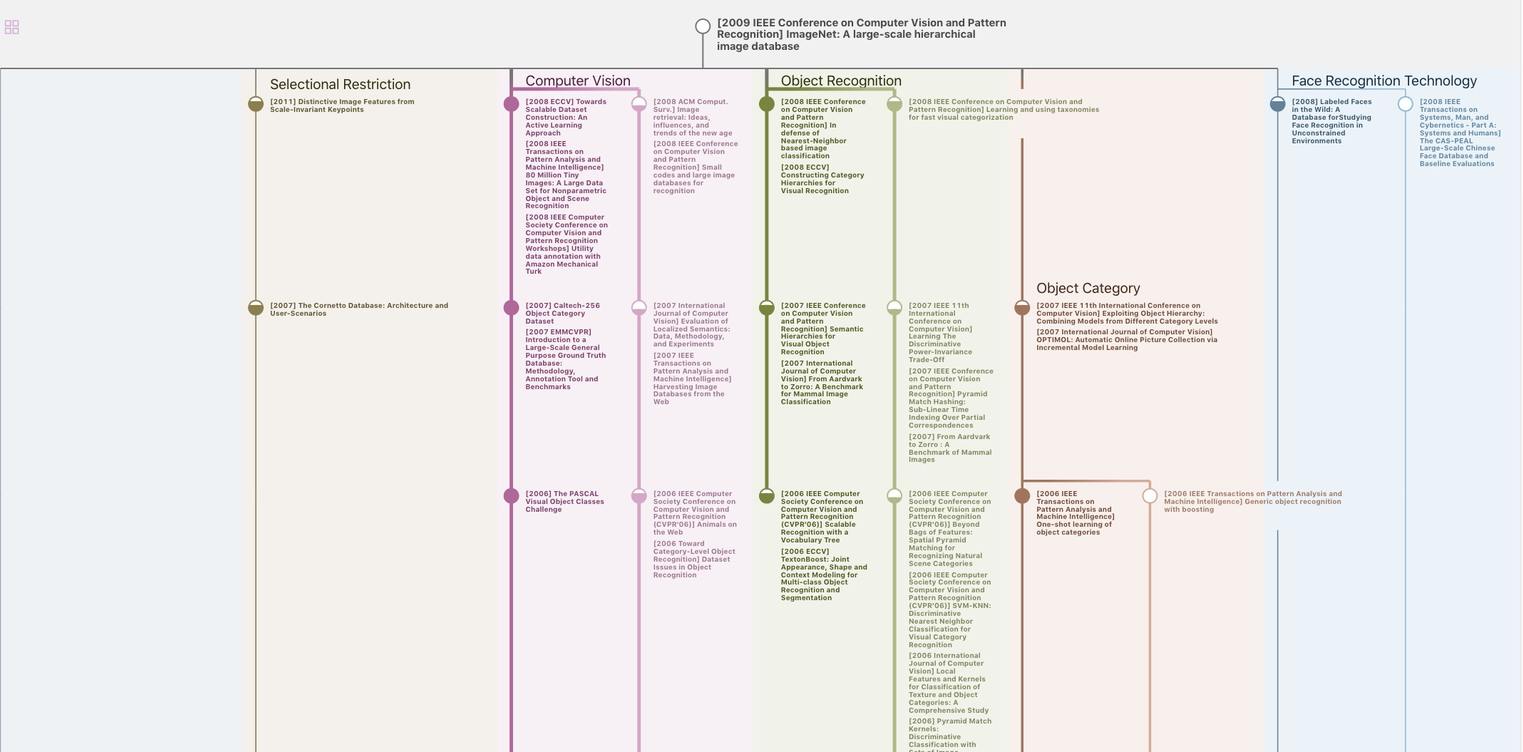
生成溯源树,研究论文发展脉络
Chat Paper
正在生成论文摘要